What is a foundation model?
This explainer is for anyone who wants to learn more about foundation models, also known as 'general-purpose artificial intelligence' or 'GPAI'.
17 July 2023
Reading time: 37 minutes
Artificial intelligence (AI) technologies have a significant impact on our day-to-day lives. AI is embedded in many systems and processes that affect us.
People have mixed views about the use of AI technologies in our lives,[1] recognising both the benefits and the risks. While many members of the public believe these technologies can make aspects of their lives cheaper, faster and more efficient, they also express worries that they might replace human judgement or harm certain members of society.
An emerging type of AI system is a ‘foundation model’, sometimes called a ‘general-purpose AI’ or ‘GPAI’ system. These are capable of a range of general tasks (such as text synthesis, image manipulation and audio generation). Notable examples are OpenAI’s GPT-3 and GPT-4, foundation models that underpin the conversational chat agent ChatGPT.
Because foundation models can be built ‘on top of’ to develop different applications for many purposes, this makes them difficult – but important – to regulate. When foundation models act as a base for a range of applications, any errors or issues at the foundation-model level may impact any applications built on top of (or ‘fine-tuned’) from that foundation model.
As these technologies are capable of a wide range of general tasks, they differ from narrow AI systems (those that focus on a specific or limited task, for example, predictive text or image recognition) in two important respects: it can be harder to identify and foresee the ways they can benefit people and society, and it is also harder to predict when they can cause harm.
As policymakers begin to regulate AI, it will become increasingly necessary to distinguish clearly between types of models and their capabilities, and to recognise the unique features of foundation models that may require additional regulatory attention.
For these reasons, it is important for the public, policymakers, industry and the media to have a shared understanding of terminology, to enable effective communication and decision-making.
We have developed this explainer to cut through some of the confusion around these terms and support shared understanding. This explainer is for anyone who wants to learn more about foundation models, and it will be particularly useful for people working in technology policy and regulation.
In this explainer we use the term ‘foundation models’ – which are also known as ‘general-purpose AI’ or ‘GPAI’. Definitions of foundation models and GPAI are similar and sometimes overlap. We have chosen to use ‘foundation models’ as the core term to describe these technologies. We use the term ‘GPAI’ in quoted material, and where it’s necessary in the context of a particular explanation.
We also explain other related terminology and concepts, to help distinguish what is and isn’t a foundation model.
We recognise that the terminology in this area is contested. This is a fast-moving topic, and we expect that language will evolve quickly. This explainer should therefore be viewed as a snapshot in time.
Rather than claiming to have solved the terminology issue, this explainer will help those working in this area to understand current norms in uses of terminologies, and their social and political contexts.
Terminology is socially constructed and needs to be understood in context – where possible we have included the origins and uses of terms, to help explain the motivations behind their use.
Why are foundation models hard to define?
It is hard to define and explain these technologies, partly because people don’t always agree on the meaning of AI itself. There is no single definition of AI, and the term is applied in a wide variety of settings. Even what is meant by ‘intelligence’ is a contested concept.[2]
In this explainer, we refer to the UK Data Ethics Framework’s definition of AI: ‘AI can be defined as the use of digital technology to create systems capable of performing tasks commonly thought to require intelligence.’[3] For a full definition, see Table 3: Glossary.
We recognise that this definition is not definitive and is UK-centric, but also that it is similar to other definitions adopted by national and international governments – including the EU High Level Expert Group on AI’s definition of AI as ‘systems that display intelligent behaviour by analysing their environment and taking actions – with some degree of autonomy – to achieve specific goals.’ [4]
There are several similar, related terms used in policymaking, academia, industry and in the media (see Table 3: Glossary). Many of these terms stem from computer science but are now being used in different ways by other sectors. Not everyone agrees on the meaning of these terms, particularly as their use evolves over time.
The terms can be difficult to define, subject to multiple interpretations and are often poorly understood. Some of these terms refer to components of AI systems, or related or subdisciplines of AI. We hope the definitions we have provided here provide a base level of shared understanding for members of the public, policymakers, industry and the media.
