Exploring legal mechanisms for data stewardship
A joint publication with the AI Council, which explores three legal mechanisms that could help facilitate responsible data stewardship
4 March 2021
Reading time: 158 minutes
The three legal mechanisms discussed in the report are data trusts, data cooperatives and corporate and contractual models, which can all be powerful mechanisms in the data-governance toolbox.
The report is a joint publication with the AI Council and endorsed by the ODI, the City of London Law Society and the Data Trusts Initiative.
Exploring legal mechanisms for data stewardship
A joint publication with the AI Council, which explores three legal mechanisms that could help facilitate responsible data stewardship.
Executive summary
Organisations, governments and citizen-driven initiatives around the world aspire to use data to tackle major societal and economic problems, such as combating the
COVID-19 pandemic. Realising the potential of data for social good is not an easy task, and from the outset efforts must be made to develop methods for the responsible
management of data on behalf of individuals and groups.
Widespread misuse of personal data, exemplified by repeated high-profile data breaches and sharing scandals, has resulted in ‘tenuous’ public trust1 in public and private-sector data sharing. Concentration of power and market dominance, based on extractive data practices from a few technological players, both entrench public concern about data use and impede data sharing and access in the public interest. The lack of transparency and scrutiny around public-private partnerships add additional layers of concerns when it comes to how data is used.2 Part of these concerns comes from the fact that what individuals might consider to be ‘good’ is different to how those who process data may define it, especially if individuals have no say in that definition.
The challenges of the twenty-first century demand new data governance models for collectives, governments and organisations that allow data to be shared for individual and public benefit in a responsible way, while managing the harms that may emerge.
This work explores the legal mechanisms that could help to facilitate responsible data stewardship. It offers opportunities for shifting power imbalances through breaking data silos and allowing different levels of participatory data governance,3 and for enabling the responsible management of data in data-sharing initiatives by individuals, organisations and governments wanting to achieve societal, economic and environmental goals.
This report focuses on personal data management, as the most common type of data stewarded today in alternative data governance models.4 It points out where mechanisms are suited for non-personal data management and sees this area as requiring future exploration. The jurisdictional focus is mainly on UK law, however this report also introduces a section on EU legislative developments on data sharing and, where appropriate, indicates similarities with civil law systems (for example, fiduciary obligations resembling trust law mechanisms).
Produced by a working group of legal, technical and policy experts, this report describes three legal mechanisms which could help collectives, organisations and governments create flexible governance responses to different elements of today’s data governance challenges.
These may, for example, empower data subjects to more easily control decisions made about their data by setting clear boundaries on data use, assist in promoting desirable uses, increase confidence among organisations to share data or inject a new democratic element into data policy.
Data trusts,5 data cooperatives and corporate and contractual mechanisms can all be powerful mechanisms in the data-governance toolbox. There’s no one-size-fits-all
solution and choosing the type of governance mechanism will depend on a number of factors.
Some of the most important factors are purpose and benefits. Coming together around an agreed purpose is the critical starting point, and one which will subsequently determine the benefits and drive the nature of the relationship between the actors involved in a data-sharing initiative. These actors may include individuals, organisations and governments although
data-sharing structures do not necessarily need to include all actors mentioned.
The legal mechanisms presented in this report aim to facilitate this relationship, however the broader range of collective action and coordination mechanisms to address data challenges also need to be assessed on a case-by-case basis. The three mechanisms described here are meant to provide an indication as to the types of approaches, conditions and legal tools that can be employed to solve questions around responsible data sharing and governance.
To demonstrate briefly how purpose can be linked to the choice of legal tools:
Data trusts create a vehicle for individuals to state their aspirations for data use and mandate a trustee to pursue these aspirations.6 Data trusts can be built with a highly participatory structure in mind, requiring systematic input from the individuals that set up the data trust. It’s also possible to build data trusts with the intention to delegate to the data trustee the responsibility to determine what type of data processing is to the beneficiaries’ interest.
The distinctive elements of this model are the role of the trustee, who bears a fiduciary duty in exercising data rights (or the beneficial interest in those rights) on behalf of the beneficiaries, and the role of the overseeing court in providing additional safeguards. Therefore, data trusts might work better in contexts where individuals and groups wish to define the terms of data use by creating a new institution (a trust) to steward data on their behalf, by representing them in negotiations about data use.
Data cooperatives can be considered when individuals want to voluntarily pool data resources and repurpose the data in the interests of those it represents. Therefore, data cooperatives could be the go-to governance mechanism when relationships are formed between peers or like-minded people who join forces to collectively steward their data and create one voice
in relation to a company or institution.
Corporate and contractual mechanisms can be used to design an ecosystem of trust in situations where a group of organisations see benefits in sharing data under mutually agreed terms and in a controlled way. This means these mechanisms might be better suited for creating data-sharing relationships between organisations. The involvement of an independent data steward is envisaged as a means of creating a trusted environment for stakeholders to feel comfortable sharing data with other parties, who they may not know or have had an opportunity to develop a relationship of trust.
This report captures the leading thinking on an emerging and timely issue of research and inquiry: how we can give tangible effect to the ideal of data stewardship: the trustworthy and responsible use and management of data.
Promoting and realising the responsible use of data is the primary objective of the Legal Mechanisms for Data Stewardship working group and the Ada Lovelace Institute, who produced this report, and who view this approach as critical to protecting the data rights of individuals and communities, and unlocking the benefits of data in a way that’s fair, equitable and focused on social benefit.
Chapter 1: Data trusts
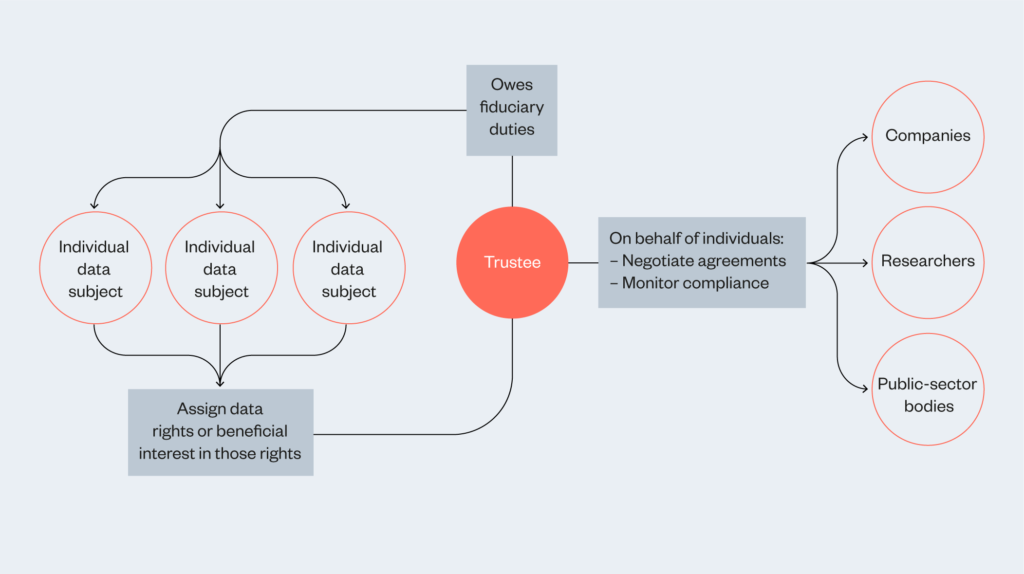
Equity as a tool for establishing rights and remedies
Trust law has ancient roots, with the fiduciary responsibilities that sit at its core being traceable to practices established in Roman law. In the UK, the idea of a ‘trust’ as an entity has its origins in medieval England: with many landowners leaving England to fight in the Crusades, systems were needed to manage their estates in their absence.
Arrangements emerged through which Crusaders would transfer ownership of their estate to another individual, who would be responsible for managing their land and fulfilling any feudal responsibilities until their return. However, returning Crusaders often found themselves in disputes with their ‘caretaker’ landowners about land ownership. These disputes were referred to the Courts of Chancery to decide on an appropriate – equitable – remedy. These courts consistently recognised the claims of the returning Crusaders, creating the concepts of a ‘beneficiary’, ‘trustee’ and ‘trust’ to define a relationship in which one party would manage certain assets for the benefit of another – the establishment of a trust.
While the practices associated with trust law have changed over time, their core components have remained consistent: a trust is a legal relationship between at least two parties, in which one party (the trustee) manages the rights associated with an asset for the benefit of another (the beneficiary).7 Almost any right can be held in trust, so long as the trust meets three conditions:
- there is a clear intention to establish a trust
- the subject matter or property of the trust is defined
- the beneficiaries of the trust are specified (including as a conceptual
category rather than nominally).
In the centuries that followed their emergence, the Courts of Chancery have played an important role in settling claims over rights and creating remedies where these rights have been infringed. Core to the operation of these courts is the concept of equity – that disputes should be settled in a way that is fair and just. In centring this concept in their jurisprudence, they have found or clarified new rights or responsibilities that might not be directly codified in Common Law, but which can be adjudicated according to legal principles of fairness. This has enabled the courts to develop flexible and innovative responses in situations where there may be gaps in Common Law, or where the strict definitions of the Common Law are ill-equipped to manage new social practices.
It is this ability to flex and adapt over time that has ensured the longevity of trusts and trust law as a governance tool, and it is these characteristics that have attracted interest in current debates about data governance.
Why data trusts?
Today’s data environment is characterised by structural power imbalances. Those with access to large pools of data – often data about individuals – can leverage the value of aggregated data to create products and services that are foundational to many daily activities.
While offering many benefits, these patterns of data use can create new forms of vulnerability for individuals or groups. Recent years have brought examples of how new uses of data can, for example, create sensitive data about individuals by combining datasets that individually seemed innocuous, or use data to target individuals online in ways that might lead to discrimination or social division.
Today, these rights are typically managed through service agreements or other consent-based models of interaction between individuals and organisations. However, as patterns of data collection and use evolve, the weaknesses associated with these processes are becoming clearer. This has prompted re-examination of consent as a foundation for data exchange and the long-term risks associated with complex patterns of data use.
The limitations of consent as a model for data governance have already been well-characterised. Many terms and conditions are lengthy and difficult to understand, and individuals might not have the ability, knowledge or time to adequately review data access agreements; for many, interest in consent and control is sparked only after they have become aware of data misuse; and the processes for an individual to enact their data rights – or receive redress for data misuse – can be lengthy and inaccessible.8
Moreover, as interactions in the workplace, at home or with public services are increasingly shaped by digital technologies, there is pressure on individuals to ‘opt in’ to data exchanges, if they are to be able to participate in society. This reliance on digital interactions exacerbates power imbalances in the governance system.
Approaches to data governance that concentrate on single instances of data exchange also struggle to account for the pervasiveness of data use, much of this data being created as a result of a digital environment in which individuals ‘leak’ data during their daily activities. In many cases, vulnerabilities arising from data use come not from a single act of data processing, but from an accumulation of data uses that may have been innocuous individually, but that together form systems that shape the choices individuals make in their daily lives – from the news they read to the jobs adverts they see. Even if each single data exchange is underpinned by a consent-based interaction, this cumulative effect – and the long-term risks it can create – is something that existing policy frameworks are not well-placed to manage.9
Nevertheless, it needs to be pointed out that the foundational elements of the GDPR that govern data processing are principles such as data protection by design and by default, and mechanisms such as data protection impact assessments (DPIAs), which are designed to help preempt potential risks as early as possible. These are legal obligations and a prerequisite step before individuals are asked for consent.10 Therefore, it is important to highlight the broader compliance failures as well as the limitations of the consent mechanism which play a significant role in creating imbalances of power and potential harm.
The imbalances of power or ability of individuals and groups to act in ways that define their own future create a data environment that is in some ways akin to the feudal system which fostered the development of trust law. Powerful actors are able to make decisions that affect individuals, and – even if those actors are expected to act with a duty of care for individual rights and interests – individuals have limited ability to challenge these structures.
There are also limited mechanisms allowing individuals who want to share data for public benefit to do so via a structure that warrants trust. In areas where significant public benefit is at stake, individuals and communities may wish to take a view on how data is used, or press for action to use data to tackle major societal challenges. At present, the vehicles for the public to have such a voice are limited.
For the purposes of this report, trust law is explored as a new form of governance that can achieve goals such as:
- increase an individual’s ability to exercise the rights they currently have in law
- redistribute power in the digital environment in ways that support individuals and groups to proactively define terms of data use
- support data use in ways that reflect shifting understandings of social value and changing technological capabilities.
The opportunities for commercial or not-for-profit organisations focused on product or research development, or which are seriously concerned about implementing a high degree of ethical obligations when it comes to data pertaining to their customers (and empower these customers not only to make active choices about data management, but also benefit from insights from this data) are briefly discussed in the section on ‘Opportunities for organisations to engage with data trusts’.
What is a data trust?
A data trust is a proposed mechanism for individuals to take the data rights that are set out in law (or the beneficial interest in those rights) and pool these into an organisation – a trust – in which trustees would exercise the data rights conferred by the law on behalf of the trust’s
beneficiaries.
Public debates about data use often centre around key questions such as who has access to data about us and how is it used. Data trusts would provide a vehicle for individuals and groups to more effectively influence the answers to these questions, by creating a vehicle for individuals to state their aspirations for data use and mandate a trustee to pursue these aspirations. By connecting the aspiration to share data to structures that protect individual rights, data trusts could provide alternative forms of ‘weak’ democracy, or new mechanisms for holding those in power to account.
The purposes for which data should be used, or data rights exercised, would be specified in the trust’s founding documents, and these purposes would be the foundation for any decision about how the trust would manage its assets. Mechanisms for deliberation or consultation with beneficiaries could also be built into a trust’s founding charter, with the form and function of those mechanisms depending on the objectives and intentions of the parties creating the trust.
Trustees and their fiduciary duties
Trustees play a crucial role in the success of such a trust. Data trustees will be tasked with stewarding the assets managed in a trust on behalf of its beneficiaries. In a ‘bottom-up’ data trust,11 the beneficiaries will be the data subjects (whose interests may include research facilitation, etc.). Data trustees will have a fiduciary responsibility to exercise (or leverage the beneficial interest inherent in) their data rights. Data trustees may seek to further the interests of the data subjects by entering into data-sharing agreements on their behalf, monitoring compliance with those agreements or negotiating better terms with service providers.
By leveraging the negotiating power inherent in pooled data rights, the data trustee would become a more powerful voice in contract negotiations, and be better placed to achieve favourable terms of data use than any single individual. In so doing, the role of the data trustee would be to empower the beneficiaries, widening their choices about data use beyond the ‘accept or walk away’ dichotomy presented by current governance structures. This role would require a high level of skill and knowledge, and support for a cohort of data trustees would
be needed to ensure they can fulfil their responsibilities.
Core to the rationale for using trust law as a vehicle for data governance is the fiduciary duty it creates. Trustees are required to act with undivided loyalty and dedication to the interests and aspirations of the beneficiaries.12 The strong safeguards this provides can create a foundation for data governance that gives data subjects confidence that their data rights are being managed with care.
Adding to these fiduciary duties, the law of equity provides a framework for accountability. If not adhering to the constitutional terms of a trust, trustees can be held to account for their actions by the trust’s beneficiaries (or the overseeing Court acting on their behalf) or an
independent regulator. Not only is a Court’s equitable jurisdiction to supervise, and intervene if necessary, not easily replicable within a contractual or corporate framework, the importance of the fact that equity relies on ex-post moral standards and emphasises good faith cannot be overestimated.
The flexibility offered by trusts also offers benefits in creating a governance system that is able to adapt to shifting patterns of data use. A range of subject matters or application areas could form the basis of a trust, allowing trusts to be established according to need: trusts would therefore allow co-evolution of patterns of data use and regulation.
In conditions of change or uncertainty around data use, this flexibility offers the ability to act now to promote some types of data use, while creating space to change practices in the future.
A further advantage of trust law is its ability to enable collective action while providing institutional safeguards that are commensurate to the vulnerabilities at stake. It is possible to imagine situations in which individuals might group together on the basis of shared values or
attitudes to risk, and seek to use this shared understanding to promote data use. In coming together to define the terms of a trust, individuals would be able to express their agency and influence data use by defining their vision. The beneficiaries’ interest can be expressed in more restrictive or prudential terms, or may include a broader purpose such as the furthering of research or influencing patterns of data use. Current legal frameworks offer few opportunities to enable group action in this way.
The relationship between data rights and trusts
Almost any right or asset can be placed in trust. Trusts have already been established for rights relating to intellectual property and contracts, alongside a range of different types of property, including digital assets, and have proven themselves to be flexible in adapting to different types of asset across the centuries.13
Understanding what data rights can be placed in trust, when those rights arise and how a trust can manage those rights will be crucial in creating a data trust. Further work will be required to analyse the sorts of powers that a trustee tasked with stewarding those rights might be able to wield, and the advantages that might accrue to the trust’s beneficiaries as a result.
In the case of data about individuals, the GDPR confers individual rights in respect of data use, which could in principle be held in trust. These include ‘positive’ rights such as portability, access and erasure that would appear to be well-suited to being managed via a trust.
The development of data trusts will require further clarity on how these rights can be exercised. There is already active work on the extent to which (and conditions according to which) those positive rights may be mandatable to another party to act on behalf of an individual, such as a trustee. Opinions on the issue differ among GDPR experts and publication of the European Commission’s draft Data Governance Act raises new questions about how and whether data rights might be delegated to a trust. The feasibility of data trusts however does not hinge on a positive answer to this delegability question, since trust law offers a potential workaround that does not require any right transfer.14
As trusts develop, they will also encounter new questions about the limitations of existing rights and what happens when different rights interact.15 For example, organisations can analyse aggregated datasets and create profiles of individuals, generating inferences about their likely preferences or behaviours. These profiles – created as a result of data analysis and modelling – would typically be considered the intellectual property of the entity that conducted the analysis or modelling. While input data might relate to individuals, once aggregated and anonymised to a certain extent, it would no longer be considered as personal data under the GDPR. However, if inferences are classified as personal data within the scope of the GDPR, individual data-protection rights should apply. Nevertheless, as some authors have explained, exercising data rights on inferences classified as personal data remains limited, and particularly in the case of data portability could give rise to different tensions with trade secrets and intellectual property.16
An example helps illustrate the challenges at stake: in the context of education technologies, data provided by a student – from homework to online test responses – would be portable under the rights set out in the GDPR, but model-generated inferences about what learning methods would be most effective for that student could be considered as the intellectual property of the training provider. The establishment of a trust to govern the use of pupil data (just like any other ‘bottom-up’ data trust) could help shed light on those necessarily contested borders between intellectual property (IP) rights – that arise from creative input in developing the models that produce individual profiles – and personal data rights.
