Algorithmic decision-making and predictive analytics in children’s social care
A one-day event to discuss the use of data analytics for delivering services within the remits of children’s social care.
On Monday 2 March 2020, the Ada Lovelace Institute, the Nuffield Family Justice Observatory and Nuffield Foundation convened a one-day event to discuss the use of data analytics for delivering services within the remits of children’s social care.
This is a summary of the one-day seminar which brought together scholars and practitioners with expertise in data analytics and social care to help translate and synthesise the arguments around the use of algorithmic decision-making systems in children’s social care and establish a joined-up direction of travel.
On the day, we heard nine presentations on the topic. Highlights from each talk and slides are included below.
Over the last few years, many local authorities have started implementing algorithmic decision-making systems to support the delivery of children’s social care services. Various forms of data analytics are currently deployed, including, but not limited to, predictive analytics. While this is a relatively recent phenomenon, limited to a minority of authorities, it has been growing alongside a robust private market and the slow development of data skills among public servants.
Diving into the use of algorithmic decision-making systems in a key sector of the architecture of national welfare, such as children’s social care—in which consensus over practices is already limited—has surfaced a number of general and specific considerations for research on data-driven public service delivery in any sector. These include:
- Top-level considerations are useful to orientate research, however, context-rich analysis is necessary to achieve a thorough understanding of the reasons behind the implementation of algorithmic decision-making systems and their effects on specific services.
- Research must engage users (service users and front-line workers) and vulnerable groups in a meaningful way, to both understand their perspectives on the systems in use and their changing relations with the services.
- Research should start by analysing the systems in use against the needs they are supposed to address. In children’s social care, the foremost objective is to improve the lives of children and their families.
- The process of implementing data-driven service delivery should be owned by the professionals providing the services and truly support their practice.
- While predictive analytics has been one of the more newsworthy applications of data analytics, it is not the only one. Other applications exist or could be produced, and it is necessary to study their functions and varying effects on people and society.
Speakers
-
Lisa Harker
Director, Nuffield Family Justice Observatory -
Dr David Leslie
Ethics Theme Lead, The Alan Turing Institute -
Dr Lisa Holmes
Director, Rees Centre, University of Oxford -
Michael Sanders
CEO, What Works Centre -
Wajid Shafiq
Chief Executive, Xantura -
Peter Buzzi
PSW Research & Practice Development Project -
Professor Fred Wulczyn
Center for State Child Welfare Data, Chapin Hall, University of Chicago
Algorithmic decision-making in public services in the context of COVID-19
Since early March 2020, the COVID-19 pandemic crisis has disrupted public life in ways that are likely to affect society for many years. The pandemic has required special intervention from the state to front the health emergency and its socio-economic consequences. It has also prompted communities to organise, formally and informally, to offer support to the most vulnerable.
In these exceptional circumstances, we are seeing an amplification of existing inequalities as well as an expansion of the notion of who is vulnerable and needs support.
And so, at this time, algorithmic decision-making has the potential to become instrumental in the delivery of existing, under-resourced public services.
In this rapidly changing scenario, it is possible we will see the implementation of new data-intensive systems, or the ad-hoc re-tooling of existing systems, to help cope with the delivery of services in the short and medium-term.
But it is important to remember that whatever is developed now may be laying the foundations for post-crisis applications. And so, what was, pre-COVID-19, an already-urgent conversation has become even more urgent.
On the day we heard the following nine presentations from 11 expert speakers. Highlights from each talk and their slides are included below:
- Algorithmic decision-making and predictive analytics in children’s social care – Lisa Harker, Director, Family Justice Observatory and Imogen Parker, Head of Policy, Ada Lovelace Institute.
- Decision-making in the age of the algorithm: how frontline practitioners interact with predictive analytics – Thea Snow, Assistant Director, Centre for Public Impact and Author of Decision-Making in the Age of the Algorithm
- Ethics review of machine learning in children’s social care – Dr David Leslie, Ethics Theme Lead, The Alan Turing Institute & Dr Lisa Holmes, Director, Rees Centre, University of Oxford
- We need a more evidence base debate about machine learning – Michael Sanders, CEO, What Works Centre
- Predicting harm and contextual safeguarding – case study from Insight Bristol – Seth Cooke, Business Intelligence Developer, Avon & Somerset Police
- Xantura’s approach to developing predictive tools with councils to prevent adverse child outcomes – Wajid Shafiq, Chief Executive, Xantura
- Reflecting on the ethics of digital practice and machine learning in social care – Peter Buzzi – PSW Research & Practice Development Project
- When policing meets care; a Dutch approach to preventing crime – Fieke Jansen, PhD candidate, Data Justice Lab and Mozilla Public Policy Fellow
- Predictive analytics in care decision making – emerging practice in the US – Professor Fred Wulczyn, Center for State Child Welfare Data, Chapin Hall, University of Chicago
Presentation 1: Algorithmic decision-making and predictive analytics in children’s social care
Presentation by Lisa Harker, Director, Family Justice Observatory and Imogen Parker, Head of Policy, Ada Lovelace Institute.