Foundation model supply chain diagram
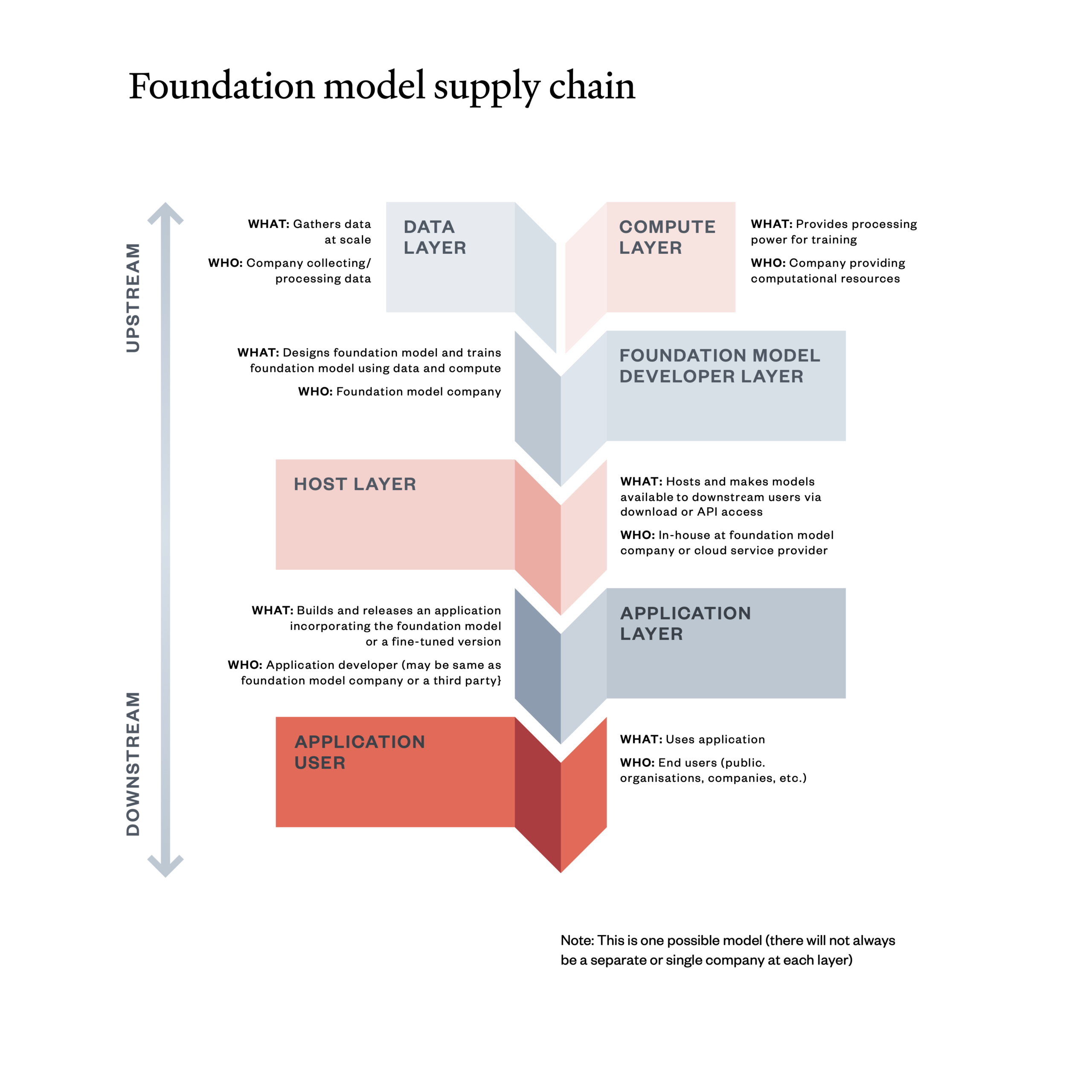
AI technologies and foundation models
What is a foundation model?
Foundation models are AI models designed to produce a wide and general variety of outputs. They are capable of a range of possible tasks and applications, such as text, image or audio generation. They can be standalone systems or can be used as a ‘base’ for many other applications.[5]
Researchers have suggested the ‘general’ definition refers to foundation models’ scope of ability, range of uses, breadth of tasks or types of output.[6]
Some foundation models are capable of taking inputs in a single ‘modality’ – such as text – while others are ‘multimodal’ and are capable taking multiple modalities of input at once, for example, text, image, video, etc., and then generating multiple types of output, (such as generating images, summarising text, answering questions) based on those inputs.
The term ‘foundation model’ was popularised in 2021 by researchers at the Stanford Institute for Human-Centered Artificial Intelligence, in collaboration with the Stanford Center for Research on Foundation Models, an interdisciplinary initiative set up by the Stanford Institute for Human-Centered AI . These researchers defined foundation models as ‘models trained on broad data (generally using self-supervision at scale) that can be adapted to a wide range of downstream tasks.’[7]
Foundation models form the basis of many applications including OpenAI’s ChatGPT, Microsoft’s Bing, and many website chatbots. They similarly underpin many image generation tools such as Midjourney or Adobe Photoshop’s generative fill tools.
For example, the popular application ChatGPT is built on the GPT-3.5 and GPT-4 families of foundation models. The GPT-3.5 and GPT-4 families of models are also the base for many other applications, such as Bing Chat and Duolingo Max.[8]
A defining characteristic of foundation models is the scale of data and computational resources involved in building them. They require datasets featuring billions of words or hundreds of millions of images scraped from the internet.[9] Foundation models also rely on ‘transfer learning’ – that is, applying learned patterns from one task to another.[10]
Current foundation models’ capabilities include but are not limited to the ability to: translate and summarise text; generate reports from a series of notes; draft emails; respond to queries and questions; and create new text, images, audio or visual content based on text or voice prompts.
A foundation model can be accessed by other companies (downstream in the supply chain) that can build AI applications ‘on top’ of a foundation model, using a local copy of a foundation model or an application programming interface (API). In this context, ‘downstream’ refers to activities post-launch of the foundation model and activities that build on a foundation model.
For example, following the launch of OpenAI’s foundation model GPT-4, OpenAI allowed companies to build products underpinned by GPT-4 models. These include Microsoft’s Bing Chat[11], Virtual Volunteer by Be My Eyes (a digital assistant for people who are blind or have low vision), and educational apps such as Duolingo Max,[12] Khan Academy’s Khanmigo[13] [14]. See Table 1 for application descriptions.