There will never be a one-size-fits-all answer on where to draw these boundaries between IP and personal data.17 Instead, what is needed is a mechanism for negotiating these borders between parties involved in data use. In such cases, data trustees could have a crucial public advocacy function in negotiations about the extent to which such inferences fall within the scope of portability provisions.
Examining the data rights that might be placed in trust points to important differences between the use of trusts as a data governance tool and their traditional application.
Typically, assets placed in trust have value at the time the trust is created. In contrast, modern data practices mean that data acquires value in aggregate – it is the bringing together of data rights in a trust that gives trustees power to influence negotiations about data use that would elude any individual. Whereas property is typically placed in trust to manage its value, data (or data rights) would be placed in trust in part to create value.
Another difference can be found in the ease with which assets can typically be removed from a trust. Central to the trusts proposition is that individuals would be able to move their data rights between trusts, within an ecosystem of trust entities that provide a choice in different types of data use.
The ecosystem of data trusts that would enable individuals to make choices between different approaches to data use and management presupposes the ability to switch from one trust to another relatively easily, probably more easily than in traditional trusts.
These differences need not present a barrier to the development of data trusts. The history of trusts demonstrates the flexibility of this branch of law, and trusts can have a range of properties or ways of working that are designed to match the intent of their creators.
Alternatives to trust law
The fiduciary duties owed by trustees to beneficiaries can be achieved by other legal models. For example, contractual frameworks or principal-agent relationships, can create duties between parties, with strong consequences if those duties are not fulfilled. Regulators can also perform a function similar to fiduciary responsibilities, for example in cases where imbalances of market power might have detrimental impacts on consumers. However, each has its limitations. For example:
- Contracts allow use of data for a purpose. Coupled with an audit function, these can ensure that data is used in line with individual wishes, and – at least for simple data transactions – contracts would require less energy to establish than a trust. However, effective auditing relies on the ability to draw a line from the intention of those entering a contract to the wording of the contract then to its implementation. Given the complexity of patterns of data use – and the fact that many instances of undesirable data use arise from multiple inconsequential transactions – this function may be difficult to achieve. Due to their obligation of undivided loyalty, a trustee may be better placed and motivated to map intent to use and understand potential pitfalls arising
from the interactions between data transactions. - Agents can be tasked with acting on behalf of an individual, taking a fiduciary responsibility in doing so. However, the interaction between an individual and their agent does not accommodate as easily the collective dimension enabled by the establishment of a trust, and it is in this collective dimension that the ability to disrupt digital power relationships lies. Another issue associated with the use of agents is accountability. Structures would be needed to ensure that agents could be held accountable by individuals, if they failed in their responsibilities. In comparison, under trust law, the Courts of Chancery (and the associated institutional safeguards) present a much stronger accountability regime.
Many jurisdictions do not have an equivalent to trust law. However, they may have mechanisms that could fulfil similar functions. For example, while Germany does not operate a trust law framework, some institutions have fiduciary responsibilities built into their very structure, with institutions such as Sparkassen, banks that operate on a cooperative and not-for-profit basis, taking on a fiduciary responsibility for their customers. Studying such mechanisms might uncover ways of delivering the key functions of trust law – stewarding the rights associated with data and delivering benefits for individuals, communities and society with strong safeguards against abuse.
Developing data trusts
Recent decades have brought radical changes in patterns of data collection and use, and the coming years will likely see further changes, many of which would be difficult to predict today. In this context, society will need a range of governance tools to anticipate and respond to emerging digital opportunities and challenges. In conditions of uncertainty, trusts offer a way of responding to emerging governance challenges, without requiring legislative intervention that can take time to produce (and is more difficult to adapt once in place).
Trusts occupy a special place in the UK’s legal system, and the skills and experience of the UK’s legal community in their development and use means it is well-placed to lead the development of data trusts. The next wave in the development of these governance mechanisms will require further efforts to analyse the assets that will be held by a data trust, investigate the powers that trustees may hold as a result, and consider the different forms of benefit that may arise as a result. Those seeking to capture this opportunity will need to:
- clarify the limits of existing data rights
- identify lessons from other jurisdictions in the use of fiduciary responsibilities to underpin data governance
- support pilot projects that assess the feasibility of creating data trusts as a framework for data governance in areas of real-world need.
Problems and opportunities addressed by data trusts
Data trusts have the potential to address some of the digital challenges we face and could help individuals better position themselves in relationship to different organisations, offering new mechanisms for chanelling choices related to how their data is being used.
While organisations could also form data trusts, this section will deal only with data trusts where the beneficiaries are individuals (data subjects). Also, while trusts could manage rights over non-personal data, this section takes as starting point the opportunities coming from individuals delegating their rights (or beneficial interest therein) over personal data. In contexts where non-personal data is managed, the practical challenges in distinguishing personal and non-personal data need to be acknowledged, and it needs to be seen how managing mixed data sets influence the structure and running of a data trust.
There are a number of issues that might arise from setting up a data trust, which aims to balance the asymmetries between those who have less power and are more vulnerable (individuals or data subjects) and those who are in a more favoured position (organisations or data controllers). This section aims at briefly presenting a number of caveats in relation to data trusts and the ecosystem they create, however it should be noted that information asymmetries could also exist between individuals and trusts, not only between individuals and organisations.18
1. Purpose of the trust and consent
Trusts are usually established for defined purposes set out in a constitutional document. The data subjects will either come together to define their vision about the purposes of data use or will need to adhere to an established data trust and be well-informed about the purposes of the trust and how data or data rights are handled. In either case, it is of the utmost importance that those joining a data trust can do so in full awareness of the trust’s terms and aims.
This raises important ‘enhanced consent’ questions: what mechanisms, if any, are available to data trustees to ensure informed and meaningful consent is achieved? Will the lack of mechanisms for deliberation or consultation with beneficiaries involve liability for the trustees? What would the trustee role be in a participatory structure (active or purely managerial)? Might data trustees for instance draw upon the significant body of work in medical ethics to delineate best practice in this respect?
This set of questions is related to the issues raised in the next section, regarding the status, oversight and required qualifications of data trustees. Important questions arise around how expertise is attracted to this position when, as we will see below, the challenges for remunerating this role and the responsibilities and liabilities of trustees are significant.
2. The role of the trustee
The trustee will be in charge of managing the relationship between the trust’s beneficiaries and the organisations the trust interacts with. Trustees will have a duty of undivided loyalty to the beneficiaries (understood here as the data subjects whose data rights they manage) and they would be responsible for skilfully negotiating the terms of use or access to the beneficiaries’ data. They could also be held responsible if terms are less than satisfactory or if beneficiaries find fault with their actions (in which case the burden of proof is reversed, and it is for the data trustee to demonstrate that they have acted with undivided loyalty).
There are open questions as to if and how beneficiaries will be able to monitor the trustees’ judgement and behaviour and how beneficiaries will be able to identify fault when complex data transactions are involved. More complexity is added also if an ecosystem of data trusts is developed, where one person’s data is spread across several trusts.
At the same time, in the context of increased concerns coming from combining different datasets, in a scenario where one data trust manages a particular dataset about its beneficiaries and another trust manages a different dataset, where the combination of these two datasets could result in harm, should there be mechanisms for trusts to cooperate in preventing such harms? Or would trustees just inform beneficiaries of potential dangers and ask them to sign a liability waiver?
If and when a data trust relies on a centralised model (rather than a decentralised one, whereby the data remains wherever it is, and the data trustee merely leverages the data rights to negotiate access, etc.), one of the central attributions of the trustees will be to ensure the privacy and security of the beneficiaries’ data. Such a task would involve a high degree of risk and complexity (hence the likely preference for decentralised models).
It is unclear what type of technical tools or interfaces will be needed in order for trustees to access credentials in a secure way, for example, and who will make these significant investments in the technical layer. Potential inspiration could come from the new Open Banking ecosystem, where data sharing is enabled by secure Application Programming Interfaces (APIs) which rely on the banks’ authentication methodologies, so that third-party providers do not have to access users’ credentials.
Managing such demanding attributions raises questions related to what will be the triggers, incentives and training required for trustees to take up such a complex role. Should there be formal training and entry requirements? Could data trustees eventually constitute a new type of profession, which could give rise to a ‘local’ and potentially more nimble layer of professional regulation (on top of court oversight and potential legislative interventions), not unlike the multilayered regulatory structure that governs medical practice today?
3. Incentives and sustainability of data trusts
The data trust ecosystem model suggests the importance of competition between trusts for members, yet at this stage it is not clear how enough competition between trusts will emerge. At the same time, it is presumed that a data trust would work best when it operates on behalf of a large number of people. This gives the data trust a bargaining power position in relation to different organisations such as companies and public institutions. Will this create a dependence on network effects, and how can the negative implications be addressed?
Moreover, there are questions related to the funding model and incentives structure underlying the sustainability of data trusts. What will attract individuals to a data trust? For example, if the concern of the beneficiaries is to restrict and to protect data, will the trust be able to generate an income stream or will the trust rely on funding from other sources (e.g. from beneficiaries, philanthropists, etc.)? At the same time, if potential income streams are maximised depending on the use of the data, what are the implications for privacy and data protection?
In addition, what happens when individuals are simply unaware or uninterested in joining a data trust? Might they be allocated to a publicly funded data trust, on the basis of arguments similar to those that were relied on when making pension contributions compulsory? If so, what would constitute adequate oversight mechanisms?
When individuals are interested in joining a data trust, will they be lured by the promise of streamlining their daily interaction with data-reliant service providers, effectively relying on data trusts as a lifestyle, paid-for intermediary service providing peace of mind when it comes to safeguarding personal data? Will individuals be motivated to join a data trust in order to contribute to the common good in a way that does not entail long-term data risks? Will there be monetary incentives for people joining a data trust (whereby individuals would obtain monetary compensation in exchange for providing data)? Should some incentives structures – such as monetary rewards – be controlled and regulated, or in some cases altogether banned?
There are a number of possible funding models for data trusts:
- privately funded
- publicly funded
- charging a fee or subscription from data trust beneficiaries (the individuals or data subjects) in return for streamlining and/or safeguarding their data interactions
- charging a fee or subscription from those who use the data (organisations)
- charging individuals for related services
- a combination of the above.
The different funding options will have both sustainability, and larger data ecosystem implications. If the trust needs to generate revenue by charging for access to the data it stewards or for related services, the focus might start to levitate towards the viability and performance of the trust. The trusts’ performance will correlate with the demand side (organisations using the trust’s beneficiaries’ data), how many people join a data trust (potentially reinforcing network effects) and which data trust can compete better. Will these interdependencies diminish the data trusts’ role as a rebalancing tool for adjusting asymmetries of power and consolidating the position of the disadvantaged?
At the same time, if the data trust operates on a model where the beneficiaries are charged for the service, much depends on how that service is understood. If the focus is on monetary rewards, and the latter are not regulated, the expectations of return from the data trust will increase, hence affecting the dynamics of the relationships. For example, if the data trusts’ funding model implies companies pay back profit on the data used, they will have to make a number of decisions regarding their profitability and viability on the market. Will this reinforce some of the business models that are considerably criticised today, such as the dominant advertising based model?
In the case of publicly funded data trusts, public oversight mechanisms and institutions will need to be developed. At the moment, it is unclear who will be responsible for ensuring funds are transparently allocated based on input from individuals, communities and data-sharing needs. The currently low levels of data awareness also raise concerns about ways of building genuine and adequate engagement mechanisms. Further, the impact, benefit, results or added value created by the data trust will need to be demonstrated. This calls for building transparency and accountability means that are specific to publicly funded data trusts, grafting themselves on top of existing fiduciary duties (and Court oversight mechanisms).
4. Opportunities for organisations to engage with data trusts
Data trusts could offer opportunities for commercial or not-for-profit organisations in a variety of ways. Some of the benefits have been briefly mentioned in the introductory section, pointing to reputational benefits, legal compliance and future-proofing data governance practices. In this respect, one may imagine a scenario whereby large corporate entities (such as banks for instance) are keen to go beyond mere regulatory compliance by sponsoring a data trust in a bid to show how seriously they take their ethical responsibilities when it comes to personal data.
Such a ‘sponsored data trust’ would be strictly separate from the bank itself (absence of conflict of interest would have to be very clear). It could be flagged as enabling the bank’s clients to ‘take the reins’ of their data and benefit from insights derived from this data. All the data that would normally be collected directly by the bank would only be so collected on the basis of terms and conditions negotiated by the data trustee on behalf of the trust’s beneficiaries. The trustee could also negotiate similar terms (or negotiate to revise terms of existing individual agreements) with other corporate entities (supermarkets for instance).
Other potential benefits for corporate and research bodies are around the trusts’ ability to enable access to potentially better quality data that fits organisations’ needs and enables a more agile use of data. This reduces overhead and provides more ease of mind, based on the trustees’ fiduciary responsibility to the data subjects. A trustee would be able to spot and prevent potential harms, therefore reducing liability issues for organisations that could have otherwise arisen from engaging with individual data subjects directly. At the same time, trusts offer a way of responding to emerging governance challenges, without requiring legislative intervention that can take time to produce (and is more difficult to adapt once in place). A broader discussion about opportunities for commercial or not-for-profit organisations could be
considered for a future report.
Mock case study: Greenfields High School
Greenfields High School is using an educational platform to deliver teaching materials, with homework being assigned by online tools that track student learning progress, for example recording test scores. The data collected is used to tailor learning plans, with the aim of improving student performance.
Students, parents, teachers and school leadership have a range of interests
and concerns when it comes to these tools:
- Students wish to understand what data is collected about them, how it is used and for how long it is kept. Parents want assurances about how their children’s data is used, stored, and processed.
- Parents, teachers, and school leadership wish to compare their performance against that of other schools, by sharing some types of data.
- The school wants to keep records of educational data for all pupils for a number of years to track progress. It also wants to be able to compare the effectiveness of different learning platforms.
- The company providing the learning platform requires access to the data to improve its products and services.
How would a data trust work?
A data trust is set up, pulling together the rights pupils and parents have over the personal data they share with the education platform provider. It tasks a data trustee with the exercise of those rights with the aim of negotiating the terms of service to the benefit and limits established by the school, parents and pupils. It also aims at maximising the school’s ability to evaluate different types of tools (and possibly pool this data with other schools), within an agreed scope of data use that maintains the pupils’ and parents’ confidence that they are minimising the risks associated with data sharing.
The trust will be able to leverage its members’ rights to data portability and/or access (under the GDPR) when the school discusses onwards terms of data usewith the educational platform service provider.
The data trust includes several schools who have joined a group of common interest in a certain educational approach. This group is overseen by a board. One of the persons sitting on that board is appointed as data trustee.
Chapter 2: Data cooperatives
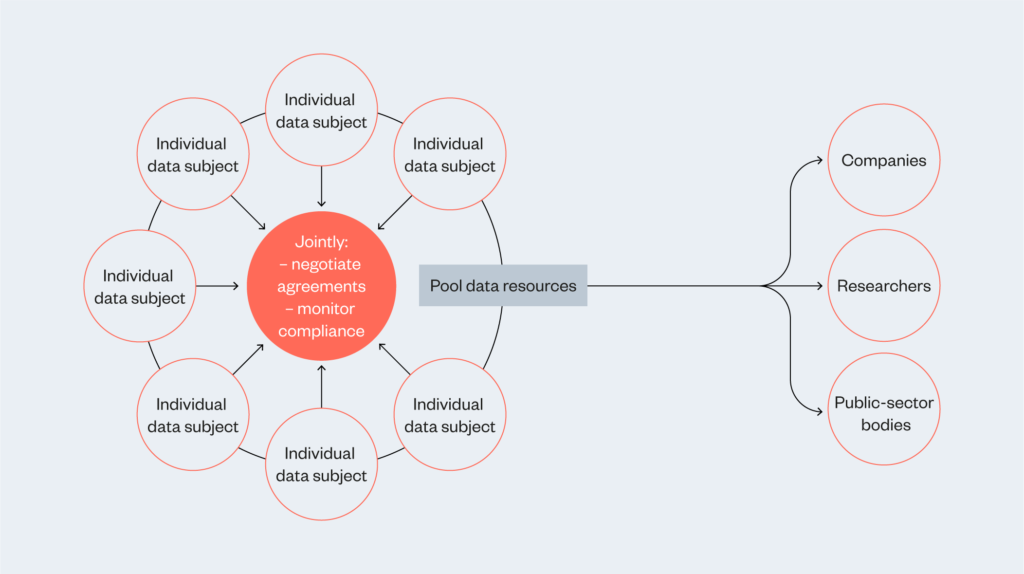
Why data cooperatives?
The cooperative approach is attractive in situations where there is a desire to give members an equal stake in the organisation they establish and an equal say in its management, as for example with traditional mutuals – businesses owned by and run for the benefits of their members – which are common in financial services, such as building societies. As the business is owned and run by its members, the cooperative approach can be seen as a solution to a growing sense of powerlessness people feel over businesses and the economy.19
The cooperative approach in the context of data stewardship can be explored in examples where groups have voluntarily pooled data resources in a commonly owned enterprise, and where the stewardship of that data is a joint responsibility of the common owners. The aim of such enterprises is often to give members of the cooperative more control over their data and repurpose the data in the interests of those represented in it, as opposed to the erection of defensive restrictions around the use of data to prevent activities that conflict with the interests of data subjects (especially but not exclusively with respect to activities that threaten to breach their privacy). In other words, cooperatives tend to have a positive rather than a negative agenda, to achieve some goal held commonly by members, rather than to avoid some outcome resisted by them.