If we look at the current status of children’s social care in numbers, most of the work of delivering social care is, indeed, already largely about calculating risk and prioritising interventions. The huge variation in social care intervention rates and care proceedings across the country shows that there is no national consensus on when the State should intervene in family life. There is a wide range of decisions made, not just about whether to place a child into care. The system, as it stands, with its geographical disparities, is clearly unequal and human decision-making is not consistent. However, it is so far unclear whether predictive analytic models could ameliorate the situation.
There are at least five contexts, each encompassing the previous one, that we should consider when studying the implementation of predictive analytics in the delivery of any public service:
- how the tools function in terms of the data they use, their accuracy, the bias they embed, how explainable they may be
- how the use of sophisticated data analytics influences professional decision-making and the day-to-day practice of social workers
- how a public service as a whole is affected – whether the implemented tools do make it more effective in terms of producing better outcomes for people, whether the use of data analytics changes how users interact with services, whether the tools in use are sustainable etc.
- how the widespread implementation of data analytics may have already started affecting the relationship between the individual and the State and what we expect from it
- how society as a whole is impacted by the use of predictive analytics in public services – what are the cumulative and distributive effects? How is our experience of government and rights to welfare changing?
Presentation 2: Decision-Making in the Age of the Algorithm: how frontline practitioners interact with predictive analytics
Presentation by Thea Snow, Assistant Director, Centre for Public Impact and Author of Decision-Making in the Age of the Algorithm
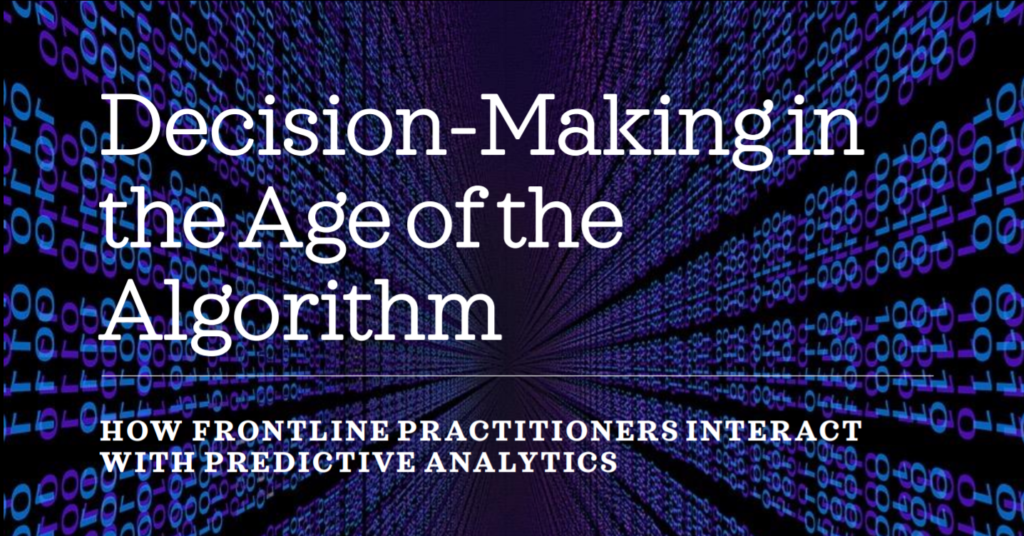
The research ‘Decision-Making in the Age of the Algorithm’ looked at the reaction of children’s social care practitioners to the use of data-driven technologies in the work context. The question driving the project was: how are practitioners in the field of children’s social services using algorithmic tools to support their decision-making?
The study started with the hypothesis that practitioners may react according to:
- algorithm aversion (ignoring the tool)
- automation bias (letting the analytic tool override their own judgement)
- biased artificing (arriving at biased resolutions when working with the tool)
- expert artificing (arriving at resolutions informed by their expertise, but using the tool).
The results showed the need for further research on:
- how the introduction of data-driven technologies changes the profession of social work
- what kind of support social workers need to navigate these changes.
It is key that social workers maintain their professional agency when the use of computational tools are integrated into their workflow. Tools ought to be introduced in a manner that is cognizant of the context in which they will be deployed and front-line staff must be able to understand how the tools operate. Adequate training should be provided to this end.
Often-times, the social workers with better digital skills have an easier grasp of the data analytics technology they are asked to utilise. Better grasp means more trust in the system as well as more confidence in overruling the recommendations made by the analytic model.