Additionally, the foundation model provider will often allow downstream companies to create a customised version of the foundation model using a process called ‘fine-tuning’ – which describes when new data is added to a foundation model to ‘fine-tune’ its performance and capabilities on specific tasks.
Foundation models vs narrow AI
Foundation models (as defined above) are different to other artificial intelligence (AI) models, which may be designed for a specific or ‘narrow’ task. A ‘narrow’ AI system is designed to be used for a specific purpose and is not designed to be used beyond its original purpose.
Narrow AI applications are trained on specific data for a specific task and context. They are not designed for reuse in new contexts. For example, a bank’s model for predicting the risk of default by a loan applicant would not also be capable of serving as a chatbot to communicate with customers.
It’s worth noting that both narrow AI models and foundation models can be either unimodal (receiving input based on just one content input type, and generating only text like BERT, or only images like DALL·E), or multimodal (capable receiving input and generating content or tasks in a range of modes, like text-to-text image captioning, and robotic arm manipulation like PaLM-E).
Foundation models are also known as…
As well as ‘foundation model’ and ‘GPAI’, there are other related terms used to describe similar models. These include ‘generative AI’ and ‘large language models (LLMs)’. These are discussed in the ‘Types of foundation model’ section.
Some other terms, such as ‘frontier models’ and ‘AGI/strong AI’ are also being used in industry, policy and elsewhere, but are more contested. This is in part because of the lack of a specific interpretation, and in part because of their origins and the context in which they are used. We discuss these terms in more detail below.
Foundation models: applications
Foundation models underpin a range of applications. These applications use foundation models with ‘fine-tuning’ to create applications.
Table 1: Examples of foundation model applications
Foundation model and company | Application name and company | Application function |
Action Transformer (ACT-1) by Adept | Make use of digital tools, for example, to observe what’s happening in a web browser and take certain actions, like clicking, typing and scrolling[15] | |
BERT by Google | BioBERT by DMIS Laboratory, Korea University | Answer questions, recognise entities, extracting semantic relations from text etc., using biomedical text mining[16] |
Claude by Anthropic | Claude by Anthropic | Provide a wide variety of conversational and text-processing tasks |
DALL·E by OpenAI[17] | Create realistic images and art from natural language descriptions | |
DALL·E 2 by OpenAI[18] | Create realistic images and art from natural language descriptions | |
Gato by Google DeepMind | Play games, caption images, chat, control a robot arm | |
GPT-4 by OpenAI
|
Bing Chat by Microsoft | Answer complex questions, summarise information[19] |
Duolingo Max by Duolingo | Provide AI roleplay and ‘explain my answer’ features as part of a modern-languages learning application | |
Virtual Volunteer by Be My Eyes | Provide virtual assistant services for people who are blind or have low vision | |
ChatGPT by Open AI | Provide answers and advice, summarise notes, generate written content | |
Khanmigo by Khan Academy
|
Generate lesson plans, provide tutor-like responses and mimic coaching for students | |
BLOOM by Huggingface | Generate text in 46 natural languages and 13 programming languages | |
PaLM 2[20] | Bard by Google | Provide an AI chat service described as experimental and conversational[21] |
Midjourney 5.1 by Midjourney | Midjourney Bot for Discord by Midjourney | Generate lifelike images |
PaLM-E | Transfer knowledge from varied visual and language domains to a robotics system | |
Proximus | YODA | Generate immediate answers to customer questions |
Spark by Meta | Instagram filters by Instagram | Augment images in a particular style
|
Stable Diffusion by Stability AI | Generate photo-realistic images with text prompt | |
Watson Assistant | Help businesses to build, train, and run conversational interactions across digital channels[22] |
Types of foundation model: established terms
As well as foundation model and GPAI, several other similar terms exist. As noted above, some of these, such as generative AI and large language model, are well-established terms to describe kinds of artificial intelligence.
As noted previously, we have chosen to use ‘foundation model’ as the core term, but recognise terminology is fluid and fast moving.
Generative AI
As suggested by the name, generative AI refers to AI systems that can generate content based on user inputs such as text prompts. The content types (also known as modalities) that can be generated include like images, video, text and audio.
Some forms of generative AI can be unimodal (receiving input and generating outputs based on just one content input type) or multimodal (that is, able to receiving input and generate content in multiple modes, for example, text, images and video).
It is important to note that not all generative AI are foundation models. Generative AI can be narrowly designed for a specific purpose.
Some generative AI applications have been built on top of foundation models, such as OpenAI’s DALL·E or Midjourney, which use natural language text prompts to generate images.
Generative AI capabilities include text manipulation and analysis, and image, video and speech generation. Generative AI applications include chatbots, photo and video filters, and virtual assistants.
Note that generative AI tools are not new, nor are they always built on top of foundation models. For example, generative adversarial networks (GANs) that power many Instagram photo filters and deepfake technologies have been in use since 2014.[23]
Table 2: Examples of generative AI tools designed for a specific use
Application name | Function | Company |
Alexa | A voice assistant that generates audio in response to verbal requests to check a calendar, launch playlists, share information about the weather, or get the latest news.
|
Amazon |
Deep Dream Generator | A computer vision program that finds and enhances patterns in images, creating a dream-like appearance in deliberately overprocessed images.
|
|
StyleGan | Synthesises photorealistic high-quality photos of faces and offers control over the style of a generated image at different levels of detail by varying the style vectors and noise. | Nvidia |
Generative AI techniques
There are a range of generative AI techniques. These include generative adversarial networks (GANs), style transfer, generative pre-trained transformers (GPT) and diffusion models. A short description of each generative AI technique is also included in the Glossary, Table 3.