This chapter looks at some examples of data cooperatives, the problems and opportunities they address and patterns of data stewardship. It explores the structure and characteristics of cooperatives and provides a summary of the challenges presented by the cooperative model, together with descriptions of alternative approaches.
What is a cooperative?
A cooperative typically forms around a group that perceives itself as having collective interests, which it would be better to pursue jointly than individually. This may be because they have more bargaining power as a collective, because some kind of network effect means the value for all increases if resources are pooled, or simply because the members of the cooperative do not want to cede control of the assets to those outside the group. Cooperatives are typically formed to create benefits for members or to supply a need that was not being catered for by the market.
The International Cooperative Alliance or ICA20 is the global steward of the Statement on the Cooperative Identity, which defines a cooperative as an ‘autonomous association of persons united voluntarily to meet their common economic, social, and cultural needs and aspirations through a jointly-owned and democratically controlled enterprise.’
According to the ICA there are an estimated three million cooperatives operating around the world,21 established to realise a vast array of economic, social and cultural needs and aspirations. Examples include:
- Consumer cooperatives, which provide goods and services to their members/owners, and so serve the community of users. They value service and low price above profit, as well as being close to their customers. They might produce goods such as utilities, insurance or food, or services such as childcare.22 They might be ‘buyers’ clubs’, intended to enable the amalgamation of buyers’ power in order to reduce prices. Credit unions are also examples of consumer cooperatives, which mutualise loans based on social knowledge of local conditions and members’ needs, and are owned by the members and therefore able to devote more capital to members’ services rather than profits for external owners.23
- Housing cooperatives take on a range of forms, from shared ownership of the entire asset to management of the leasehold or managing tenants’ participation in decision-making.
- Worker cooperatives, where the entity is owned and controlled by employees.
- Agricultural cooperatives, which might be concerned with marketing, supply of goods or sharing of machinery on behalf of members. Many agricultural cooperatives in the US are of significant size: the largest, for example, had revenues of $32 billion in 2019.24 These cooperatives are formed to address a market power imbalance created by small producers and large distributors or buyers – power asymmetries that are also experienced by individuals in the data ecosystem.
The estimated three million cooperatives subscribe to a series of cooperative values and principles.25 Values typically include self-help,self-responsibility, democracy, equality, equity, solidarity, honesty and transparency, social responsibility and an ethics of care.26 Fundamental cooperative characteristics include: voluntary and open membership, democratic member control (one member, one vote), member benefit and economic participation (with surpluses shared on an equitable basis), and autonomy and independence.27
Cooperatives in the UK: characteristics and legal structures
According to Co-operatives UK28 there are more than 7,000 independent cooperatives in the UK, operating in all parts of the economy and collectively contributing £38.2 billion to the British economy.29
UK law does not provide a precise definition of a cooperative, nor is there a prescribed legal form that a cooperative must take. According to Co-operatives UK, a cooperative in the UK can generally be taken to be any organisation that meets the ICA’s definition of a cooperative and espouses the cooperative values and principles set out in the Statement on the Cooperative Identity.30 This status can be implemented via many different unincorporated and incorporated legal forms. Deciding which one is best will depend on a number of case-specific factors, including the level of liability members are willing to expose themselves to, and the way members want the cooperative to be governed.
A possible, and seemingly obvious, choice of legal form is registering as a cooperative society under the Co-operative and Community Benefit Societies Act 2014.31 This Act consolidated a range of prior legislation and helped to clarify the legal form for cooperative societies in the UK (different rules apply for registration of a credit union under the Credit Unions Act 1979). Subsequent guidance from the Financial Conduct Authority (FCA) on registration, and the Charity Commission on share capital withdrawal allowances, have further clarified and codified the regulatory regime for cooperative societies. In particular, to register as a cooperative society under the Act, it must be a ‘bona fide co-operative society’. The Act however does not precisely define what is included as a bona fide co-operative society. In its guidance, the FCA adopted the definition in the ICA’s Statement on the Cooperative Identity and says it considers it an indicator that the condition for registration is met where the society puts the values from the ICA’s Statement into practice through the principles set out in the Statement.32
The cooperative society form is widely used by all types of cooperatives. Registration under the 2014 Act imposes a level of governance through a society’s rules and a level of transparency through certain reporting requirements that has some common ground with Companies Acts requirements for other types of organisations.
However, as noted above, this is not the only legal form available for a cooperative, and alternative legal forms that can be used include a private company limited by shares and a private company limited by guarantee. For a more detailed exploration of the options Co-operatives UK has published guidance,30 and has a ‘Select-a-Structure’ tool on its website.34
Cooperatives and data stewardship
For the purposes of this report we see data cooperatives as cooperative organisations (whatever their legal form) that have as their main purpose the stewardship of data for the benefit of their members, who are seen as individuals (or data subjects).35 This is in contrast to stewardship of data primarily or exclusively for the benefit of the community at large.
Under the Co-operative and Community Benefit Societies Act 2014,if the emphasis is to benefit a wider community then the appropriate legal form would be a community benefit society.
As for cooperative societies, other legal forms could also be used to achieve the same aims and deciding which is best will depend on a number of case-specific factors. However, that is not to say that a cooperative whose aim is to benefit its members might not also benefit wider society – we will see examples later (e.g. Salus Coop) where members’ benefits are also intended to benefit wider society. Indeed, where members see the wider benefits as their own priorities (as with philanthropic giving), the distinction between members’ benefits and social benefits may be hard to discern.
In a data cooperative, those responsible for stewarding the data act in the context of the collective interests of the members and – depending on how the cooperative is governed – may have to advance the interests of all members at once, and/or achieve consensus over whether an action is allowed.
The stewardship of data may be (and with increasing tech adoption is increasingly likely to be) a secondary function to the main purpose of a cooperative. For example, if the cooperative is enabled by technology, such as through the use of a social media platform, then it will routinely produce data that it may be able to capture. If so, this data might be of use to the cooperative’s own operations in future. Some of these groups have been described as social machines.36
Examples of areas where valuable data may be produced are medical applications, interest groups, such as religious or political groups, fitness, wellbeing and self-help groups, particularly including the quantified self movement, and gaming groups. While questions around the management and use of data produced by cooperatives through their ordinary business will become increasingly important (as with other types of organisations that produce data as part of their business) this is not our focus here.
Data cooperatives versus data commons
In their collaborative, consensual form, data cooperatives are similar to data commons. A commons is a collective set of resources that may be: owned by no one; jointly owned but indivisible; or owned by an individual with others nevertheless having rights to usage (as with some types of common land). Management of a commons is typically informal, via agreed institutions and social norms.37
The distinction between commons and cooperatives is blurred; one possible marker is that a commons is an arrangement where the common resource is undivided, and the stakeholders all have equal rights, whereas in a cooperative, the resources may have been owned by the members and brought into the cooperative. The cooperative therefore grows or shrinks as resources are brought in or out as members join or leave, whereas the commons changes organically, and its stakeholders use but do not contribute directly to the resources.
In the case of data, the cooperative model would imply that data was brought to and withdrawn from the cooperative as members joined and left. A data commons implies a body of data whose growth or decline would be independent of the identity and number of stakeholders.
The governance of commons can provide sustainable support for public goods,38 and data commons are often written and theorised about.39 However, as this report is focused on existing examples of practice, in this respect it is difficult to identify actual paradigms of data commons (either intended as such, or merely as institutions whose governance happens to meet Ostrom’s principles).40 Hence, while data commons may possibly be an exciting way forward, and while there are indeed some domains where a commons approach might be appropriate (such as OpenStreetMap and Wikidata), the prospects of their emergence from the complex legal position surrounding data at the time of writing are not strong, so will not be discussed further in this report.
Examples of cooperatives as stewards of data
For the purpose of this report, data cooperatives are seen as cooperative organisations (irrespective of their legal form) that have as their main purpose the stewardship of data for the benefit of its members. This section focuses on examples from the data cooperative space, sharing remarks on governance, approach to data rights and sustainability. Although they take different legal forms (particularly as they are not all UK-based projects) all are working along broadly cooperative principles.
1. Salus Coop
Salus Coop is a non-profit data cooperative for health research (referring not only to health data, but also lifestyle-related data more broadly, such as data that captures the number of steps a person takes in a day), founded in Barcelona by members of the public in September 2017. It set out to create a citizen-driven model of collaborative governance and management of health data ‘to legitimize citizens’ rights to control their own health records while facilitating data sharing to accelerate research innovation in healthcare’.41
Governance: Salus have developed a ‘common good data license for health research’ together with citizens through a crowd-design mechanism,42 which it describes as the first health data-sharing license. The Salus CG license applies to data that members donate and specifies the conditions that any research projects seeking to use the member data must adhere to.43 The conditions are:
- health only: the data will only be used for biomedical research activities and health and/or social studies
- non-commercial: research projects will be promoted by entities of general interest, such as public institutions, universities and foundations
- shared results: all research results will be accessible at no cost maximum privacy: all data will be anonymised and unidentified before any use
- total control: members can cancel or change the conditions of access to their data at any time.
Data rights: Individual members will have access to the data they’ve donated, but Salus will only permit third-party access to anonymised data. Salus describes itself as committed to ensuring, and requires researchers interacting with the data to ensure, that: individuals have the right to know under what conditions the data they’ve contributed will be used, for what uses, by which institutions, for how long and with what levels of anonymisation; individuals have the right to obtain the results of studies carried out with the use of data they’ve contributed openly and at no cost; and any technological architecture used allows individuals to know about and manage any data they contribute.
Note therefore that Salus meets the definition of a data cooperative, as it provides clear and specified benefits for its members – specifically a set of powers, rights and constraints over the use of their personal health data – in such a way as to also benefit the wider community by providing data for health research. Some of these powers and rights would be provided by GDPR, but Salus is committed to providing them to its members in a transparent and usable way.
Sustainability of the cooperative: Salus has run small-scale studies since 2016, and promotes itself as being about to generate ‘better’ data for research (in relation, for example, to surveys), creating ‘new’ datasets (such as heartbeat data generated through consumer wearables) and ‘more’ data than other approaches. However, the cooperative’s approach to sustainability is unclear. In June 2021, it aims to publicly launch CO3 (Cooperative COVID Cohort), a project stream to help COVID-19 research,44 and it aims to capture a fraction of the value generated by providing data for researchers to sustain itself.
2. Driver’s Seat
Driver’s Seat Cooperative LCA (‘Driver’s Seat’)45 is a driver-owned cooperative incorporated in the USA in 2019,95 with ambitions to help unionise or collectivise the gig economy. It helps gig-economy workers gain access to work-related smartphone data and get insight from it:
it is ‘committed to data democracy … [and] empowering gig workers and local governments
to make informed decisions with insights from their rideshare data.’
The Driver’s Seat app, available only in the US, allows on-demand drivers to track the data they generate, and share it with the cooperative, which can then aggregate and analyse it to produce wider insights. These are fed back to members, enabling them to optimise their incomes. Driver’s Seat Cooperative also collects and sells mobility insights to city agencies to enable them to make better transportation-planning decisions. According to the website, when ‘the Driver’s Seat Cooperative profits from insight sales, driver-owners receive dividends and share the wealth’.
One issue here, unexplored on the website, is that in the ride-hailing market, in geographically limited areas, drivers may indeed have common interests, but they are also in competition with each other for rides. Access to data could also open up job allocation to scrutiny, something that is concerning drivers in the UK, where a recent complaint against Uber has been brought by drivers who want to see how algorithms are used to determine their work, on the basis that this could be allowing discriminatory or unfair practices to go unchecked.46
Governance: Driver’s Seat Cooperative is an LCA or Limited Cooperative Association in the US, so will be governed by the legislation and rules associated with this type of entity. It is not obvious from the website what the terms and conditions are for becoming a member of the cooperative and how it is democratically controlled.
Data rights: Driver’s Seat is headquartered outside the jurisdiction of the GDPR. A detailed privacy notice sets out how Driver’s Seat collects and processes personal data from its platform, which includes its website and the Driver’s Seat app.47 By accessing or using the platform the user consents to the collection and processing of personal data according to this notice.
Sustainability of the cooperative: Driver’s Seat is a very new cooperative and a graduate of the 2019 cohort of the start.coop accelerator programme in the US.48 PitchBook reports that it secured $300k angel investment in August 2020.49 According to its website, Driver’s Seat sells mobility insights to city agencies, which is doubtless at least part of its plan for long-term sustainability. It is not obvious from the website if there is any further investment requirement from the driver-owners of the cooperative above and beyond sharing their data. The app itself is free.
3. The Good Data (now dissolved)
The Good Data Cooperative Limited (‘The Good Data’)50 was a cooperative registered in the UK that developed technology
to collect, pool, anonymise (where possible) and sell members’ internet browsing data on their own terms, to correct the power imbalance between individuals and platforms (selling ‘on fair terms’).51 Members participated in The Good Data by donating their browsing data through this technology, so that the cooperative could trade with it anonymously enabling the cooperative to raise funds to cover costs and fund charities.52
As with Salus Coop, The Good Data provided benefits for members while simultaneously promising potential benefits for the wider community (and indeed many of those wider benefits would also be reasons for members to join).
Governance: The Good Data was registered as a cooperative society under the Co-operative and Community Benefit Societies Act 2014, and accordingly was subject to the requirements of that Act and had to be governed according to its rules filed with the FCA. The Good Data determined which consumers should receive the data, and made decisions about what to sell and how far to anonymise on a case-by-case basis. It declined to collect data from ‘sensitive’ browsing behaviour, which included looking at ‘explicit’ websites, as well as health-related and political sites.53 According to The Good Data’s last annual return filed at the FCA,54 The Good Data had three directors. Members had online access to all relevant information and based on that could present ideas or comments in the online collaboration platform at any time. Members could also participate in improving existing services and an Annual General Meeting was held once a year.
Data rights: It is hard to say what rights were invoked here. If the data has been anonymised, it is no longer personal data under the GDPR. If the data is likely to be re-identifiable or to be attributed to an individual, then the data is pseudonymised (and thus still personal data).
Sustainability of the cooperative: Revenue was generated from the sale of anonymised data to data brokers and other advertising platforms, and the profits redistributed, to maintain the system, and for social lending in developing countries. Decisions about the latter were determined by cooperative members. However, the model proved not to be sustainable, as its website announces the dissolution of the cooperative: ‘we thought that the best way to achieve our vision was by setting up a collaborative and not for profit initiative. But we failed to pass through the message and to attract enough members.’ The Request to Cancel filed at the FCA55
also indicated that this was due to Google rejecting The Good Data’s technology, which was intended to allow members to gain ownership of their browsing data from its Chrome Webstore, and being unable to build a new platform to pursue this objective given the required technical complexity and lack of sufficient human and financial resources.
Created with similar intentions, Streamr56 advocates for the concept of ‘data unions’ and seeks to create financial value for individuals by creating aggregate collections of data in a similar way, including focusing on web browser data – it’s unclear whether this effort will prove more sustainable than The Good Data.
Problems and opportunities addressed by data cooperatives
From the examples surveyed above, data cooperatives appear mostly concerned with personal data (as opposed to non-personal data) and, in general, are directed towards giving members more control over data they generate, which in turn can be used to address existing problems (including social problems) or open up new opportunities. This is very much in line with the purpose of the cooperative model generally. For example, Salus Coop allows members to control the use of their health data, while opening up new opportunities for health research. The Good Data was aimed at giving data subjects more control and bargaining power with respect to data platforms, to get a better division of the economic benefits. Unionising initiatives, such as Driver’s Seat, have focused largely but not exclusively on the gig economy, and using data to empower workers and enable them to optimise their incomes and working practices.
Many data cooperatives seek to repurpose existing data at the discretion of groups of people, to create new cooperatively governed data assets. In this respect, they tend to pursue a positive agenda that uses data as a resource. For example, Driver’s Seat brings in data from sources such as rideshare platforms and sells mobility insights based on this data, sharing profits among members. The Good Data’s business model was to trade anonymised internet browsing data. Some data cooperatives do also seek to refactor the relationship between organisations that hold data and individuals who have an interest in it. The Good Data’s technology to collect internet browsing data was also designed to give members using it more privacy by blocking data trackers.
See also RadicalxChange’s proposal in Annex 3, which contains elements of all three legal mechanisms presented in this report. Described as a conceptual model, it would shake up the status quo even more by making corporate access to data subject’s data the cooperative decision of a Data Coalition.
Although privacy is usually a feature they respect, it is hard to find data cooperatives intending to preserve privacy as a first priority, through limiting the data that is collected and processed. Indeed, this is rather a negative aim, constraining the use of data, rather than pursuing a positive agenda and opening up a new purpose for the data.
More often data anonymisation techniques and privacy-preserving technologies are referred to, however these areas require research and investment,57 especially given the legal uncertainty as to what it takes for companies to anonymise data in the light of the GDPR, and the complexity of the task of anonymisation itself, which requires a thorough understanding of the environment in which the data is held.58
Examples that we have surveyed could be said to recognise the balance between 1) complete privacy and 2) the potential benefits to the individual from collecting and processing personal data and communicating the insights to the individual, and 3) in those individuals then being able to better influence the market and receive a better division of the economic benefits (e.g. through selling the data and/or insights).