Presentation 3: Ethics review of machine learning in children’s social care
Presentation by Dr David Leslie, Ethics Theme Lead, The Alan Turing Institute & Dr Lisa Holmes, Director, Rees Centre, University of Oxford
Together, The Turing Institute and Rees Centre conducted research on the ethics of machine learning in the context of children’s social care. The project studied whether it is ethical to use machine learning approaches in children’s social care and, if so, how and under what circumstance?
Among other aspects, the study highlighted:
- in-practice ethical issues – such as loss of agency for service users and social workers, poor quality outcomes and further embedding of bias and discrimination in the social care system
- technical and structural questions – concerning the level of institutional preparedness and rationale for deploying data-driven technologies, whether high-quality data is ever enough to describe a family’s situation, etc.
Through engaging with families who interact with children’s social services, the research highlighted concerns around the widespread lack of transparency over the data-driven systems in use and who is implementing them. There is a need to:
- improve data quality
- standardize the processing mechanisms
- conduct more empirical research to establish the impact of data-driven technologies on children’s social services and what skills are necessary to make the best of it.
The responsible design and use of technologies, such as machine learning in children’s social care, should be mandated at the national level and be truly inclusive and consent-based. The engagement with the families should be thorough and enable them to express meaningful consent.
Notably, the families engaged did not show an immediate and un-reflective rejection to the use of predictive analytics. However, they stressed that the systems, as implemented, are un-transparent, and therefore unaccountable, and their efficacy is not proved.
Presentation 4: We need a more evidence-based debate about machine learning
Presentation by Michael Sanders, CEO, What Works Centre
The remit of the What Works Centre for Children’s Social Care is to produce an empirical evidence base for policymaking and its team has been working together with five UK local authorities, using datasets already available, to develop analytic models that can predict the occurrence of events. The models are not currently implemented nor will be in the future, rather the exercise of programming and testing them aims to benchmark the application of predictive analytics to children’s social care.
Once the analytic models are ready, their source-code, performance and programming development process will be made public in order to display best practice in terms of transparency.
The Centre hopes to engage local authorities and service users with their empirical findings and discuss questions of consent and accountability of other systems in use or of the ones that may soon be implemented.
Presentation 5: Predicting harm and contextual safeguarding – case study from Insight Bristol
Presentation by Seth Cooke, Business Intelligence Developer, Avon & Somerset Police
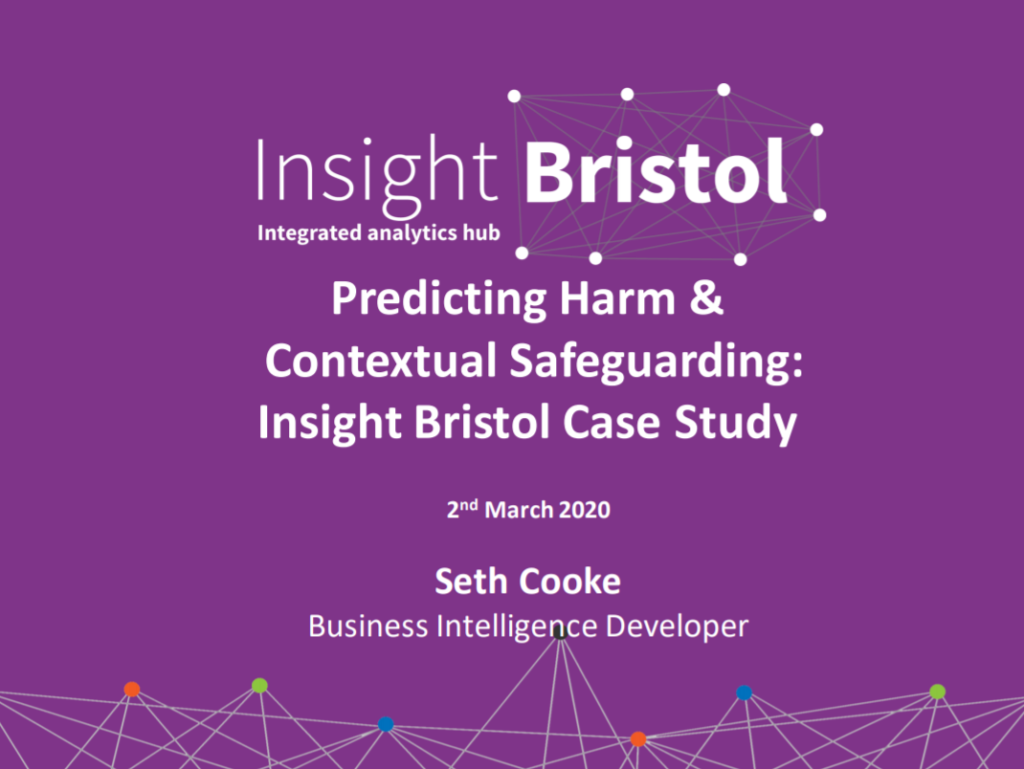
The Think Family Database aggregates data-sets from a variety of sources and is programmed and managed by Insight Bristol, a multiagency hub made up of council workers and police forces. One function of Think Family is social network analysis for contextual safeguarding. It is not a predictive technique but used to prevent harm to children.