Large language models
Language models are a type of AI system trained on text data that can generate natural language responses to inputs or prompts.[24] These systems are trained on ‘text prediction tasks’.
This means that they predict the likelihood of a character, word or string, based on the preceding or surrounding context. For example, language models can predict the next most likely word in a sentence given the previous paragraph.[25] This is commonly used in applications such as SMS, Google Docs or Microsoft Word, which make suggestions as you are writing.
Large language models (LLMs) generally refer to language models that have hundreds of millions (and at the cutting edge, hundreds of billions) of parameters, which are pretrained using billions of words of text and use a transformer neural network architecture. A parameter is a connection chosen by the language model and learned during training, sometimes called weights. Transformers are neural networks that learn context and understanding through sequential data analysis.[26]
LLMs are the basis for most of the foundation models we see today (though not all, as some are being trained on vision, robotics, or reasoning and search, for example), performing a wide range of text-based tasks such as question-answering, autocomplete, translation, summarisation, etc. in response to a wide range of inputs and prompts.
Increasingly, these large models are multimodal – that is, can use multiple inputs and generate multiple outputs. For example, while GPT-4 is primarily text-based and gives only text-based outputs, it can use both text and images simultaneously as an input.[27]
Similarly, Google’s PaLM-E, an embodied multimodal language model, is also capable of visual tasks (such as describing images, detecting objects or classifying scenes), and robotics tasks (such as moving a robot through space and manipulating objects with a robotic arm).[28]
Types of foundation model: more contested terms
Frontier models
‘Frontier models’ are a type of AI model within the broader category of foundation models. The term ‘frontier model’ is currently used by industry, policymakers and regulators.
Although there is not a consistent definition, it is increasingly being used to refer to an undefined group of cutting-edge powerful models, for example, those that may have newer or better capabilities than other foundation models. As new models are introduced, they may be labelled as ‘frontier models’. And as technologies develop, today’s frontier models will no longer be described in those terms.
The term ‘frontier model’ is contested, and there is no agreed way of measuring whether a model is ‘frontier’ or not. Currently the computational resources needed to train the model is a proxy that is sometimes used – as it is measurable and provides an approximate correlation with models that might be described as ‘frontier’. However, this may change in the future as compute efficiencies improve and better ways of measuring capability emerge.
Artificial general intelligence (AGI)
Artificial general intelligence (AGI) and ‘strong’ AI are sometimes used interchangeably to refer to AI systems that are capable of any task a human could undertake, and more. Both of these are contested terms. This is partly because they are futuristic terms that describe an aspirational rather than a current AI capability – they don’t yet exist – and partly because they are inconsistently defined by major technology companies and researchers who use this term.
OpenAI and Google DeepMind have both stated ambitions to build AGI, but it is not something that yet exists. There are no current AI models that could be defined as AGI.
However, AGI has entered policy conversations – the European Parliament describes AGI as ‘machines designed to perform a wide range of intelligent tasks, think abstractly and adapt to new situations’.[29] It is therefore important to reference AGI and its place in the overall foundation model discussion.
Researchers at Microsoft have define AGI as ‘systems that demonstrate broad capabilities of intelligence, including reasoning, planning, and the ability to learn from experience, and with these capabilities at or above human-level’.[30]
ISO/IEC (the international standard setting body that manages information security standards) defines AGI as: ‘A type of AI system that addresses a broad range of tasks with a satisfactory level of performance.’ [31] It adds that AGI means ‘systems that not only can perform a wide variety of tasks, but all tasks that a human can perform.’
AGI has also been described by some researchers as ‘high-level machine intelligence’, or when ‘unaided machines can accomplish every task better and more cheaply than human workers’.[32]
AGI’s description therefore contrasts with most AI systems as they exist today, which could be better categorised as ‘artificial narrow intelligence’ (ANI) – also referred to as ‘weak’ AI. These are trained to perform specific tasks and operate within a predefined environment.[33]
Foundation model ecosystems: supply chains, deployers and developers
Supply chains
The AI products we use operate within a complex supply chain, which refers to the people, processes and institutions that are involved in their creation and deployment. For example, AI systems are trained using data that has been collected ‘upstream’ in a supply chain (sometimes by the same developer of the AI system, other times by a third party.
Foundation models require an extremely large corpus of training data, and acquiring that data is a significant undertaking. That data is cleaned and processed, sometimes by the company that develops the model, other times by another company. Once an AI model is put into service, it may be relied on by ‘downstream’ developers, deployers and users, who use the model or build their own applications on it.
For all AI systems, it is important that policymakers understand and regulate not just the original developers of an AI technology, but also the downstream developers and hosting companies within the supply chains of these technologies. [34]
In the case of foundation models, as well as many end applications and purposes, there can be multiple developers and deployers in the supply chain. Because of their general capabilities, there may be a much wider range of downstream developers and users of these models than with other technologies, adding to the complexity of understanding and regulating foundation models.