Challenges
The cooperative approach appeals to a sense of data democracy, participation and fair dealing that may inform and shape the structuring of any data-sharing platform but, in themselves, cooperatives face a number of challenges:
1. Uptake
While the examples we’ve analysed represent experimentation around data cooperatives, there doesn’t appear to be significant uptake and use of them, and little evidence that they will scale to steward significant amounts of data within a particular geography or domain. This is perhaps unsurprising, given a number of challenges to uptake, as cooperatives require motivated individuals to come together and actively participate by:
- recognising the significance of the problem a cooperative is trying to solve (resonance challenge)
- being interested enough to find or engage with a data cooperative as a means to solve the problem (mobilisation challenge)
- trusting a particular cooperative and its governance as the best place to steward data (trust challenge)
- being data literate enough to understand the implications of different access permissions, and/or willing to devote time and effort to managing the process. Because cooperatives presume a role for voluntary members and rely on positive action to function, this is more likely to work in circumstances where all participants
are suitably motivated and willing to consent to the terms of participation (capacity challenge).
The examples surveyed offer some insights into how these elements of the uptake challenge could be met. A strong common incentive could be enough to meet the mobilisation challenge by employing bottom-up attempts to create data cooperatives. For example, Driver’s Seat could use the interest and perceived injustice among gig-economy workers in their working conditions and pay to build an important worker-owned and controlled data asset. If endorsed or even delivered by trusted institutions such as labour unions this could further enhance uptake.
Other examples, such as The Good Data, were aiming to mobilise people around the concept of correcting a power imbalance between individuals and platforms. In a similar vein, the aim of the RadicalxChange model (discussed further in Annex 3) works at the level of power imbalance, with an added requirement for legislative change to make their data coalitions possible and reduce the market failure of data.59
Such a top-down approach could create challenges not too far removed from the issues that many data cooperatives seek to address, such as around the selected default sharing and processing options that the data would be subject to, and the abilities of people to opt-out or switch. Relying on individual buy-in for success may never move the needle, without more of a purpose or affiliation to coalesce around. Changing the world for the better is more abstract and often less motivating than changing one’s particular corner of it for one’s (and others’) benefit.
These uptake challenges are not unique to cooperatives and are experienced by many other data-stewardship approaches that focus on empowering individuals in relation to their personal data. However, potentially, the features of a cooperative approach to data stewardship could themselves hinder the uptake and scalability of a data cooperative
initiative. These are discussed next.
2. Scale
There are additional features of cooperatives that may make this approach unsuitable for large-scale data-stewardship initiatives:
a. Democratic control and shared ownership
The cooperative model presumes shared ownership. The implied level of commitment may be an asset to the organisation, but may similarly make it hard for the model to scale if everyone wants their say.
The cooperative model also favours democratic control. Depending on how the cooperative is established and governed, the democratic control of cooperatives could be too high a burden for all but the most motivated individuals, limiting its ability to scale. Alternatively, where a cooperative has managed to scale, this approach could become too unwieldy for a cooperative to effectively carry out its business in a nimble and timely fashion.
Democracy and ownership also need to be balanced by a constitution. It may aim for equal say for members (one member, one vote), or alternatively it may skew democratic powers toward those members with more of a commitment (e.g. based on the amount of data donated).
Questions need to be resolved about what members vote for – particular policies, or simply for an executive board. Can the latter restriction, which will lead to more efficient decision-making, still enable individual members to feel the commitment to the cause that is needed to meet
the mobilisation challenge? If, on the other hand, members’ votes feed directly into policy, can the cooperative sustain sufficient policy coherence to meet the trust challenge?
b. Rights, accountability and governance
To establish and enforce rights and obligations, a cooperative needs to be able to use additional contractual or corporate mechanisms, and this requires members to engage and understand their rights and obligations. This is particularly important where data is concerned, given legal duties under legislation such as the Data Protection Act 2018, which implements the GDPR in the UK.
Cooperatives can create a large audience of members who can demand accountability and these members may be exposed themselves to personal liability, with associated challenges to manage potential proliferation of claims and fear of unjust proceedings.
Cooperatives may establish high levels of fiduciary responsibility but do not inherently determine particular governance standards or establish clear management delegation and discretion. Registration under the Co-operative and Community Benefit Societies Act 2014
imposes a level of governance that partially echoes the greater body of legislation applicable to registered companies under the Companies Acts. Registration as a company under the Companies Acts will import a broader array of governance provisions.
With respect to data, governance is a particularly sensitive requirement, especially as a cooperative scales. If a cooperative ended up holding a large quantity of data, this may become extremely valuable as network effects kick in. The cooperative would certainly need a level of professionalism in its administration to prosper, especially if its mission required it to negotiate with large data consumers, such as social networks. Moreover, the overarching governance of the administrators of the cooperative would need to be addressed. For example, there could be a data cooperative board with each individual having ownership shares in the cooperative based on the data contributed (which in turn would need a quasi-contractual model to define the role of the board and its governance role regarding data use).
Failure of governance may also leave troves of data vulnerable, if the proper steps have not been taken. In one recent incident, a retail cooperative venture in Canada called Mountain Equipment Co-Op was sold to an American private-equity company from underneath its five million members, after years of poor financial performance (losing CAD$11 million in 2019), with the COVID-19 pandemic as the last straw. The board felt that the sale was the only alternative to liquidation, although the decision was likely to be challenged in court.60 This case throws up data issues specifically – does the buyer get access to data
about the members, for example? But the main point is that a data cooperative managing a large datastore effectively and securely might well have to endure significant costs (e.g. for security), and will need a commensurate income.
If that income could not be secured, could the cooperative members prevent the sale of the cooperative – and therefore the data – to a predator? Under UK law, the assets of a cooperative should be transferred, at least in some circumstances, to a ‘similar’ body or organisation with similar values if and when it is wound up. Sometimes even an asset lock can be involved under Community Interest Company law. The extent of legal restraint on the disposal of the assets of the cooperative will of course depend on how it is defined and incorporated, and the sensitivity of the data should be reflected in the care with which the fate of the data is constrained. There may be legal protections, but it is still worth pointing out that the very existence of the data cooperative, as a single point of access to the data, may represent a long-term vulnerability.
c. Financial sustainability
Cooperatives do not easily lend themselves to development funding other than grant aid or pure philanthropy. In combination with the mobilisation challenge this suggests financial sustainability is likely to be a significant issue.
One problem this creates for many cooperatives is that they have to fall back on internally generated resources (i.e. donated by the members). Without a substantial and sustainable income, a cooperative will find it difficult to recruit capable managers and administrators, and so will be forced to form committees selected from the membership. Without capable managers, a cooperative will be less able to generate income and manage resources effectively, and, for example, will be less able to raise external capital because of a low rate of expected return.
These factors constrain the scope for a cooperative to mature and operate in a commercial environment when compared with other models.
Mechanisms to address the challenges
The cooperative structure has longstanding heritage and diverse application, as demonstrated by the examples we have analysed, and ready appeal because of the inherent assumptions of common economic, social and cultural purpose. It is a natural mechanism by which an enterprise can be owned by people with a common purpose and managed for the benefit of those who supply and use shared services.
Recognising the challenges identified above that are inherent in a cooperative structure, we observe that cooperatives often rely on contract or incorporation to establish rights, obligations and governance, and either route might be selected as the preferred form while still
seeking to capture some of the essence of a cooperative through stated purpose, rights, obligations and oversight. However, neither is perfect – or, put another way, each, by diluting the cooperative ideal, may reintroduce some of the challenges that the cooperative model
was designed to address. These mechanisms are:
- The contractual model, where all rules for the operation of the data platform should be set down in bilateral (or multilateral) agreements between data providers and data users. This, when combined with the fact that each party would need to take action on its own behalf to enforce the terms of these agreements against any counterparties,
imposes a burden on participants to negotiate agreements and encourages participants to negotiate specific terms. It therefore has limited utility and is restricted to relatively limited groups of participants of similar sophistication, and may be vulnerable to the mobilisation or the capacity challenge. - The corporate model, often adopted in the form of a company limited by guarantee to underpin a cooperative, to achieve what a contractual model offers with additional flexibility, scalability and stability that is lacking from that model. This model may run into the trust challenge, however. In conceptual terms, data providers are being
asked to give up a degree of control over the data they are providing in return for the inherent flexibility, scalability and stability of the structure. They will only do so if they feel they can trust the structure or organisation that has been set up to effect this, which can be offered via a combination of clear stated purpose of the institution, the
reporting and accountability obligations of its board and an additional layer of oversight by a guarantor constituted to reflect the character of participants and charged with a duty to review and enforce due performance by the board. In time that might be supplemented by a suitably constituted, Government-sponsored regulator.
Although there is currently no obstacle in the way of data cooperatives – the law is in place, the cooperative model well-established – we can see a number of challenges to uptake, growth, governance and sustainability. The problem is rendered doubly hard by the fact that some of the challenges pull in different directions. For instance, the capacity challenge might be met by a division of labour, hiving off certain decision-making and executive functions, but then this might lead to the emergence of the trust challenge as the board’s decisions come under scrutiny. Failure to meet the mobilisation challenge could result in the members being as alienated from the stewardship of their data by the data cooperative as they were by other more remote corporate structures, but addressing the mobilisation challenge might lead to an
engaged set of members developing hard-to-meet expectations about the level of involvement they could aspire to, consistent with streamlined decision-making.
Mock case study: Greenfields High School
Greenfields High School and other educational facilities are interested in coordinating educational programs to meet the needs of their learners and communities in a way that complements and strengthens school programmes. All educational institutions use online educational tools to tailor learning plans for improving student performance and see a real opportunity to better serve their community through data sharing.
Greenfields High School proposes to the other educational boards to convene and explore the idea of pooling resources together for achieving these goals. They all have a shared interest in working together to gain better insights as to how they might improve educational outcomes for their community members.
In an act of good governance, educational facilities consult with their students, parents and teachers, and together they develop the rules and governance of the cooperative:
- Members of the community vote on the collaborative agreement between
educational facilities and decide what data can be shared and for what
purposes. The agreement is transparent about what data is collected, stored,
processed and how it is used. - The schools gain better understanding of the effectiveness of online tools
and educational plans throughout the learning cycle. - Where educational programmes are developed for the community based
on analysed data, members also decide on the price thresholds for such
educational services.
How would a data cooperative work?
A data cooperative is set up, pulling together the data educational facilities have from using digital technologies. Schools maximise their aims in comparing performance and understanding what digital tools are more effective. Students have a direct say into how their data is used and decide on the management and organisation of the cooperative.
Chapter 3: Corporate and contractual mechanisms
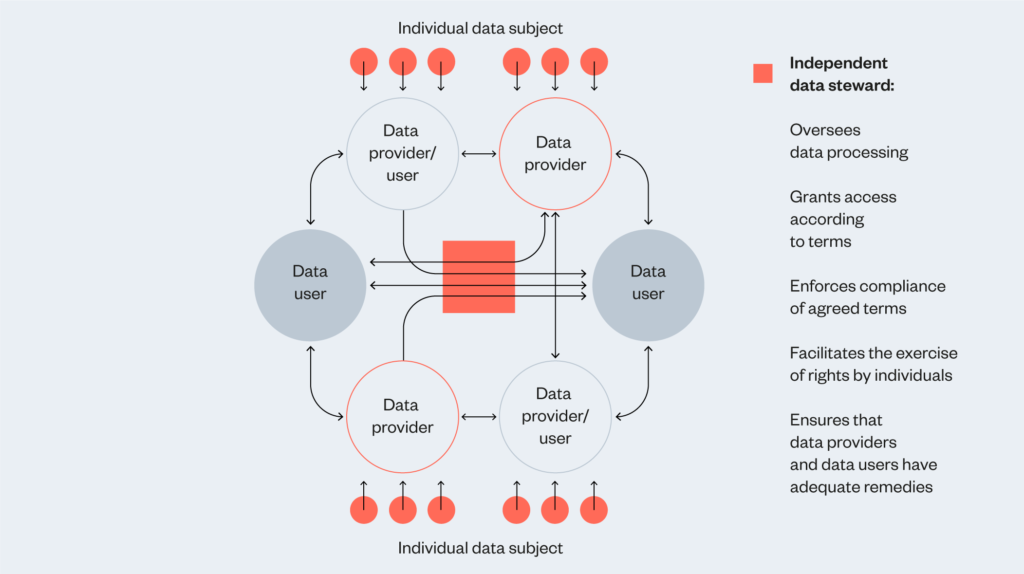
Corporate and contractual mechanisms can create an ecosystem of trust where those involved:
- establish a common purpose
- share data on a controlled basis
- agree on structure (corporate or contractual).
Why corporate and contractual mechanisms?
Corporate and contractual mechanisms can facilitate data sharing between parties for a defined set of aims or an agreed purpose. For the purposes of this report, it is envisaged that the overall purpose of a new data model will be to achieve more than mere data sharing, and
data stewardship can be used to generate trust between all the parties and help overcome relevant contextual barriers. The core purpose for data sharing will be wider than just the benefit gained by those who make use of data.
The role of the data model we envisage therefore includes:
- enabling data to be shared effectively and on a sustainable basis
- being for the benefit of those sharing the data, and for wider public benefit
- ensuring the interests of those with legal rights over data
- ensuring data is ethically used and in accordance with the rules of the institution
- ensuring data is managed safely and securely.
How to establish the right approach?
The involvement of an independent data steward is envisaged as a means of creating a trusted environment for stakeholders to feel comfortable sharing data with other parties who they may
not necessarily know, or with whom they have not had an opportunity to develop a relationship of trust.
Incentives for allowing greater access to data and for making best use of internal data will vary according to an individual organisation’s circumstances and sector. While increased efficiency, data insights, improved decision making, new products and services and getting value from data are potential drivers, there are also a number of challenges to sharing data:
- operating in highly competitive or regulated sectors, and concerns about undermining value in IP and confidential information
- a fear of being shown up as having poor-quality or limited data sets
- a fear of breaching commercial confidentiality, competition rules or GDPR
- a lack of knowledge of business models to support data sharing – access to examples, lessons learned and data sharing terms can help others feel able to share
- a lack of understanding of the potential benefits
- not knowing where to find the data or limited technical resource to implement (e.g. to extract the data and transform it into appropriate formats for ingestion into a data-sharing platform)
- fear of security and cybersecurity risks.
All these challenges can lead to inertia and lack of motivation.
Where a group of stakeholders see benefits in coming together to share data they will still need to be confident that this is done in a way that maintains a fair equilibrium between them, and that no single stakeholder will dominate the decision as regards the management and sharing of data. In order to establish and maintain the confidence of the stakeholders, they should all be fully engaged in the determination of what legal mechanism should be established. One or two stakeholders deciding and simply imposing a structure on other stakeholders is unlikely to engender a sense of trust, confidence and common purpose.
It is for this reason that we recommend the following approach.
1. Establish a clearly defined purpose
Establishing a clearly defined purpose is the essential starting point for stakeholders. Not only will a compelling statement of purpose engender trust among stakeholders, but it will also provide the ultimate measure against which governance bodies and stakeholders can check to ensure that the data-sharing venture remains true to its purpose. A clearly defined purpose can also help in assessing compliance with certain principles of the GDPR and other data-related regulations, including ePrivacy,61 or Payment Services Directive 2,62 which are often tested against the threshold of whether a data-processing activity, or a way in which it is carried out, is ‘necessary’ for a particular purpose or objective.
Any statement of purpose will need to be underpinned by agreement on:
- the types of data of which the data-sharing venture will take custody or facilitate sharing
- the nature of the persons or organisations who will be permitted access to that data
- the purpose for which they will be permitted access to that data
- the data-stewardship model and governance arrangements for overseeing the structure and processing, including to enforce compliance with its terms and facilitate the exercise of rights by individuals, and to ensure that data providers and data users have adequate remedies if compliance fails.
2. Data provider considerations
The data-sharing model has to be an attractive proposition for the intended data providers, with clear value and benefit, and without unacceptable risk. There will need to be strong and transparent governance to engender the level of confidence required to encourage data sharing. This will include confidence in not only the data provider’s ability to share the data with the data-sharing venture without incurring regulatory risk or civil liability, but also in its ability to recoup losses from the data-sharing set up or from relevant data users if the governance fails and this results in a liability for the data provider. Other considerations for governance could be related to managing intellectual property rights and control over products developed based on the data shared.
3. Data user considerations
As with the data providers, the data-sharing model must be an attractive proposition for intended data users. The data will need to be of sufficient quality (including accuracy, reliability, currency and interoperability) and not too expensive, for the data users to want to participate. Data users will also require adequate protection against unlawful use of data. For example, in relation to personal data, data users will typically have no visibility of the origins of the datasets and the degree of transparency (or lack of it) provided to the underlying data subjects. They will also be relying on the data providers’ compliance with the governance model to ensure that use of the contributed datasets will not be a breach of third-party confidentiality or IP rights.
4. Data steward considerations
The data steward’s role is to make decisions and grant access to data providers’ data to approved data users in accordance with the purpose and rules of the data-sharing model. The steward may take on additional responsibilities such as due diligence on data providers and users, and enforcement of the purpose of the data-sharing model; however, the way in which the model is funded and structured63 will impact on the extent of any such duties and who is practically responsible for performing them.
The responsibilities of the data steward may impact on considerations for the data providers and data users of the overall impact on risk and developing trust in the relationships.
5. Relationship/legal personality
The formal relationship between the parties will depend on the previous steps and the project structure that the stakeholders are comfortable with, based on the relevant risk, economic, regulatory and commercial considerations. Where there is no distinct legal personality, the
relationship may be governed by a series of contracts between the data providers, users and data steward – whether bilateral or a contract club with multiple parties. Where there is a legal personality, then as well as there being likely to be a series of contracts, there will be the documents establishing the relevant legal entity.
6. The rules
The rules of the data-sharing model will form part of the corporate and/or contractual relationship between stakeholders. This is discussed in more detail below in the ‘Mapping data protection requirements onto a data sharing venture’ section and in Annex 1 on ‘Existing mechanisms for supporting data stewardship’ when discussing regulatory mechanisms.
What is the appropriate legal structure?
As outlined, the aim is to design an ecosystem of trust. The data stewardship model will sit at the heart of this ecosystem. In this section we address two broad possibilities as to the legal form this should take:
- a contractual model: this would involve a standardised form of data sharing
agreement without the establishment of any form of additional legal structure or personality - a corporate model: this would involve the establishment of a company or other legal person, which would be responsible for various tasks relating to the provision of access to and use of data. The documents of incorporation would be supplemented by contractual arrangements.
In the contractual model, all of the rules for the operation of the data venture would need to be set down (and repeated) in a series of bilateral (or multilateral) agreements between data providers and data users. This, when combined with the fact that each party would need to take action on its own behalf to enforce the terms of that agreement against any counterparties, makes it likely that providers of data will only be willing to provide access to data on highly specific terms.
Where the aims of the stakeholders will require significant flexibility and scalability then a simple contractual model may not be the most appropriate. For example, a contractual model does not easily accommodate dedicated resources which may be required to govern and administer a growing data-sharing establishment (such as full-time employees, for which an employing entity is required). Also, an independent entity may find it easier to vary the rules of participation, or make other changes for the benefit of all, as the model evolves or laws change. Whereas a multilateral contractual arrangement may require protracted negotiation amongst the various stakeholders who each bring their own commercial objectives to the discussion.
In the corporate model, there is a degree of flexibility and scalability that is lacking from the contractual model. This model requires a greater degree of trust on the part of stakeholders, however. In conceptual terms, data providers are being asked to give up a degree of control over the data they are providing – presumably in return for some incentive or reward. They will only do so if they feel they can trust the structure or organisation that has been set up to effect this.
We consider three forms of company here: a company limited by shares, a company limited by guarantee (a CLG) and a community interest company (a CIC).
Whichever form is chosen, the company in question would operate as the data-platform owner and manager, and would enter into contractual arrangements with providers of data and proposed users.
The contractual terms would allow for:
- required investment in the company to fund infrastructure requirements such as platform development and maintenance – this could be by way of non-returnable capital contribution or loan from either the data provider or data users as circumstances merit required returns on supply of data
- required charges for use of the data
- other contractual rights and obligations specific to the circumstances including access to and usage of data.
Returns and charges could be related to commercial exploitation or fixed. Also, depending on the nature of the venture, data users may be obliged to share insights gained from access to the data with the venture so that it can be shared with other data users (e.g. see the Biobank example below). The contract terms would dictate all required obligations and liabilities between the contracting parties. Bear in mind that the structure of a data-sharing venture could be adapted over time. For example, at the outset, the stakeholders may not be in a position to finance the establishment and resourcing of a corporate entity, or it may not be seen as appropriate to a data-sharing trial. As the venture scales, however, the stakeholders may determine that a corporate structure should be implemented.
1. Choice of corporate form
One of the key questions that will determine the appropriate form of company, is whether the data-sharing venture is intended to be able to make a profit other than for the benefit of its own business – i.e. whether profits are required to be applied to the furtherance of its business,
or whether surplus profits may be dividended up to the data-sharing venture’s shareholders.
CLGs are not usually used as a vehicle for a profit-making enterprise, and a CLG’s articles of association will often (but not always) prohibit or restrict the making of distributions to members. Any profits made by a CLG will generally be applied to a not-for-profit cause such as the data-sharing venture’s purpose.
A CLG may be the most appropriate vehicle where it is not envisaged that profit or surplus generated will be distributed to its members; and it is not envisaged that the institution will seek to raise debt or equity finance. In this case activities will need to be financed by other means,
such as revenue generated from its own activities including the provision of data services or third-party funding. If the focus changes over time to encompass more commercial activities, then establishing a trading subsidiary company limited by shares could also be considered.
It should be borne in mind that a CLG (unlike a company limited by shares) does not have share capital that it is able to show on its balance sheet. This often makes it more difficult for a CLG to raise external debt finance. The alternative possibility available to companies limited
by shares, of investment by way of equity finance, is precluded here because of the structure of the CLG. Because of these difficulties, it is worth drawing attention to CICs as a further alternative corporate vehicle.
A CIC is a limited-liability company that has been formed specifically for the purpose of carrying on a business for social purposes, or to benefit a community. Although it is a profit-making enterprise, its profits are largely applied to its community purpose rather than for private gain. This is achieved by way of a cap on any movements of value from the CIC to its shareholders or members (such as by way of dividends).
This model allows shareholders to share in some of the profit, while ensuring that the CIC continues to pursue its community purpose. CICs are regulated by the Office of the Regulator of CICs (the CIC Regulator), and are required to file a community interest statement at Companies House, which is also scrutinised by the CIC Regulator. The CIC’s share
capital would appear on its balance sheet, thus increasing its ability to raise external finance.
If surpluses generated by its activities (including the provision of data services) are to be applied to its business, and its financing arrangements are secure, then a CLG will likely assist in gaining traction with those stakeholders who believe that the independence of the data trust would be compromised by virtue of its ability to pay dividends to shareholders. The structure of a company limited by guarantee provides a well-established framework of governance and liability management, and avoids the risk of exposure to a proliferation of liabilities that exists
in shareholding and trust environments.
A guarantor, which could be a non-government organisation (NGO) or other suitably established and populated body, could be appointed to monitor compliance and governance. This could address the requirement for oversight in a way that is specific to the requirements of the platform and data supplier, and to subjects not easily undertaken by other pre-established bodies, such as the Charity Commission or Regulator of Community Interest Companies, neither of which is specifically equipped to perform this function.
2. Governance and rules
The agreed purpose for the data-sharing venture will drive the overall governance of the data arrangement and its objectives, the rules for its operation and the parameters for all data-sharing agreements entered into. That purpose and those objectives should be reflected (including, where appropriate, as binding obligations) in its governance framework,
rules and the contractual framework governing the provision and use of data.
While governance and rules are not necessarily made public documents, the greater the degree of transparency as to the data venture’s operations, the greater the level of confidence that stakeholders and the wider public will be likely to feel in its functioning. Strong and transparent governance is a critical factor in establishing trust to encourage data
sharing. The rules and governance framework will underpin the purpose. Confidence that strong governance will ensure strict compliance with the rules of the trust and enforcing any failings is critical.
There needs to be confidence that the interests of all key stakeholders are represented. In a corporate model, there are a number of means of achieving this that may include board representation and/or a mix of decision-making and advisory committees representing the various interest groups. Boards and committees that are made up of trusted, respected independent members will also help engender confidence.
Depending on the circumstances and scale of the data-sharing venture, as well as an overall Governance Board, there may be an Operations Committee, a Funding Risk Advisory Committee, an Ethics Committee, a Technical Committee and a Data Committee. Alternatively, committees might be set up to represent different groups of stakeholders e.g. data providers, data users and data subjects.
With the contractual model, it would also be possible to constitute an unincorporated governance body, such as a board that comprises representatives of the stakeholders, together with some independent members who have relevant expertise. However, one can foresee potential practical difficulties with governance bodies that are more ad hoc and decentralised, including generating sufficient trust for data providers and users to submit to the jurisdiction of the body via the contractual arrangements.
3. Documentation
The documentation will need to cover the constituent parts that make up the data-sharing venture and also, if the contractual model is adopted, how these will be constituted from among the stakeholders. Participants will need to sign up to the rules of the venture, either as a stand-alone document, or by incorporation into the operational agreements, such as a data-provision agreement or data-use agreement, or the articles of a corporate vehicle. The exact contracting arrangements will be bespoke to the specific arrangement. If the venture is intended to enable additional participants to join, there will also need to be robust
arrangements (e.g. through accession agreements) to avoid re-execution of multilateral arrangements for each new joiner.
The common agreement could prescribe the arrangement in broad terms, the nature of the data that will be collected; the identity or class of the persons or organisations with whom it will be shared; and the uses to which such persons or organisations will be entitled to put that data.
It can address leaver/joiner bases,64 due diligence, terms that underpin certain values or principles, for example the five data-access ‘control dimensions’ commonly referred to as the ‘Five Safes’.65 Or, in the context of personal data, the core principles contained in Article 5 of the GDPR, change approval, the financial model for the operation of the club, dispute resolution, etc.
As mentioned above, the framework documents would need to cover the purpose of the venture and the type(s) of data in issue, along with the identity of persons or entities, or types of those that may be granted access, and the use to which they may put that data.
In addition, the documents will need to cover other important areas, such as:
- technical architecture
- interoperability
- decision-making roles
- the obligations of each participant and how any monitoring or audit of data use, particularly in respect of personal data will take place
- information security.
There will inevitably be other areas that the rules should also cover.
Key legal considerations include data protection and privacy law; regulatory obligations or restrictions; commercial confidentiality; intellectual property rights; careful consideration of liability flows (particularly important if personal data is in issue), competition and external contractual obligations. As will be seen from some of the examples (detailed in the section below), such as iSHARE, it is possible to utilise existing standard documents to cover off some of the key issues, rather than developing everything from scratch. For example, existing open-source licences could be used to protect intellectual property rights of the data providers and control data usage, bolstered by data-sharing arrangements specific to the venture.
As regards the nature of the data and its use in specific circumstances, the data providers may want to share data on a segregated and controlled basis. This means there will not be access to overall aggregated data, but there may be layered access or access to a limited number of aggregated datasets to reflect any restrictions on sharing of some data (e.g. certain data only to be shared with certain users or shared for specific insights/activities). In some instances there may be agreement to pool datasets between parties. The following requirements may be set:
- each contributor would provide raw data/datasets that include but are not limited to personal data, and that data could include normal personal data as well as special category/sensitive personal data
- no contributor would see all the raw data provided by the other contributors66
- each contributor would want to be able to analyse, and to derive data and insights from aggregate datasets, without being able to identify individuals or confidential data in the datasets
- individuals whose data is shared in this way would have the usual direct rights under data protection law in relation to the processing of their personal data.
Mapping data protection requirements onto a data-sharing venture
Where the data-sharing venture will involve processing of personal data, it will of course be necessary for all data providers, users and others processing personal data to comply with the GDPR (see in Annex 1 some of the key GDPR considerations). Depending on the nature of
the legal structure, there will be contractual terms and also potentially a Charter/Code of Conduct or Rulebook setting out the obligations of the data providers and data users including those relating to the GDPR. In some sectors, these may incorporate by referencing internationally recognised standards for data sharing, rather than completely reinventing the wheel.67
It will be necessary for each stakeholder who processes data (whether they are a data controller, joint data controller or data processor) to ensure they are compliant with GDPR requirements. This will be determined by the individual circumstances and a particular stakeholder may well be a data controller in some regards and a joint data controller
in others. Similarly, a stakeholder may be a data controller as regards some processing and a data processor in relation to others.
Privacy-enhancing technologies (PETs) are increasingly being advocated as a means to help ensure regulatory compliance and the protection of commercially confidential information more generally. For example, technologies facilitating pseudonymisation, access control and
encryption of data (in transit and at rest) and more sophisticated PETs such as differential privacy and homomorphic encryption. This is an area of development with some already mature market offerings and others still undergoing significant development.
Examples of data-sharing initiatives with elements of data stewardship
1. The Data Sharing Coalition
The Data Sharing Coalition is an international initiative started in January 2020, after the Dutch Ministry of Economic Affairs and Climate Policy invited the market to seek cooperation in pursuit of cross-sectoral data-sharing.68 It ‘builds on existing data-sharing initiatives to enable data sharing across domains. By enabling multilateral interoperability between existing and future data-sharing initiatives with data sovereignty as a core principle, parties from different sectors and domains can easily share data with each other, unlocking significant economic and societal value.’
It aims to foster collaboration between a wider range of stakeholders, providing a platform for structured exchange of knowledge in the data-sharing coalition community.69 It plans to explore and document generic data-sharing agreements which it will capture in a Trust Framework governed by the Coalition. It will support the development of existing and new data-sharing initiatives, including around technical standards, data semantics, legal agreements, and trustworthy and reusable digital identities.
Principles
The Data Sharing Coalition has six core principles:
- Be open and inclusive: any interested party is welcome to participate in the Data Sharing Coalition.
- Deliver practical results: the Data Sharing Coalition will deliver functional frameworks and facilities that provide true value for all stakeholders of the data economy and that will help them accelerate in their data sharing context.
- Promote data sovereignty: the Data Sharing Coalition aims to enable the entitled party(ies) to control their data by including this as a requirement in the use cases and frameworks.
- Leverage existing building blocks: all Data Sharing Coalition frameworks and facilities will incorporate international open standards, technology and other existing facilities where possible.
- Utilise collective governance: all frameworks and facilities produced by the Data Sharing Coalition will be governed in a transparent, consensus-driven manner by a collective of all Data Sharing Coalition participants.
- Be ethical, societal and compliant: all activities of the Data Sharing Coalition are in line with societal values and compliant with relevant legislation.
Approach
It has two initial use cases:
- green mortgages for investment in energy-saving measures
- improving risk management for shipment insurance.
Members
The Data Sharing Coalition currently has about 30 member participants including: iSHARE, IDSA, MAAS Lab, Equinix, NLAI Coalition, Amsterdam University: Connect2Trust, Dexes, ECP, Equinix, FOCWA, Fortierra, GO FAIR, HDN, International Data Spaces Association, iSHARE, KPN, Maas-Lab, MedMij, Nederlandse AI Coalitie, NEN, Netbeheer Nederland, Nexus, NOAB, Ockto, Roseman Labs, SAE ITC, SBR, SURF, Sustainable Rescue, TanQyou, Techniek Nederland, Thuiswinkel.org, Universiteit van Amsterdam, UNSense, Verbond van Verzekeraars and Visma Connect.
2. iSHARE
iSHARE is a Dutch Transport and Logistics Trust Framework for data sharing and was developed as part of the Government-backed Data Sharing Coalition.70
It is a decentralised model, where parties maintain control of what data will be shared with whom and on what conditions/for what purpose. iSHARE is not a platform but a framework. INNOPAY co-created the iSHARE framework with about 20 organisations (customs, ports, logistics, etc). It has only the list of participants and the fact that they have agreed to and demonstrated conformance with operational, technical and legal specifications; so it deals with identification, authentication and access. The idea is that an accession agreement removes the need for separate bilaterals.
It doesn’t appear to involve any data stewardship in the sense of a trusted third party being given control of what data is shared, for what purpose and with whom.
iSHARE is trying to facilitate info on or access to various agreement terms to choose from. The website has a 50-page document setting out typical agreement terms for data sharing and then links to 10–15 sets of licences, and a table for each one setting out which of those typical
terms that particular licence covers.71 The aim is to have 50 sets of terms during 2020. Currently, the licence agreements include Creative Commons, Google API Licence, Montreal, ONS, Open Banking, NIMHDA, Apache, CDLA – (copyleft Linux), Open Database Copyleft, Swedish API Open Source, Microsoft Data Use Agreement and Norwegian Open Data. Currently about 20 organisations are participants.
3. Amsterdam Data Exchange
AMDEX was initiated by the Amsterdam Economic Board and was backed by Amsterdam Science Park and Amsterdam Data Science.72 The project is supported by the City of Amsterdam.
Vision
‘The Amsterdam Data Exchange (in short: Amdex) aims to provide broad access to data for researchers, companies and private individuals. Inspired by the Open Science Cloud of the European Commission, the project is intended to connect with similar projects across Europe.
And eventually even become part of a global movement to share data more easily.’
Amdex’s CTO, Ger Baron is quoted as follows: ‘Since 2011, the municipality have had an open data policy. Municipal data is from the community and must therefore be available to everyone, unless privacy is at stake. In recent years we have learned to open up data in different
ways… We want to share data, but under the right conditions. This requires a transparent data market which is exactly what the Amsterdam Data Exchange can offer.’
The owner decides which data can be shared with whom and under what conditions. They build a ‘market model in which everyone is able to consult and use data in a transparent, familiar manner.’ 12
4. INSIGHT: The Health Data Research Hub for Eye Health
INSIGHT is a collaboration between University Hospitals Birmingham NHS Foundation Trust (lead institution), Moorfields Eye Hospital NHS Foundation Trust, the University of Birmingham, Roche, Google and Action Against AMD.
INSIGHT’s objective is to make anonymised, large-scale data, initially from Moorfields Eye Hospital and University Hospitals Birmingham, available for patient-focused research to develop new insights in disease detection, diagnosis, treatments and personalised healthcare.
Access to the datasets curated by INSIGHT is through the Health Data Research Innovation Gateway. Applications to access the data will be reviewed by INSIGHT’s Chief Data Officer and then passed to the Data Trust Advisory Board (Data TAB). The Data TAB is formed of members of the public, patients and other stakeholders joining in a private capacity.
Applications will be accepted or rejected in a transparent manner and applicants will need to sign strict licensing agreements that prioritise data security and patient benefit.
Currently the governance of INSIGHT is managed through the Advisory Board but at the recent ODI Data Institutions event, it is anticipated that a company Limited by Guarantee may be created.
5. Nallian for Cargo
Nallian is a common infrastructure for data sharing between commercial sectors.74 Nallian for Air Cargo is a set of applications built on top of Nallian’s Open Data Sharing Platform. The platform allows all stakeholders of a cargo community to connect and share relevant data across their processes, resulting in de-duplication and a single version of the truth for the benefit of airport operators, ground handlers, freight forwarders, shippers, etc. Each data source stays in control of who sees which parts of his data for which purpose. Example communities include Heathrow, Brussels and Luxembourg (e.g. Heathrow Cargo Cloud).75
6. Pistoia Alliance
The Pistoia Alliance’s mission is to lower barriers to R&D innovation by providing a legal framework to enable straightforward and secure pre-competitive collaboration.76 The Alliance is a global, not-for-profit members’ organisation conceived in 2007 and incorporated in 2009 by representatives of AstraZeneca, GSK, Novartis and Pfizer, who met at a conference in Pistoia, Italy.
The Pistoia Alliance’s projects help to overcome common obstacles to innovation and to transform R&D – whether identifying the root causes of inefficiencies, working with regulators to adopt new standards, or helping researchers implement AI effectively. There are currently more than 100 member companies – ranging from global organisations, to medium enterprises, to start-ups, to individuals – collaborating as equals on projects that generate value for the worldwide life sciences community.