Hosting and sharing
Foundation models can be made available to downstream users and developers through different types of hosting and sharing. Some models are private and hosted inside a company (like Google DeepMind’s Gato), some are made widely available via ‘open source’ distribution (like HuggingFace’s BLOOM), and some are hosted on cloud computing platforms,[35] like Microsoft Azure or Google Cloud and made accessible via an application programming interface (API).
An API allows developers and users to access and fine-tune – but not fundamentally modify – the underlying foundation model. Two prominent examples of foundation models distributed via API are OpenAI’s GPT-4 and Anthropic’s Claude.
In open-source access, on the other hand, the model (or some elements of it) are released publicly for anyone to download, modify and distribute, under the terms of a licence.
Foundation models with similar capabilities can be shared in different ways. For example, Stability AI and RunwayML released Stable Diffusion – a text-to-image application similar to OpenAI’s DALL·E – via open-source access, in contrast to DALL·E’s API-based release.
Whether released via API or open source, a single issue with a model at the foundation stage could create a cascading effect that causes problems for all subsequent downstream users.
As European civil society groups have noted: ‘A single GPAI system can be used as the foundation for several hundred applied models (for example, chatbots, ad generation, decision assistants, spambots, translation, etc.) and any failure present in the foundation will be present in the downstream uses.’ [36]
Snapshot in time
The topic of AI is fast moving and evolving, and this explainer has been developed as a snapshot in time that can help members of the public, policymakers, industry and media to understand common terms.
While we use ‘foundation models’ as the core term in this explainer, we expect that terminology will quickly evolve. Where possible, we have aimed to provide context relating to the origins and use of terms.
Our aim is to help create a shared understanding, to help ourselves and others select and use meaningful terms that enable effective decision-making. And to better recognise when different interpretations are preventing meaningful conversations.
There are many important questions and debates around this fast-moving field which we will continue to explore and challenge.
Table 3: Glossary
Glossary of terms used within this explainer
Term | Meaning | Origin/context/notes |
Artificial general intelligence (AGI) | Researchers from Microsoft define AGI as ‘systems that demonstrate broad capabilities of intelligence, including reasoning, planning, and the ability to learn from experience, and with these capabilities at or above human-level’.
ISO refer to ‘systems that not only can perform a wide variety of tasks, but all tasks that a human can perform.’ [37] |
Used by DeepMind, OpenAI, Anthropic, Microsoft,
Google.
This term refers to a future form of AI that does not yet exist. Companies like DeepMind refer to AGI as part of its mission – what it hopes to create in the long term. |
Artificial intelligence
|
‘AI can be defined as the use of digital technology to create systems capable of performing tasks commonly thought to require intelligence.
‘AI is constantly evolving, which means its properties and uses change. Two stable characteristics are that it: – involves machines that use statistical methods to find patterns in large amounts of data – enables a machine to perform repetitive tasks with data without the need for constant human guidance’
|
Definition from the UK Government Data Ethics Framework.
We recognise that the terminology in this area is contested. This is a fast-moving topic, and we expect that terminology will evolve quickly.
|
Diffusion models
|
Diffusion models work by adding noise to the available training data and then reversing the
process (known as de-noising or the reverse diffusion process) to recover the original data. The noise is added to help the diffusion model learn to generate new data that is similar to the training data, even when the input is not perfect
|
|
Downstream (in foundation model supply chain) | Refers to activities post-launch of the foundation model and activities that build on a foundation model | |
Embodied multimodal language model | Multimodal language models combine text with other kinds of information, such as images, videos, audio and other sensory data. ‘Embodied’ here means directly incorporating real-world continuous sensor modalities into language models. | |
Fine-tuning | Fine-tuning trains a pretrained model with an additional specialised dataset, removing the need to train from scratch.[38] | |
Foundation model (see also ‘GPAI’)
|
Described by researchers at Stanford University Human-Centered Artificial Intelligence as:
‘AI neural network trained on broad data at scale that can be adapted to a wide range of tasks’ [39] [40] |
Coined by Stanford University Human-Centered Artificial Intelligence.
‘Foundation models are general-purpose technologies that function as platforms for a wave of AI applications, including generative AI: AI systems that can generate compelling text, images, videos, speech, music, and more’. [41] This term is often used interchangeably with GPAI. |
Frontier model | This is used to reference a group of cutting-edge, powerful foundation models. | Used by UK Government including Chair of the UK AI Foundation Model Taskforce, Ian Hogarth.
It is unclear how a model is labelled as at the ‘frontier.’ Benchmarks for general-purpose performance are difficult to define and are frequently debated.[42] |
Generative adversarial network (GAN)
|
GANs are a type of machine learning algorithm that can be used to generate realistic images, text and other forms of data. They work by pitting two neural networks against each other. A generator (trained to generate new examples) and a discriminator (trained to classify examples as real or fake). Once the discriminator is ‘fooled’ about 50% of the time, it shows that the generator model is generating plausible examples.[43] | |
Generative AI
|
A type of AI system that can create a wide variety of data, such as images, videos, audio, text and 3D models.[44] | |
Generative pre-trained transformer (GPT)
|
GPTs are large language models trained on significant datasets of text and code. They can be used to generate text, translate languages and write different kinds of creative content. | |
GPAI (see also ‘foundation model’) | ‘General purpose AI system’ means an AI system that can be used in and adapted to a wide range of applications for which it was not intentionally and specifically designed.’[45] | Under the EU AI Act, the term GPAI refers to an AI system that can be adapted to a wide range of applications.