7. Biobanks
Biobanks collect biological samples and associated data for medical-scientific research and diagnostic purposes and organise these in a systematic way for use by others.77 The UK Biobank is a registered charity that had initial funding of circa £62 million. Its aim is to improve the prevention, diagnosis and treatment of a wide range of serious and life-threatening illnesses such as cancer, heart disease and dementia.
UK Biobank was established by the Wellcome Trust medical charity, Medical Research Council, Department of Health, Scottish Government and the Northwest Regional Development Agency. It has also had funding from relevant charities. UK Biobank is supported by the National Health Service (NHS). Researchers apply to access its resources. The resource
is available to all bona fide researchers for all types of health-related research that is in the public interest. Researchers submit an application explaining what data they would like access to and for what purpose. The website provides summaries of funded research and academic papers.
Researchers have to pay for access to the resource on a cost-recovery basis for their proposed research, with a fixed charge for initiating the application review process and a variable charge depending on how many samples, tests and/or data are required for the research project.
- UK Biobank remains the owner of the database and samples, but will have no claim over any inventions that are developed by researchers using the resource (unless they are used to restrict health-related research or access to health-care unreasonably).
- Researchers granted access to the resource are required to publish their findings and return their results to UK Biobank so that they are available for other researchers to use for health-related research that is in the public interest.
The personal information of those joining the UK Biobank is held in strict confidence, so that identifiable information about them will not be available to anyone outside of UK Biobank. Identifying information is retained by UK Biobank to allow it to make contact with participants when required and to link with their health-related records. The level of access that is allowed to staff within UK Biobank is controlled by unique usernames and passwords, and restricted on the basis of their need to carry out particular duties.
8. Higher Education Statistics Agency
The Higher Education Statistics Agency (HESA) is the body responsible for collecting and publishing detailed statistical information about the UK’s higher education sector.78 It acts as a trusted steward of data that is made available and used by public-sector bodies including universities, public-funding bodies and the new Office for Students.
HESA was set up by agreement between funding councils, higher education providers and Government departments. It is a charitable company operating under a statutory framework and it is a recognised data source for ‘statistical information on all aspects of UK higher
education’.79 It was confirmed as a designated data body (DDB) for Higher Education in England in 2018.80
HESA collects, assures and disseminates higher education data on behalf of specific public bodies e.g. Department for Business, Energy and Industrial Strategy (DBEIS), Department for Education (DfE), Office for Students (OfS), UK Research & Innovation (UKRI) and its counterparts in the rest of the UK. As DDB, it compiles appropriate information about higher education providers and courses and makes this available to OfS, UKRI and the Secretary of State for Education. It consults as to the information it publishes with providers, students
and graduate employers. OfS holds HESA to account, reporting on its performance every three years.
HESA provides a trusted source of information, supporting better decision making, and promoting public trust in higher education. In addition, it is driven by the wider public purpose of advancing higher education in the UK.
It deploys statistical and open-data techniques to transform and present higher education data. It looks to develop low-cost techniques to improve quality and efficiency of data collection, and aims to ensure as much data as possible is open and accessible to all.
HESA may charge cost-based fees, operating on a subscription basis.
9. Safe Havens Scotland NHS Trusts for Patient Data
Safe Havens were developed in line with the Scottish Health Informatics Programme (SHIP), a blueprint that outlined a programme for a Scotland-wide research platform for the collation, management, dissemination and analysis of anonymised Electronic Patient Records(EPRs).81 The agreed principles and standards to which the Safe Havens are required to operate are set out in the Safe Haven Charter. They aim to get funding research from grants.
The Safe Havens provide a virtual environment for researchers to securely analyse data without the data leaving the environment. Their data repositories provide secure handling and linking of data from multiple sources for research projects. They also provide research support, bringing together teams around health data science. The research coordinators provide support to researchers navigating the data requirements, permissions landscape and provide a mechanism to share the lessons from one project to the next. Users are researchers who are vetted and approved. Data is never released, and personal data cannot be sold. Together, the National Safe Haven within Scottish Informatics Linkage Collaboration (SILC)82 and the four NHS Research Scotland (NRS) Safe Havens have formed a federated network of Safe Havens in order to work collaboratively to support health informatics research across Scotland.
All the Safe Havens have individual responsibility to operate at all times in full compliance with all relevant codes of practice, legislation, statutory orders and in accordance with current good professional practice. Each Safe Haven may also work independently to provide advice and assistance to researchers as well as secure environments, to enable health informatics research on the pseudonymised research datasets they create. The charter and the network facilitate collaboration between the Safe Havens by ensuring that they all work to the same principles and standards.
Problems and opportunities addressed by corporate and contractual mechanisms
Many organisations have started to explore data sharing via the use of contracts, and this model is already used in practice. The complexity of the governance model will vary depending on whether the relationships involved are one-to-one or multi-party data-sharing arrangements and whether there are singular use cases or multiple uses for the same type of purpose. Where the tools of use such as machine learning or AI become part of the agreement, further consideration is needed for defining the architecture of the legal mechanisms involved.
Multi-party and multi-use scenarios using corporate and contractual mechanisms will need to ensure an independent governance body is able to function within the structure. The role of the specific parties involved in the data ecosystem, their responsibilities, qualifications and potential competing interests will need to be considered and balanced. A difficult question emerges where the stewardship entity is absent. In this scenario, who would be the data steward that a contract could be entered into with? For example, an oversight committee composed of representatives of data users and providers could be established, but this would not be a legal entity with an ability to contract.
Other requirements that will need thoughtful consideration, as they have been mentioned throughout this chapter, are connected to the privacy and security of the data, the retention and deletion policy, and restrictions on use and onward transfers and rules of publication of
results or research.
To conclude, a series of steps need to be walked through with stakeholders to reach an agreed decision about the model to be employed. Concrete use cases are more likely to generate tangible and efficient mechanisms for the sharing of data, than vague overarching
statements of general purpose. The key element here is stakeholder engagement and the more engagement that can be encouraged at the design stage – in terms of purpose, structure and governance – the more likely it is that a data-sharing venture institution will succeed.
Case study: The Social Data Foundation
Brief overview
The Social Data Foundation83 aims to improve health and social care by accelerated access to linked data from citizens, local authorities and healthcare providers through the creation of an innovative trustworthy and scalable data-driven health and social-care ecosystem overseen by independent data stewards (i.e. the Independent Guardian).84 This new data institution takes a socio-technical approach to governing collaborative and trustworthy data linkage – and endeavours to support multi-party data sharing while respecting societal values endorsed by the community. Members of the Social Data Foundation will include the Southampton City Council, the University Hospital Southampton NHS Foundation Trust and the University of Southampton. Flexible membership is envisaged in order to allow other organisations to join and the institution to grow.
Governance
A key strength of the Social Data Foundation lies in its socio-technical approach to data governance, which necessitates a high-level of interdisciplinarity and strong stakeholder engagement from the outset (i.e. from the initial stages of design and development). This initiative therefore brings together a multi-disciplinary team of clinical and social-care practitioners with data governance, health data science, and security experts from ethics, law, technology and innovation, web science and digital health.
The Social Data Foundation builds on the data foundations governance framework85 developed by the Web Science Institute at the University of Southampton (UK) and Lapin Ltd (Jersey), which includes robust governance mechanisms together with strong citizen representation. Foundations laws are a source of inspiration for the data foundations governance framework. Two particular jurisdictions of interest are the Bailiwicks of Jersey and Guernsey (the Channel Islands) where the role of the guardian is a unique requirement, and is peculiar to these types of structures, which in a data governance model gives rise to independent data stewardship.86
Data rights
The Social Data Foundation will not only empower citizens to co-create and participate in health and social care systems transformation, but to exercise their data-related rights. As a trusted third party intermediary (TTPI) that facilitates shared data-analysis projects, the Social Data Foundation will provide a centralised hub for citizens and their datarelated requests in relation to a wide range of data (re)usage activities. Agreements will govern relationships between all stakeholders.
The Social Data Foundation will promote adequate data protection and security – and will carry out a risk assessment for each shared data analysis project before any data is shared. Data providers will only share de-identified data as part of the Social Data Foundation. Each of the parties will undertake not to seek to reverse or circumvent any such de-identification of data. Where the Social Data Foundation provides a dynamic linking service87 for authorised data users and data at rest remains within data providers’ premises, citizens are better empowered to exercise their rights over data linkage activities and oppose, restrict, or end their participation as part of the processing activities.
Case study: Emergent Alliance
Brief overview
The Emergent Alliance initiative was launched in April 2020 with the aim to aid societal recovery post COVID-19.88 Its objectives are to use data in order to accelerate global economic recovery in response to the outbreak, to make available datasets in the public domain and to develop secure data-sharing systems and infrastructure.89
The Emergent Alliance operates as a not-for-profit voluntary community made out of corporations, individuals, NGOs and government bodies that ‘contribute knowledge, expertise, data, and resources to inform decision making on regional and global economic challenges to aid societal recovery.’90
The Emergent Alliance operates as a not-for-profit voluntary community made out of corporations, individuals, NGOs and government bodies that ‘contribute knowledge, expertise, data, and resources to inform decision making on regional and global economic challenges to aid societal recovery.’90 There can be different roles in this community, such as data contributors (either members of the alliance or participants in the community) making available agreed datasets to the public domain. There can be data scientists interpreting or modelling the data with resources coming from members or crowd-sourced from partners. There could also be individuals or organisations bringing or responding to domain-based problems to the alliance, contributing with datasets, data science or technical resources.
Governance
This case study is based on information from September 2020, and the Emergent Alliance’s legal structure has progressed significantly since then. Initially, the governance structure was operating on the basis of Articles of Association, and using ‘letters of intent’ from members to govern the alliance.92 Two directors were appointed, and the structure was designed to allow different committees to be formed in order to carry out the set objectives.
Mock case study: Greenfields High School
Greenfields High School is increasingly using digital technologies to deliver
teaching materials and improve educational processes. It uses different
service providers, which are used by other schools as well. On the one hand,
Greenfields High School is interested to compare its performance with other
schools, and gain access to data and insights from its service providers. On
the other hand, Greenfields High School is interested to learn from the other
schools’ experience, and share data to understand the effectiveness of different
learning tools and methods.
Greenfields High School is not the only one in this situation. Other schools
using online tools are interested in the same goal: to get better insights
from the different service providers, to compare performances and to learn
from other schools about what tools are most effective for delivering better
educational outcomes. They all need data from the different service providers,
and from each other, to reach these goals, which ultimately serve the wider
public benefit of improving education. Greenfields High School proposes
to the other school leadership boards to convene and explore the idea of
working together. They also invite their service providers and start discussing
a data-sharing agreement that enables a trustworthy environment where each
party feels confident to share data with each other.
An independent data steward is appointed in order to ensure the proper
management of data and oversee who gets to access what type of data and
under which conditions. The data-governance framework also takes into account
the students, parents, teachers’ rights and interests. The agreement establishes
rules for:
• schools to safely and reliably exchange relevant data among themselves,
to compare their performance against that of other schools, by sharing some
types of data
• schools to share data, to understand the effectiveness of different learning
tools and methods for different educational cycles by comparing student
progress (schools keep records of educational data for all pupils for a number
of years to track progress)
• a transparent agreement about what data is collected, stored, processed
and how it is used, including rules for safeguarding students’ and parents’
rights and interests.
How would contractual mechanisms work?
Data-sharing agreements are set up with a very clear purpose in mind, and the
rules and documents could be made public to increase transparency.
An independent data steward is appointed and oversees data management. The
governance framework contains provisions around who will be permitted access
to data, for what purpose and under what circumstances. The governance
arrangements will include mechanisms for enforcing compliance and ensuring
that data users have adequate remedies if compliance fails.
The stakeholders could establish a company limited by guarantee (CLG) to fulfil
these roles with its members being participating schools – both state and private,
academies, further education bodies and data providers.
Final remarks and next steps
This report makes the first attempts to answer the question of how
legal mechanisms can help enable trustworthy data use and promote
responsible data stewardship. Trustworthy and responsible data
use are seen as key to protecting the data rights of individuals and
communities, increasing the confidence of organisational data sharing
and unlocking the benefits of data in a way that’s fair, equitable and
focused on societal benefit.
The legal mechanisms suggested in this report may offer support
for encouraging fair and trusted data sharing where individuals and
organisations retain control over the use of their data for their own
benefit, and often for wider societal good. At the same time, it is
important to highlight that responsible data stewardship should not
be equated in all circumstances with data sharing, and that responsible
data use may sometimes necessitate a decision not to share data.
Responsible data use also means robust data-governance architectures
that allow for a participatory element in taking decisions about data.
It remains to be seen whether the demand for transformation of data
practices will be driven bottom up, top down or from a mixture of both.
The mechanisms presented here may form part of the triggers that
increase the confidence of individuals to hand over the management
of their data, as well as of organisations to break data silos and
encourage beneficial uses.
As experience in the digital-platform economy demonstrates, the
commodification of data use may ultimately undermine individual or
societal interests. For this reason, it needs to be carefully considered
whether introducing financial gains for stimulating people to join
a data trust or a data cooperative would risk creating an even greater
dependency on how efficiently data is exploited, as the economic
performance of the company will translate directly into the type
of financial rewards those individuals would receive.93
Extractive data practices have proven to be successful in maximising the
economic performance of some of the big technological companies on
the market, despite these problematic business models being criticised
today. Therefore, open questions remain around the incentives models
for establishing the structures presented in this report, and to what
extent such incentives can be considered empowering and truly driving
the transformation of data practices.
Importantly, in considering these alternative mechanisms, the benefits
coming out of them as institutions – rather than a relationship between
parties – is vital. As digital technologies advance and patterns of data
use shift, the rules and principles on which civic institutions are founded
can act as a stabilising force for collective good. Further exploration
is needed as to what democratic accountability would look like for
more effective control, compared to the type of control contractual
interactions offer.
Remaining challenges
A number of challenges and difficult questions have been pointed
out throughout the report, and more issues will arise from the digital
challenges that we face today. For example, while the different
mechanisms presented here imply structures that offer considerable
flexibility, further questions remain regarding how they are able to
respond in the context of the new Internet of Things ecosystem, where
data sharing is part of everyday life, in real time.
At the same time, it can be imagined that the same type of mechanism
can be seen as the solution to distinct problems. For example, there
might be groups interested in increasing the amount of data gathered,
others interests may be around increasing the amount of data shared,
or decreasing the amount of data shared.94 If the same mechanism is used to respond to such different objectives, what are the potential tensions and how can they be addressed?
Moreover, there is the question of dealing with potential conflicts arising
between trusts, cooperatives, and corporate and contractual models.
These models will control overlapping data, therefore this could create potential tensions between structures of the same type (for example between different data trusts themselves), as well as between different structures (for example a data trust in a rivalrous relationship with
opposite interest from a data cooperative).
These models should not be seen as container-based models, and
important questions arise from interactions between the different types
of structures presented. For example, what types of interventions will
be needed in order to address potential conflicts between the different
structures? How will data rights be enforced when potentially combining
datasets across such structures?
This leads to questions around identifying ways in which more
granular mechanisms for data protection can be built in and how
to strengthen existing regulation. The structures presented here are
not meant as enclaves of protection, therefore a strong underlying
data protection layer is essential for preventing harm and achieving
responsible outcomes.
There is also an important conversation about how legal mechanisms
and other types of mechanisms such as technical ones (for example
data passports and others briefly described in Annex 1) might interact
or reinforce data stewardship.
Other difficult questions that need further research and consideration
would be:
• How will different privacy standards apply in certain situations,
for instance if the data is stored by a merchant located outside
of the UK (or the EU)?
• How can the challenges related to ensuring the independence
of different governance boards be addressed?
• What are the limitations for each legal mechanism presented? For
example, in a contractual model where a stewardship entity is absent,
who would be the data steward that a contract could be entered into
with? (An oversight committee composed of representatives of data
users and providers could be established, but this would not be a legal
entity with an ability to contract.)
•What are the implications for the transferability or mandatability
of GDPR rights in light of the Data Governance Act?
• Would a certification scheme similar to BCorps provide value for
certifying data stewardship structures?95
• Could these models be used for handling other types of assets?
On a broader scale, in the context of data sovereignty or data
nationalism, where increasing numbers of countries insist that the
personal data of their nationals be stored on servers in that jurisdiction,
the demands of data governance are likely to increase going forward. If
data contexts involve data from nationals of more than one jurisdiction,
managing data across jurisdictions would involve complex administration
requiring sufficient income to support it.
Notwithstanding the aim to facilitate trusted data sharing that results in
wider societal, economic and environmental benefit, there remains the
broader societal question of what do we want societies to do with data,
and towards which positive ambitions are we aspiring in practice?
Next steps
As observed from the list of case studies, some of the legal mechanisms
are in existence and available for immediate operation. Important lessons
can be drawn from these examples, but there remains an overarching
need for more testing, development, investment and knowledge building.
Other mechanisms such as data trusts represent a novel and unexplored
model in practice and require piloting and better understanding.
Next steps would involve practical implementation of each approach,
research and trialling and developing guidance for practitioners.
Challenges created by the global state of public health emergency from
the COVID-19 virus, as well as developments on the geopolitical side
(such as the UK leaving the European Union and new trade agreements
being discussed) and on the technological side (for example with new
data sources and new ways of data processing), trigger the need for
robust data-sharing structures where data is stewarded responsibly.
This creates an opportunity for the UK to take the lead in shaping the
emerging data-sharing ecosystem by investing in alternative approaches
to data governance. The mechanisms presented in this report offer
a starting ground for consolidating responsible and trustworthy
data management and a way towards establishing best practices
and innovative approaches that can be used as reference points
more globally.
Annexes
Annex 1
The legal mechanisms presented in this report support organisational solutions to collective action problems with data, and can be complemented by norms and rules for data stewardship and technology.
Examples of these complementarities include regulatory mechanisms, like the General Data Protection Regulation and the European Commission’s proposed Data Governance Act (which envisions data-sharing intermediaries and mechanisms for ‘data for the common good’ or data altruism).