This term is often used interchangeably with foundation model. |
Large language model (LLM)
|
Large language models are type of AI system trained on significant amounts of text data that can generate natural language responses to a wide range of inputs.
|
Increasingly, these large models are multimodal. For example, while GPT-4 is primarily text-based and only gives text-based outputs, it can use both text and images simultaneously as an input. |
Modality | Type of content. For example, text, images, audio, video, etc. are all ‘modalities’. | |
Multimodal | Multimodal models can take prompts from various modalities (text, image, audio, etc.) and also produce results in multiple modalities (text, image, audio, etc.). [46]
|
|
Narrow AI | AI models that focus on a specific or limited task, for example, predictive text or image recognition. | |
Natural language processing | A branch of artificial intelligence that helps computers understand, interpret and manipulate human language. [47] | |
Neural network | A computing model whose layered structure resembles the networked structure of neurons in the human brain.[48] | |
Noise | Additional artefacts not present in the original content. | |
Parameter | A parameter is a connection chosen by the language model and learned during training. Parameters are sometimes called weights. A parameter count is the number of connections between nodes in a neural network. [49] | |
Pre-trained model | A pretrained AI model is a deep learning model that is already trained (pre-trained) on large datasets to accomplish a specific task.[50] | |
Style transfer | A technique that can be used to apply the style of one image to another image. | |
Text prediction tasks | . A prediction model’s task is to generate the word or words with the highest probability of following the initial prompt, from a sentence, or series of words. [51] | |
Topic model | A type of statistical modelling that uses unsupervised machine learning to identify clusters or groups of similar words within a body of text.[52] | |
Training data | A dataset that is used to teach a machine learning model.[53] | |
Transfer learning | Applying knowledge from one task to another.[54] | |
Transformer model | A transformer model is a neural network architecture that can track relationships in sequential data and form the basis for most current foundation models.[55] | |
Unimodal models | Take prompts from the same ‘modality’ (or type) as the content they generate. | |
Upstream (in foundation model supply chain) | Upstream refers to the component parts and activities in the supply chain that feed into developing the foundation model. |
Footnotes
[1] Ada Lovelace Institute, ‘How do people feel about AI?’ <https://www.adalovelaceinstitute.org/report/public-attitudes-ai/> accessed 17 June 2023
[2] Leverhulme Centre for the Future of Intelligence, ‘THE PROBLEM WITH INTELLIGENCE: ITS VALUE-LADEN HISTORY AND THE FUTURE OF AI’ http://lcfi.ac.uk/resources/problem-intelligence-its-value-laden-history-and-f/ accessed 30 June 2023
[3] Central Digital & Data Office, ‘Data Ethics Framework: glossary and methodology’ (gov.uk) <www.gov.uk/government/publications/data-ethics-framework/data-ethics-framework-glossary-and-methodology> accessed 16 June 2023.
[4] European Commission, ‘COMMUNICATION FROM THE COMMISSION TO THE EUROPEAN PARLIAMENT, THE EUROPEAN COUNCIL, THE COUNCIL, THE EUROPEAN ECONOMIC AND SOCIAL COMMITTEE AND THE COMMITTEE OF THE REGIONS <https://eur-lex.europa.eu/legal-content/EN/TXT/HTML/?uri=CELEX:52018DC0795> accessed 26 June 2023
[5] Küspert, Moës and Dunlop, (2023), ‘The value chain of general-purpose AI’,, <https://www.adalovelaceinstitute.org/blog/value-chain-general-purpose-ai/> accessed: 27 March 2023
[6] Fairness, Accountability, and Transparency (FAccT), ‘Regulating ChatGPT and other Large Generative AI Models’ <https://doi.org/10.48550/arXiv.2302.02337> accessed 30 June 2023.
[7] Stanford Institute for Human-Centered Artificial Intelligence,’Reflections on Foundation Models’ <https://hai.stanford.edu/news/reflections-foundation-models>, accessed 1 July 2023.
[8] OpenAI platform, ‘Models overview’, <https://platform.openai.com/docs/models/overview> accessed 1 July 2023
[9] Strictly speaking, general-purpose transformer models are trained on tokens, not words or images. Tokens can be words, characters, subwords, symbols, images, parts of images or any number of different objects that can ordered in a sequences, depending on the modalities, type and size of the model. Maeda, (2023), LLM AI Tokens, https://learn.microsoft.com/en-us/semantic-kernel/prompt-engineering/tokens (Accessed: 5 June 2023) Ryoo and Arnab, (2021), ‘Improving Vision Transformer Efficiency and Accuracy by Learning to Tokenize’, https://ai.googleblog.com/2021/12/improving-vision-transformer-efficiency.html (Accessed: 5 June 2023);
[10] Risto Uuk, ‘General Purpose AI and the AI Act’ (Future of Life Institute 2022) <https://artificialintelligenceact.eu/wp-content/uploads/2022/05/General-Purpose-AI-and-the-AI-Act.pdf> accessed 26 March 2023.