By way of illustration, some of the key GDPR considerations that will translate into all the legal mechanisms described in this report for data providers will include:
1. ensuring that the data sharing is lawful and fair, which in addition to not being in breach of other laws, will include establishing a lawful basis under GDPR, such as:
a. the ‘legitimate interests’ basis, which requires the data provider to satisfy itself, via a three-part test and documented Legitimate
Interests Assessment, that the data-sharing is necessary to achieve legitimate interests of the data provider or a third party and that these interests are not overridden by the rights and interests of the data subjects; or
b. that the data provider has the consent of the data subjects to
share the data, which may be impractical or difficult to achieve, particularly for legacy data; to the extent that the data is ‘special category data’ (such as health data), whether one of the limited conditions for sharing such data is satisfied e.g. necessary for scientific research;
2. whether the principle of transparency has been satisfied in terms of informing data subjects of the specific disclosure of their data to, and use of their data by, the data-sharing venture;
3. whether processing of the data by the venture is incompatible with the original purposes for which the data provider collected and processed the data and thereby in breach of GDPR’s ‘purpose limitation’ principle;
4. ensuring that the shared data is limited to what is necessary
for the purposes for which the venture will process it (the ‘data minimisation’ principle);
5. ensuring that the data is accurate and where necessary kept up to date (‘accuracy’);
6. ensuring that the data will not be retained in a form that permits identification of the data subjects for any longer than necessary;
7. conducting due diligence on the data security measures established to protect data contributed to the venture;
8. ensuring that there is a mechanism in place enabling data subjects to
exercise their rights of data access, rectification, erasure, portability and right to object, including the right not to be subject to automated decision-making (‘rights’);
9. identifying any cross-border transfers of the data, or remote access to the data from outside the UK, and ensuring that such transfers or access are conducted in compliance with one of the mechanisms under GDPR; and
10. ensuring that all accountability requirements under GDPR are satisfied where appropriate, including Data Protection by Design and Default, Data Protection Impact Assessments, Appropriate Policy Document, Record of Processing Activities and mandatory
contractual requirements.96
Other complementaries could be technical mechanisms, such as Decidim, a digital platform for citizen participation97 – mechanisms that also being explored as part of the Open Data Institute programme98 – or the Alan Turing Institute’s framework on Data safe havens in the cloud,99 and the UK Anonymisation Network (UKAN) methodology for Data Situation Audits, part of the Anonymisation Decision-Making Framework.100 Together, these are the building blocks of a trustworthy institutional regime for data governance that could unlock the value of data.
There are also governance mechanisms that are starting to show what might work. For example, the participatory data governance mechanisms deployed in Genomics England101 or The Good Data102 mean that members can participate in the decision-making process and realise the potential of good data stewardship. Furthermore, work highlighted by researchers such as Salomé Viljoen and research institutes such as the Bennett Institute for Public Policy show there are also institutional mechanisms which can be used to improve the stewardship of data.103 The rules in place, the choice of collaboration and how this translates in contractual terms constitute the ‘institutional framework’ within which organisational forms. This report speaks to the possibilities of how these organisational structures and how association take place.
Other complementaries could be codes of practice or ethical codes together with social arrangements that create pressure for abiding by the rules (e.g. being thrown out of the group and denied access to the data). For example, aside from contractual terms, different legal structures might also have a rulebook or code of conduct that sets out the obligations of the data providers and data users, including those relating to GDPR. This could form a formal code of conduct under GDPR. The UK Information Commissioner’s Office (ICO) is keen to incentivise such codes. If such a code was created in compliance with the GDPR and approved by the ICO, there is the potential to create a standard form Rulebook that could be used by other similar data models. There are however certain requirements that would need to be complied with – e.g. the Code must have a clear purpose and scope. It would have to be prepared and submitted by a body representative of the categories of the data controllers and data processors involved. The Code would need to meet the particular needs of the sector or processing activities and address a clearly identified problem. It would need to facilitate the application of GDPR and be tailored to the sector – in other words add value through clear specific solutions and go beyond mere compliance with the law. Any amendments would need to be approved by the ICO. It is also important to note the ICO’s efforts in establishing regulatory sandboxes to enable companies to test new data innovations and technologies – including data-sharing projects – in a safe and controlled environment, while receiving privacy and regulatory guidance. Such regulatory sandboxes provide an interesting tool to promote data sharing for the benefit of individuals and society, while minimizing risks to people’s privacy, security and human rights
Annex 2: EU data economy regulation
Background information
Between 1960 and 1980 public concerns around automation increased around the world. In Europe, member states were facing challenges around computerisation, predominantly in public administration, and member states started adopting different data-protection rules. The first efforts to harmonise data-protection rules began and led to the adoption of the Directive 95/46/EC (Data Protection Directive) on personal data protection, which entered into force in 1995.104
The two main objectives of the Data Protection Directive were to protect fundamental rights and freedoms of individuals, and to focus on the free movement of personal information as an important component of the internal market. Therefore, the adoption of European data protection legislation is rooted in the internal market and integration efforts.
With the consolidation of individual rights in the EU in the Charter of Fundamental Rights, which entered into force in 2009, the right to personal data protection was recognised as a distinct right to the right to privacy. The right to data protection is enshrined in Article 8 of the Charter of Fundamental Rights of the European Union (the Charter) and in Article 16 of the Treaty on the Functioning of the European Union (TFUE). Thus, the EU’s competence to enact the Data Protection Directive was an internal market one.
In 2015, building on early harmonisation and integration efforts, the European Commission adopted the Digital Single Market (DSM) Strategy, which set the goal to develop a European data economy.105 This means creating a common market across member states that eliminates impediments to transnational online activity in order to foster competition, investments and innovation:
A Digital Single Market is one in which the free movement of goods, persons, services and capital is ensured and where individuals and businesses can seamlessly access and exercise online activities under conditions of fair competition, and a high level of consumer and personal data protection, irrespective of their nationality or place of residence.’
The Digital Agenda talks about better access to online goods and services, high-speed, secure and trustworthy infrastructures and investment in cloud computing and big data.12 For these purposes a number of regulatory interventions were proposed, such as consumer protection laws, the reform of the telecommunications framework, a review of the privacy and data protection in electronic communications law, and new rules for ensuring the free flow of data.
In 2018, the General Data Protection Regulation entered into force after a two-year transition period.107 The regulation updates the data-protection measures while maintaining the same two goals as the 1995 Data Protection Directive: strengthen individual rights and enable the free flow of data in the EU internal market.
Another relevant regulation adopted in 2018 was the Regulation on the Free flow of non-personal data. It aims to ensure data processing increases productivity to create new opportunities and supports the development of the data economy in the Union.108 It aims to achieve these goals by prohibiting data localisation requirements in member states (except for national security grounds) and counters vendor lock-in practices in the private sector. It also includes rules supporting data portability and interoperability as a way to ensure data mobility within the EU, increase competition and foster innovation. The Regulation intends to deal only with anonymised and aggregate data sets such as for big data analytics, farming related data, industrial production data – e.g. data on maintenance for industrial machines.
On 19 February 2020, the European Commission published the EU Data Strategy,109 along with a whitepaper on artificial intelligence110 and a communication on shaping Europe’s digital future.111 The European Commission supports a ‘human centric approach’ to technological development and the creation of ‘EU-wide common, interoperable data spaces […] overcoming legal and technical barriers to data sharing across organisations.’112
Annex 3: RadicalxChange’s Data Coalitions
This is a conceptual model that incorporates elements of all of the three legal mechanisms presented in this report
The RadicalxChange Foundation is a non-profit ‘envisioning institutions that preserve democratic values in a rapidly-changing technological landscape’,113 premised on the idea that data is essentially associated with groups, not individuals. If value comes from network effects, they ask, who owns the network? Social graphs of individuals necessarily contain information about a network of others; most records such as emails and calendar entries also refer to others; any data about one individual may be used to create a predictive profile of others. Through this account, in correcting imbalances and asymmetries, privacy is a red herring.114
To that end, RadicalxChange proposes data coalitions, which are fiduciaries for their members, but would require legislation, new regulation and an oversight board (in the US context). The problem they are meant to solve is that data subjects have less bargaining power with data consumers because the data they supply overlaps in content with that of other individuals. A data coalition would in effect bargain for all its members, aggregating and thereby increasing their influence. In this respect, they are intended to play a similar role to bottom-up data trusts.115
Governance: RadicalxChange envisages a Data Relations Board created by legislation with quasi-judicial powers to administer the area. A data coalition would legally interpose between individuals and data consumers to negotiate terms of use, privacy policies, etc. Governance
would be democratic through the membership. Decisions would have
to be binding on all members.
Data rights: To become a member, individuals would assign exclusive rights to use (some of) their data to the coalition (e.g. assigning exclusive rights to all their browsing data). The coalition would then negotiate with data consumers for the use of the data. The coalition’s rights to data would be defined contractually, and the board would ensure that the relevant data could not be collected by another entity, except through the coalition. Rights to the use of data could never be transferred permanently to a data consumer. Members could leave, and take their data with them, perhaps to an alternative coalition.
The outcome of a successful initiative would be not unlike the ambitions of the UK Government’s Smart Data Initiative.116
Sustainability of the initiative: Given the legal framework the idea requires, it would be sustainable if there was enough business to support a coalition. The proposed business model is that the coalition makes money from the data, and passes a proportion of the profits on to its
members. It is, however, on the drawing board and presumes an objective to share profits with members proportionally. The legal framework itself is unlikely to emerge in the near term.
This report was authored by Valentina Pavel.
Preferred citation: Ada Lovelace Institute. (2021). Exploring legal mechanisms for data stewardship. Available at: https://www.adalovelaceinstitute.org/report/legal-mechanisms-data-stewardship/
Image credit: Jirsak
Footnotes
- Centre for Data Ethics and Innovation (2020). Addressing trust in public sector data use. [online] GOV.UK. Available at: www.gov.uk/government/publications/cdei-publishes-its-first-report-on-public-sector-data-sharing/addressing-trust-in-public-sector-data-use [Accessed 18 Feb. 2021].
- In 2020, in partnership with Understanding Patient Data at the Wellcome Trust, the Ada Lovelace Institute convened patient roundtables and citizen juries across the UK and commissioned a nationally representative survey of 2,095 people. The findings show that 82% of people expect the NHS to publish information about data access partnerships; 63% of people are unaware that the NHS gives third parties access to data; 75% of people believe the public should be involved in decisions about how NHS data is used. The two reports that underpin this research are available at: https://understandingpatientdata.org.uk/news/accountability-transparencyand- public-participation-must-be-established-third-party-use-nhs [Accessed 18 Feb. 2021].
- For a more detailed discussion on participatory governance see the Ada Lovelace Institute’s forthcoming report on Exploring participatory mechanisms for data stewardship (March 2021).
- See ‘Annex 2: Graphical Representation’ in Manohar, S., Kapoor, A. and Ramesh, A. (2020). Data Stewardship – A Taxonomy. [online] The Data Economy Lab. Available at: https://thedataeconomylab.com/2020/06/24/data-stewardship-a-taxonomy/ [Accessed 18 Feb. 2021].
- For the purposes of this report, data trusts are regarded as underpinned by UK trust law.
- Delacroix, S. and Lawrence, N.D. (2019). Bottom-up data Trusts: disturbing the “one size fits all” approach to data governance. International Data Privacy Law, [online] 9(4). Available at: https://academic.oup.com/idpl/article/9/4/236/5579842 [Accessed 6 Nov. 2019].
- Chambers, R. (2010). Distrust: Our Fear of Trusts in the Commercial World. Current Legal Problems, [online] 63(1), pp.631–652. Available at: https://academic.oup.com/clp/article-abstract/63/1/631/379107 [Accessed 18 Feb. 2021].
- British Academy, techUK and Royal Society (2018). Data ownership, rights and controls: seminar report. [online] The British Academy. Available at: www.thebritishacademy.ac.uk/publications/data-ownership-rights-controls-seminar-report [Accessed 18 Feb. 2021].
- Delacroix, S. and Lawrence, N. D. (2019) ‘Bottom-up data Trusts.’
- Jasmontaite, L., Kamara, I., Zanfir-Fortuna, G. and Leucci, S. (2018). Data Protection by Design and by Default: Framing Guiding Principles into Legal Obligations in the GDPR [online] European Data Protection Law Review, 4(2), pp.168–189. Available at: https://doi.org/10.21552/edpl/2018/2/7. [Accessed 18 Feb. 2021].
- Delacroix, S. and Lawrence, N. D. (2019) ‘Bottom-up data Trusts’.
- Ibid.
- McFarlane, B. (2019). Data Trusts and Defining Property. [online] Oxford Law Faculty. Available at: www.law.ox.ac.uk/research-andsubject- groups/property-law/blog/2019/10/data-trusts-and-defining-property [Accessed 18 Feb. 2021].
- Prof. McFarlane puts forward this potential workaround in a conversation with Paul Nemitz and Sylvie Delacroix. See Data Trusts Initiative (2021) Understanding the Data Governance Act: in conversation with Sylvie Delacroix, Ben McFarlane and Paul Nemitz.
- For further discussion of this and other issues in the development of data trusts, see: Data Trusts Initiative (2020b). Data Trusts: from theory to practice, working paper 1 [online] Data Trusts Initiative. Available at: https://static1.squarespace.com/ static/5e3b09f0b754a35dcb4111ce/t/5fdb21f9537b3a6ff2315429/1608196603713/Working+Paper+1+-+data+trusts+- +from+theory+to+practice.pdf [Accessed 18 Feb. 2021].
- Wachter, S. and Mittelstadt, B. (2018). A Right to Reasonable Inferences: Re-Thinking Data Protection Law in the Age of Big Data and AI. [online] papers.ssrn.com. Available at: https://papers.ssrn.com/abstract=3248829 [Accessed 18 Feb. 2021].
- A broader discussion could be around whether drawing boundaries is the right approach or whether we might need a different regime for inferences.
- For a more detailed discussion on caveats and shortcomings see O’hara, K. (2020) ‘Data Trusts’. For further discussion regarding the development of data trusts see: Data Trusts Initiative (2020) Data Trusts: from theory to practice, working paper 1.
- See Co-operatives UK (n.d.). Understanding co‑ops. [online] uk.coop. Available at: www.uk.coop/understanding-co-ops [Accessed 18 Feb. 2021].
- The ICA is the global federation of co-operative enterprises. More information available at International Cooperative Alliance (2019). Home. [online] ica.coop. Available at: www.ica.coop/en [Accessed 18 Feb. 2021].
- International Cooperative Alliance (2019a). Facts and figures. [online] ica.coop. Available at: www.ica.coop/en/cooperatives/factsand- figures [Accessed 18 Feb. 2021].
- More information available at: Consumer Federation of America (n.d.). Consumer Cooperatives. [online] Consumer Federation of America. Available at: https://consumerfed.org/consumer-cooperatives [Accessed 18 Feb. 2021].
- More information available at: Find Your Credit Union (n.d.). About Credit Unions. [online] Find Your Credit Union. Available at: www.findyourcreditunion.co.uk/about-credit-unions [Accessed 18 Feb. 2021].
- Morning AgClips (2021). A snapshot of the top 100 agricultural cooperatives. [online] morningagclips.com. Available at: www.morningagclips.com/a-snapshot-of-the-top-100-agricultural-cooperatives [Accessed 18 Feb. 2021].
- More information available at: www.ica.coop/en/cooperatives/cooperative-identity and International Cooperative Alliance (2017) The Guidance Notes on the Cooperative Principles. Available at: www.ica.coop/en/media/library/research-and-reviews/guidancenotes- cooperative-principles [Accessed 18 Feb. 2021].
- For example, there have been a number of experiments in using cooperative forms to manage data equitably, especially in the area of healthcare. See Blasimme, A., Vayena, E. and Hafen, E. (2018). Democratizing Health Research Through Data Cooperatives. Philosophy & Technology, [online] 31(3), pp.473–479. Available at: https://doi.org/10.1007/s13347-018-0320-8 [Accessed 18 Feb. 2021]; Hafen, E. (2019). Personal Data Cooperatives – A New Data Governance Framework for Data Donations and Precision Health. Philosophical Studies Series, pp.141–149. Available at: https://doi.org/10.1007/978-3-030-04363-6_9 [Accessed 18 Feb. 2021].
- See International Cooperative Alliance, Facts and figures and Cooperatives UK (2017). Simply Legal. [online] Available at: www.uk.coop/sites/default/files/2020-10/simply-legal-final-september-2017.pdf [Accessed 18 Feb. 2021].
- Co-operatives UK is a network for thousands of co-operative businesses with a mission to grow the co-operative economy. More information available at: www.uk.coop/about [Accessed 18 Feb. 2021].
- See Co-operatives UK (2021), Understanding co‑ops. [online]. Available at: www.uk.coop/about/what-co-operative [Accessed 18 Feb. 2021].
- Co-operatives UK (2017) Simply Legal.
- See: Co-operative and Community Benefit Societies Act 2014. [online] Available at: www.legislation.gov.uk/ukpga/2014/14/contents [Accessed 18 Feb. 2021].
- See Financial Conduct Authority (2015) Guidance on the FCA’s registration function under the Co-operative and Community Benefit Societies Act 2014, Finalised guidance 15/12 [online]. Available at: www.fca.org.uk/publication/finalised-guidance/fg15-12.pdf
- Co-operatives UK (2017) Simply Legal.
- See Co-operatives UK (2018), Support for your co‑op. [online]. Available at: www.uk.coop/developing-co-ops/select-structure-tool [Accessed 18 Feb. 2021].
- Depending on the type of cooperative, members of a cooperative can also be SMEs, enterprises, different types of individuals or groups or a combination of these. For more information see Co-operatives UK (2018), Types of Co-ops. [online]. Available at: www.uk.coop/understanding-co-ops/what-co-op/types-co-ops [Accessed 18 Feb. 2021].