[11] Mehdi, (2023), ‘Reinventing search with a new AI-powered Microsoft Bing and Edge, your copilot for the web’, https://blogs.microsoft.com/blog/2023/02/07/reinventing-search-with-a-new-ai-powered-microsoft-bing-and-edge-your-copilot-for-the-web/ (Accessed: 26 March 2023);
[12] Duolingo Team, (2023), ‘Introducing Duolingo Max, a learning experience powered by GPT-4’, https://blog.duolingo.com/duolingo-max/ (Accessed: 26 March 2023);
[13] Khan, (2023), Harnessing GPT-4 so that all students benefit. A nonprofit approach for equal access!, https://blog.khanacademy.org/harnessing-ai-so-that-all-students-benefit-a-nonprofit-approach-for-equal-access/ (Accessed: 26 March 2023);
[14] Be My Eyes, (2023), ‘Introducing Our Virtual Volunteer Tool for People who are Blind or Have Low Vision, Powered by OpenAI’s GPT-4’, https://www.bemyeyes.com/blog/introducing-be-my-eyes-virtual-volunteer (Accessed: 26 March 2023);
[15] ADEPT, ‘ACT-1: Transformer for Actions’ <https://www.adept.ai/blog/act-1> accessed 28 June 2023
[16] Jinhyuk Lee and others, ‘BioBERT: A Pre-Trained Biomedical Language Representation Model for Biomedical Text Mining’ (2020) 36 Bioinformatics 1234.
[17] Aditya Ramesh, Mikhail Pavlov, Gabriel Goh, Scott Gray, Chelsea Voss, Alec Radford, Mark Chen, Ilya Sutskever, ‘Zero-Shot Text-to-Image Generation’ (Proceedings of the 38th International Conference on Machine Learning, PMLR 139:8821-8831, 2021.) accessed 26 June 2023
[18] OpenAI, ‘DALL·E 2’ <https://openai.com/dall-e-2> accessed 30 June 2023
[19] Microsoft, ‘Bing Chat’ <https://www.microsoft.com/en-us/edge/features/bing-chat?form=MT00D8> accessed 3 July 2023
[20] Google AI, ‘PaLM 2’ <https://ai.google/discover/palm2/> accessed 29 June 2023
[21] Google, ‘Bard Experiment’ <https://bard.google.com/> accessed 29 June 2023
[22] IBM,’Watson Assistant: Build better virtual agents, powered by AI’ <https://www.ibm.com/products/watson-assistant> accessed 4 July 2023
[23] MIT Technology Review ‘The GANfather: The man who’s given machines the gift of imagination’ <https://www.technologyreview.com/2018/02/21/145289/the-ganfather-the-man-whos-given-machines-the-gift-of-imagination/> accessed 5 July 2023
[24] Analysis and Research Team, ‘ChatGPT in the Public Sector – Overhyped or Overlooked?’ (Council of the European Union General Secretariat 2023) 19 <https://www.consilium.europa.eu/media/63818/art-paper-chatgpt-in-the-public-sector-overhyped-or-overlooked-24-april-2023_ext.pdf> accessed 24 May 2023.
[25] Emily M Bender and others, ‘On the Dangers of Stochastic Parrots: Can Language Models Be Too Big?’, Proceedings of the 2021 ACM Conference on Fairness, Accountability, and Transparency (Association for Computing Machinery 2021) 611 <https://doi.org/10.1145/3442188.3445922> accessed 22 March 2022.
[26] https://www.turing.com/kb/brief-introduction-to-transformers-and-their-power
[27] OpenAI, ‘GPT-4 Technical Report’ (OpenAI 2023) 8 <https://cdn.openai.com/papers/gpt-4.pdf> accessed 16 March 2023.
[28] Danny Driess and others, ‘PaLM-E: An Embodied Multimodal Language Model’ (arXiv, 6 March 2023) <http://arxiv.org/abs/2303.03378> accessed 12 April 2023.
[29] European Parliament ‘General-purpose artificial intelligence’ (2023)<https://www.europarl.europa.eu/RegData/etudes/ATAG/2023/745708/EPRS_ATA(2023)745708_EN.pdf> accessed 3 July 2023
[30] Bubeck, S., Chandrasekaran, V., Eldan, R., Gehrke, J., Horvitz, E., Kamar, E., Lee, P., Lee, Y.T., Li, Y., Lundberg, S. and Nori, H.,’Sparks of Artificial General Intelligence: Early experiments with GPT-4′ (2023) <https://doi.org/10.48550/arXiv.2303.12712> accessed 20 June 2023
[31] International Organization for Standardization and the International Electrotechnical Commission (ISO/IEC) who work on international standards, including for AI
[32] Zhang, B., Dreksler, N., Anderljung, M., Kahn, L., Giattino, C., Dafoe, A. and Horowitz, M.C. ‘Forecasting AI progress: Evidence from a survey of machine learning researchers’ (2022) <https://doi.org/10.48550/arXiv.2206.04132> accessed 25 June 2023
[33] European Parliament ‘General-purpose artificial intelligence’ (2023)<https://www.europarl.europa.eu/RegData/etudes/ATAG/2023/745708/EPRS_ATA(2023)745708_EN.pdf> accessed 3 July 2023
[34] Ada Lovelace Institute, ‘Expert explainer: Allocating accountability in AI supply chains’ (2023) <https://www.adalovelaceinstitute.org/resource/ai-supply-chains/> accessed 1 July 2023
[35] Public cloud platforms are third-party providers (such as Amazon Web Services, Google Cloud, and Microsoft Azure) that offer access to computing resources over the Internet.