- Shadbolt, N., O’Hara, K., De Roure, D. and Hall, W. (2019). The Theory and Practice of Social Machines. Lecture Notes in Social Networks. Cham: Springer International Publishing. Available at: https://www.springer.com/gp/book/9783030108885
- For a richer discussion on governing the commons see Ostrom, E. (2015). Governing the Commons. Cambridge: Cambridge University Press.
- Ostrom, E. (2015) Governing the Commons. Available at: https://doi.org/10.1017/CBO9781316423936
- Grossman, R. (2018). A Proposed End-To-End Principle for Data Commons. [online] Medium. Available at: https://medium.com/ @rgrossman1/a-proposed-end-to-end-principle-for-data-commons-5872f2fa8a47 [Accessed 18 Feb. 2021].
- See Ada Lovelace Institute (2020). Exploring principles for data stewardship. [online] www.adalovelaceinstitute.org. Available at: www.adalovelaceinstitute.org/project/exploring-principles-for-data-stewardship [Accessed 18 Feb. 2021] and Ostrom, E. (2015) Governing the Commons.
- See Salus Coop (n.d.). Home. [online] SalusCoop. Available at: www.saluscoop.org [Accessed 18 Feb. 2021].
- More information available at: Salus Coop (2020). TRIEM: Let’s choose a better future for our data. [online] SalusCoop. Available at: www.saluscoop.org/proyectos/triem [Accessed 18 Feb. 2021].
- The terms of the licences are available at Salus Coop (2020). Licencia. [online]. Available at: www.saluscoop.org/licencia [Accessed 18 Feb. 2021].
- More information available at: Salus Coop (2020). Co3. [online]. Available at: www.saluscoop.org/proyectos/co3 [Accessed 18 Feb. 2021].
- See Driver’s Seat Cooperative (n.d). Home. [online]. Available at: www.driversseat.co [Accessed 18 Feb. 2021].
- See OpenCorporates (2021). Salus Coop. [online] opencorporates.com. Available at: https://opencorporates.com/companies/us_ co/20191545590 [Accessed 18 Feb. 2021].
- See Driver’s Seat Cooperative (2020). Privacy notice [online]. Available at: www.driversseat.co/privacy [Accessed 18 Feb. 2021].
- See Start.Coop (2019), Cohort report 2019. [online] Available at: https://start.coop/wp-content/uploads/2019/12/Start. coop_2019Report.pdf [Accessed 18 Feb. 2021].
- See PitchBook (n.d.), Driver’s Seat Cooperative Company Profile: Valuation & Investors. [online] Available at: https://pitchbook.com/ profiles/company/251012-17 [Accessed 18 Feb. 2021].
- More information available at: TheGoodData (n.d). Home. [online]. Available at: www.thegooddata.org [Accessed 18 Feb. 2021].
- For more information see: Nesta (n.d.). The Good Data. [online] Nesta. Available at: www.nesta.org.uk/feature/me-my-data-and-i/thegood- data/ [Accessed 18 Feb. 2021].
- See Partial Amendment to Rules dated 18 July 2017, filed at the FCA: https://mutuals.fca.org.uk/Search/Society/26166 [Accessed 18 Feb. 2021].
- For more information see Nesta (n.d.). The Good Data.
- See Annual Return and Accounts dated 31 December 2018 filed at the FCA: https://mutuals.fca.org.uk/Search/Society/26166 [Accessed 18 Feb. 2021].
- See Request to Cancel dated 6 September 2019 filed at the FCA: https://mutuals.fca.org.uk/Search/Society/26166 [Accessed 18 Feb. 2021].
- Konings, R. (2019). Join a data union with the Swash browser plugin. [online] Medium. Available at: https://medium.com/streamrblog/ join-a-data-union-with-the-surf-streamr-browser-plugin-d9050d2d9332 [Accessed 18 Feb. 2021].
- Royal Society (2019). Protecting privacy in practice: The current use, development and limits of Privacy Enhancing Technologies in data analysis. [online] Royal Society. Available at: https://royalsociety.org/-/media/policy/projects/privacy-enhancing-technologies/ privacy-enhancing-technologies-report.pdf?la=en-GB&hash=862C5DE7C8421CD36C105CAE8F812BD0 [Accessed 18 Feb. 2021].
- For a more detailed discussion see: UK Anonymisation Network (2020). Anonymisation Decision-Making Framework. [online] Available at: https://ukanon.net/framework [Accessed 18 Feb. 2021].
- In RadicalxChange’s view, data fails because most of the information we have at our disposal (about ourselves and others) is largely the same as information others have at their disposal. The price is dragged down to zero as buyers can always find a cheaper seller for the same data. However, data’s combined value, which is higher than zero, is almost entirely captured by the (well-capitalised) parties that have capacity to combine data and extract insights. Because of this market failure, which is peculiar to data, RadicalxChange believes that top-down intervention is needed to make bottom-up organisation possible through Data Coalitions. Through the right type of legislation, the problem of buy-in for joining data coalitions would be removed, because joining would be costless or virtually costless and immediately advantageous or remunerative. RadicalxChange is discussed as a conceptual model in Annex 3.
- Cecco, L. (2020). Members of Canada’s largest retail co-op seek to block sale to US private equity fund. [online] The Guardian. Available at: www.theguardian.com/world/2020/sep/22/canada-mountain-equipment-co-op-members-bid-block-sale-us-firm [Accessed 18 Feb. 2021].
- Directive 2002/58/EC of the European Parliament and of the Council of 12 July 2002 concerning the processing of personal data and the protection of privacy in the electronic communications sector (Directive on privacy and electronic communications). Available at: https://eur-lex.europa.eu/legal-content/EN/ALL/?uri=CELEX%3A32002L0058 [Accessed 18 Feb. 2021].
- Directive 2015/2366 of the European Parliament and of the Council of 25 November 2015 on payment services in the internal market. Available at: https://eur-lex.europa.eu/legal-content/EN/TXT/?uri=CELEX:32015L2366 [Accessed 18 Feb. 2021].
- Article 11 and Recital 25 of the draft Data Governance Act include requirements for data-sharing services to be placed in a separate legal entity. This is required both in business-to-business data sharing as well as in business-to-consumer contexts where separation between data provision, intermediation and use needs to be provided. The text does not distinguish between closed or open groups.
- In order to improve the chances of participation, and where technically feasible, the exit arrangements for leavers should focus on the ability of a participant to leave the venture and remove their data. This respects the data sovereignty of the participant and enables them to remain in control of data, particularly important for personal data as participants will be conscious of their obligations under GDPR.
- The ‘Five Safes’ comprise: safe projects, safe people, safe data, safe settings and safe outputs. Ritchie, F. (2017). The “Five Safes”: a framework for planning, designing and evaluating data access solutions. [online] Zenodo. Available at: https://zenodo.org/ record/897821 [Accessed 18 Feb. 2021].
- As part of the stewardship model, one of the protections should be only the data needed for an activity is accessed by other participants/stakeholders.
- An example is the Rules of Participation used by Health Data Research UK (HDR UK). Organisations requesting data access from one of the hubs set up through HDR UK (including the INSIGHT hub) are required to commit to these rules, which reference published standards. See Health Data Research UK (2020). Digital Innovation Hub Programme Prospectus Appendix: Principles For Participation. [online]. Available at: www.hdruk.ac.uk/wp-content/uploads/2019/07/Digital-Innovation-Hub- Programme-Prospectus-Appendix-Principles-for-Participation.pdf [Accessed 18 Feb. 2021].
- See The Data Sharing Coalition (n.d.) Home. [online]. Available at: https://datasharingcoalition.eu.
- The Data Sharing Coalition published an exploration on standards and agreements for enabling data sharing. See Data Sharing Coalition (2021). Harmonisation Canvas [online]. Available at: https://datasharingcoalition.eu/app/uploads/2021/02/210205- harmonisation-canvas-v05-1.pdf
- See Support Centre for Data Sharing (2020). iSHARE: Sharing Dutch transport and logistics data. [online] Support Centre for Data Sharing. Available at: https://eudatasharing.eu/examples/ishare-sharing-dutch-transport-and-logistics-data [Accessed 18 Feb. 2021].
- Support Centre for Data Sharing (2019). Report on collected model contract terms. [online]. Available at: https://eudatasharing.eu/ sites/default/files/2019-10/EN_Report%20on%20Model%20Contract%20Terms.pdf [Accessed 18 Feb. 2021].
- For more information see Amsterdam Smart City (2020). Amsterdam Data Exchange [online]. Available at: https://amsterdamsmartcity.com/updates/project/amsterdam-data-exchange-amdex [Accessed 18 Feb. 2021].
- Ibid.
- For more information see Nallan (2020). Home. [online] Available at: www.nallian.com [Accessed 18 Feb. 2021].
- For more information see Heathrow (2020). Cargo. [online] Available at: www.heathrow.com/company/cargo [Accessed 18 Feb. 2021].
- For more information see Pistoia Alliance (2020). About. [online]. Available at: www.pistoiaalliance.org/membership/about [Accessed 18 Feb. 2021].
- For more information see UK Biobank (2020). Home. [online]. Available at: www.ukbiobank.ac.uk [Accessed 18 Feb. 2021].
- For more information see HESA (2020). About. [online] Available at: www.hesa.ac.uk/about
- HESA (2017). HE representatives comment on consultation on designated data body [online] hesa.ac.uk. Available at: www.hesa.ac.uk/news/19-10-2017/consultation-designated-data-body [Accessed 18 Feb. 2021].
- See HESA (2020). Designated Data Body. [online]. Available at: www.hesa.ac.uk/about/what-we-do/designated-data-body [Accessed 18 Feb. 2021].
- Scottish Government (2015). Charter for Safe Havens in Scotland: Handling Unconsented Data from National Health Service Patient Records to Support Research and Statistics. [online] www.gov.scot. Available at: www.gov.scot/publications/charter-safe-havensscotland- handling-unconsented-data-national-health-service-patient-records-support-research-statistics/pages/3 [Accessed 18 Feb. 2021].
- For more information see Data Linkage Scotland (2020). Home. [online ] Available at: www.datalinkagescotland.co.uk [Accessed 18 Feb. 2021].
- Boniface, M., Carmichael, L., Hall, W., Pickering, B., Stalla-Bourdillon, S. and Taylor, S. (2020). A Blueprint for a Social Data Foundation: Accelerating Trustworthy and Collaborative Data Sharing for Health and Social Care Transformation. [online] Available at: https://southampton.ac.uk/~assets/doc/wsi/WSI%20white%20paper%204%20social%20data%20foundations.pdf [Accessed 18 Feb. 2021]
- The Independent Guardian is defined as follows: ‘A team of experts in data governance, who are independent from the Social Data Foundation Board and oversee the administration of the Social Data Foundation to ensure it achieves its purposes in accordance with its rulebook i.e. that all data related activities realise the highest standards of excellence for data governance. In particular, the Independent Guardian shall (i) help set up a risk-based framework for data sharing, (ii) assess the use cases in accordance with this risk-based framework and (iii) audit and monitor day-to-day all data-related activities, including data access, citizen participation and engagement.’ See Boniface, M. et al. (2020) A Blueprint for a Social Data Foundation.
- Stalla-Bourdillon, S., Wintour, A. and Carmichael, L. (2019). Building Trust Through Data Foundations: A Call for a Data Governance Model to Support Trustworthy Data Sharing. [online] Available at: https://cdn.southampton.ac.uk/assets/imported/transforms/ content-block/UsefulDownloads_Download/69C60B6AAC8C4404BB179EAFB71942C0/White%20Paper%202.pdf [Accessed 18 Feb. 2021]. The Social Data Foundation is an example of a functional data foundation – for more information see: StallaBourdillon, S., Carmichael, L., & Wintour, A. (Forthcoming). Fostering trustworthy data sharing: Establishing data foundations in practice. Data & Policy; Stalla-Bourdillon, S., Carmichael, L., & Wintour, A. (2020, September). Fostering Trustworthy Data Sharing: Establishing Data Foundations in Practice. Data for Policy Conference 2020, Available at: http://doi.org/10.5281/zenodo.3967690. [Accessed 18 Feb. 2021].
- Note that all foundations incorporated under Jersey foundations law must have a guardian.
- Dynamic linking service is understood as where two or more sources of health and social care data are brought together on demand according to the specific parameters of an authorised data user’s query where the risk of re-identification is both evaluated before and after data linkage, and mitigated through assurance processes facilitated by the Data Foundation.
- For more information see Emergent Alliance (n.d). Home. [online]. Available at: https://emergentalliance.org [Accessed 18 Feb. 2021]
- See Emergent Alliance (2020). Articles of Incorporation, p. 16. Available at: https://find-and-update.company-information.service.gov. uk/company/12562913/filing-history [Accessed 18 Feb. 2021].
- See Emergent Alliance (n.d.). Frequently Asked Questions. Available at: https://emergentalliance.org/?page_id=440 [Accessed 18 Feb. 2021]
- See Emergent Alliance (n.d.). Frequently Asked Questions. Available at: https://emergentalliance.org/?page_id=440 [Accessed 18 Feb. 2021]
- See Emergent Alliance (n.d), Statement of Intent. Available at: https://emergentalliance.org/?page_id=452 [Accessed 18 Feb. 2021].
- For a more detailed description of this failure model and others see Porcaro, K. (2020). Failure Modes for Data Stewardship. [online] Mozilla Insights. Available at: https://drive.google.com/file/d/1twxDGIBYz0TyM3yHDgA8qyf16Ltkk4V7/view [Accessed 18 Feb. 2021].
- See O’hara, K. (2020). Data Trusts
- BCorps are companies balancing profit gains with societal outcomes which receive a certification based on social and environmental performance, public transparency, and accountability. For more information see B Corporation (n.d.) About B Corps. [online]. Available at: https://bcorporation.net/about-b-corps [Accessed 18 Feb. 2021].
- The Information Commissioner’s Office (ICO) published a draft Data Sharing Code of Practice that covers many of the above requirements, including expectations in terms of data sharing agreements. See Information Commissioner’s Office (2020). ICO publishes new Data Sharing Code of Practice (online). Available at: https://ico.org.uk/about-the-ico/news-and-events/news-andblogs/2020/12/ico-publishes-new-data-sharing-code-of-practice
- For more information see https://decidim.org
- See Thereaux, O. and Hill, T. (2020). Understanding the common technical infrastructure of shared and open data. [online] theodi.org. Available at: https://theodi.org/article/understanding-the-common-technical-infrastructure-of-shared-and-open-data [Accessed 18 Feb. 2021]
-
Alan Turing Institute (n.d.). Data safe havens in the cloud. [online] The Alan Turing Institute. Available at: www.turing.ac.uk/research/
research-projects/data-safe-havens-cloud [Accessed 18 Feb. 2021]. - See UK Anonymisation Network (UKAN), Anonymisation Decision-Making Framework.
- For more information see: Genomics England (n.d.) Home. [online]. Available at: www.genomicsengland.co.uk
- For more information see: TheGoodData (2020). Home. Available at: www.thegooddata.org
- Viljoen, S. (2020). Democratic Data: A Relational Theory For Data Governance. [online] Available at: https://doi.org/10.2139/ ssrn.3727562 [Accessed 18 Feb. 2021]; Coyle, D. et al. (2020) Valuing data.
- Directive 95/46/EC of the European Parliament and of the Council of 24 October 1995 on the protection of individuals with regard to the processing of personal data and on the free movement of such data. Available at https://eur-lex.europa.eu/legal-content/EN/ TXT/?uri=celex%3A31995L0046
- European Commission (2015). A Digital Single Market Strategy for Europe. [online]. Available at: https://eur-lex.europa.eu/legalcontent/EN/TXT/?uri=COM%3A2015%3A192%3AFIN
- Ibid.
- Regulation 2016/679 of the European Parliament and of the Council of 27 April 2016 on the protection of natural persons with regard to the processing of personal data and on the free movement of such data (General Data Protection Regulation). Available at: https://eur-lex.europa.eu/legal-content/EN/TXT/?uri=CELEX%3A32016R0679
- Recital 2 of the Regulation 2018/1807 on a framework for the free flow of non-personal data in the European Union. Available at: https://eur-lex.europa.eu/legal-content/EN/TXT/?uri=CELEX:32018R1807
- See European Commission (2020). A European strategy for data.
- European Commission (2020c). On Artificial Intelligence – A European approach to excellence and trust. [online] Available at: https://ec.europa.eu/info/sites/info/files/commission-white-paper-artificial-intelligence-feb2020_en.pdf [Accessed 18 Feb. 2021]
- European Commission (2020e). Shaping Europe’s Digital Future. [online]. Available at: https://ec.europa.eu/info/sites/info/files/ communication-shaping-europes-digital-future-feb2020_en_4.pdf [Accessed 18 Feb. 2021].
- European Commission (2020). A European strategy for data.
-
Posner, E. A. and Weyl, E. G. (2018) Radical markets. Uprooting Capitalism and Democracy for a Just Society.
Princeton University Press. Available at: https://doi.org/10.2307/j.ctvc77c4f - See RadicalxChange Foundation’s Data Freedom Act. Available at: www.radicalxchange.org/kiosk/papers/data-freedom-act.pdf [Accessed 18 Feb. 2021].
- Delacroix, S. and Lawrence, N. D. (2019). ‘Bottom-up data Trusts’
-
UK Department for Business, Energy and Industrial Strategy (2019). Smart Data: Putting consumers in control of their data and
enabling innovation. [online] Gov.uk Available at: https://assets.publishing.service.gov.uk/government/uploads/system/uploads/
attachment_data/file/808272/Smart-Data-Consultation.pdf [Accessed 18 Feb. 2021].
Related content
Disambiguating data stewardship
Why what we mean by ‘stewarding data’ matters
Exploring legal mechanisms for data stewardship
Launch of a joint publication with the AI Council exploring legal mechanisms that could help facilitate responsible data stewardship
Removing the pump handle – stewarding data at times of public health emergency
By examining our past, we can find lessons for our future - avoiding pitfalls and ensuring equitable outcomes.
The foundations of fairness for NHS health data sharing
How do the public expect the NHS, and third-party organisations to steward their data?