[36] Access Now et al., (2022), ‘Call for better protections of people affected at the source of the AI value chain’, https://futureoflife.org/wp-content/uploads/2022/10/Civil-society-letter-GPAIS-October-2022.pdf (Accessed: 21 March 2023);
[37] ISO ‘Information technology — Artificial intelligence — Artificial intelligence concepts and terminology ‘ https://www.iso.org/obp/ui/en/#iso:std:iso-iec:22989:ed-1:v1:en accessed 11 July 2023
[38] AWS, ‘Fine-Tune a Model’ <https://docs.aws.amazon.com/sagemaker/latest/dg/jumpstart-fine-tune.html> accessed 3 July 2023
[39] Stanford Institute for Human-Centered Artificial Intelligence,’Reflections on Foundation Models’ <https://hai.stanford.edu/news/reflections-foundation-models>, accessed 1 July 2023
[40] European Parliament, ‘DRAFT Compromise Amendments on the Draft Report Proposal for a regulation of the European Parliament and of the Council on harmonised rules on Artificial Intelligence (Artificial Intelligence Act) and amending certain Union Legislative Acts’ <https://www.europarl.europa.eu/meetdocs/2014_2019/plmrep/COMMITTEES/CJ40/DV/2023/05-11/ConsolidatedCA_IMCOLIBE_AI_ACT_EN.pdf> accessed 1 July 2023
[41] Department of Commerce, National Telecommunications and Information Administration, ‘AI Accountability Policy Request for Comment’ (2023) <https://hai.stanford.edu/sites/default/files/2023-06/Reponse-to-NTIAs-.pdf> accessed 8 July 2023
[42] Raji, I.D., Bender, E.M., Paullada, A., Denton, E. and Hanna, A. ‘AI and the everything in the whole wide world benchmark’ (arXiv preprint arXiv:2111.15366 2021) <https://doi.org/10.48550/arXiv.2111.15366> accessed 1 July 2023
[43] Machine Learning Mastery ‘A Gentle Introduction to Generative Adversarial Networks (GANs)’ (2019) <https://machinelearningmastery.com/what-are-generative-adversarial-networks-gans/> accessed 30 June 2023
[44] GenerativeAI, ‘All Things Generative AI’ <https://generativeai.net/> accessed 1 July 2023
[45] European Parliament, ‘DRAFT Compromise Amendments on the Draft Report Proposal for a regulation of the European Parliament and of the Council on harmonised rules on Artificial Intelligence (Artificial Intelligence Act) and amending certain Union Legislative Acts’ <https://www.europarl.europa.eu/meetdocs/2014_2019/plmrep/COMMITTEES/CJ40/DV/2023/05-11/ConsolidatedCA_IMCOLIBE_AI_ACT_EN.pdf> accessed 1 July 2023
[46] Walid, H, ‘Unlocking the Potential of ChatGPT: A Comprehensive Exploration of Its Applications, Advantages, Limitations, and Future Directions in Natural Language Processing’ (2023) <https://www.researchgate.net/publication/369771657_Unlocking_the_Potential_of_ChatGPT_A_Comprehensive_Exploration_of_Its_Applications_Advantages_Limitations_and_Future_Directions_in_Natural_Language_Processing> accessed 1 July 2023
[47] SAS, ‘Natural Language Processing (NLP) What it is and why it matters’ <https://www.sas.com/en_gb/insights/analytics/what-is-natural-language-processing-nlp.html> accessed 2 July 2023
[48] Data Bricks, ‘What is a Neural Network?’ <https://www.databricks.com/glossary/neural-network> accessed 1 July 2023
[49] Life Architect, ‘Definitions: A brief list of important definitions in language models (e.g. GPT-3, GPT-J), datasets (e.g. Common Crawl), and AI.’ <https://lifearchitect.ai/definitions/> accessed 30 June 2023
[50] NVIDIA, ‘What Is a Pretrained AI Model?’ (2022), <https://blogs.nvidia.com/blog/2022/12/08/what-is-a-pretrained-ai-model/> accessed 4 July 2023
[51] RStudio, ‘Text Prediction using Natural Language Processing’, <https://rstudio-pubs-static.s3.amazonaws.com/41958_b814065499c04f3dbd3ed287ee57e748.html> accessed 4 July 2023
[52] LevityAI, ‘What Is Topic Modeling? A Beginner’s Guide’ (2022), <https://levity.ai/blog/what-is-topic-modeling#:~:text=Topic%20modeling%20is%20a%20type,predefined%20tags%20or%20training%20data> accessed 3 July 2023
[53] MIT Management, ‘Machine learning, explained’ (2021) <https://mitsloan.mit.edu/ideas-made-to-matter/machine-learning-explained> accessed 6 July 2023
[54] Future of Life Institute, ‘General Purpose AI and the AI Act’ (2022) <https://artificialintelligenceact.eu/wp-content/uploads/2022/05/General-Purpose-AI-and-the-AI-Act.pdf> accessed 3 July 2023
[55] NVIDIA, ‘What Is a Transformer Model?’ (2022) <https://blogs.nvidia.com/blog/2022/03/25/what-is-a-transformer-model/> accessed 3 July 2023
Related content
Foundation models in the public sector
A rapid review of the development and deployment of foundation models in the UK public sector
The value chain of general-purpose AI
A closer look at the implications of API and open-source accessible GPAI for the EU AI Act