Contextual safeguarding is based on an analysis of children’s networks of peers and not only of kin and extends harm prevention outside the family circle. Studying someone’s network may contribute to identifying emerging risks, cohorts and their habits and establish patterns. Notably, in order to carry out any effective intervention on the basis of the insights offered through social network analysis, a significant amount of expert staff is needed, not only at the level of data analytics but also front-line service level. While the technique differs from predictive analytics, it foregrounds comparable ethical issues and requires robust governance and public awareness.
Presentation 6: Xantura’s approach to developing predictive tools with councils to prevent adverse child outcomes
Presentation by Wajid Shafiq, Chief Executive, Xantura
Xantura and its CEO, Wajid Shafiq, have had an instrumental function in highlighting the necessity of data linkage and standardisation, and lack thereof, in government functions.
Their One View platform, recently adopted in multiple UK local councils, links datasets from different sources and amplifies the capacity to establish correlations between phenomena and potential situations of harm.
The data analytic model may offer insights without generating alerts, but a variety of issues do emerge when alerts are generated. These should be the subject of further research. Data analytics offers the opportunity to anticipate harm, but, considering that interventions can be operationalised only when harm is somehow already happening, the logic of anticipation is curtailed in the process. Further to this, an analytic model, if accurately observed may show how bias is embedded in datasets, therefore raising ethical concerns as to how one can act upon certain alerts.
Presentation 7: Reflecting on the ethics of digital practice and machine learning in social care
Presentation by Peter Buzzi – PSW Research & Practice Development Project
From the perspective of social workers and care practitioners, the lack of standard practices and digital preparedness are among the main concerns.
The implementation of artificial intelligence in children’s social care is often perceived as the direction of travel and the lack of adequate training and debate in this context hinders its application and adds to the difficulty of working in this context in a regime of austerity. The sector has seen £16bn cuts in government core funding and the risk is not being able to deliver on the principles of the Children’s Act, which states that each child should have equal opportunities to flourish.
Presentation 8: When policing meets care – a Dutch approach to preventing crime
Presentation by Fieke Jansen, PhD candidate, Data Justice Lab and Mozilla Public Policy Fellow
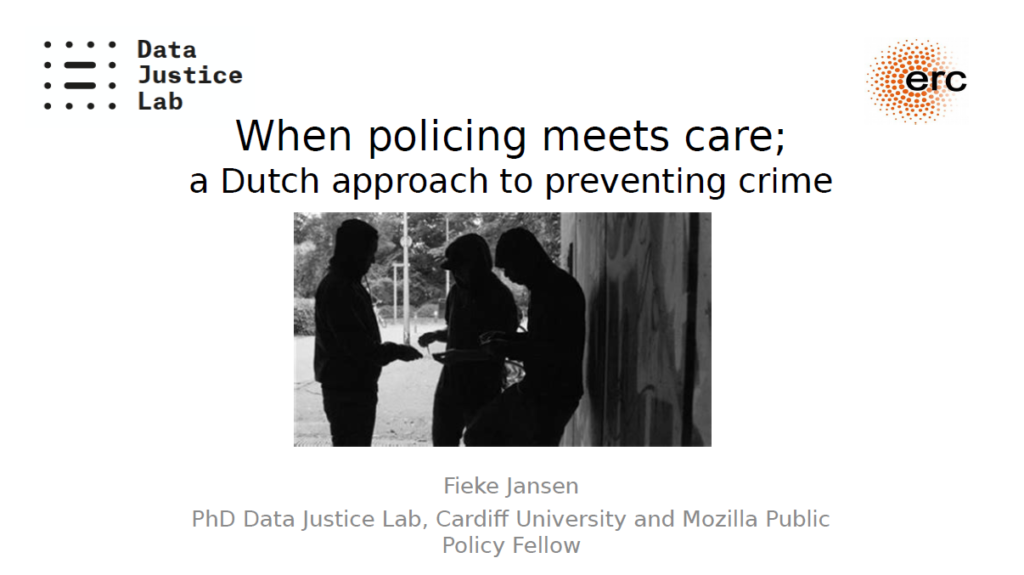
The Top 600 model, in use in the city of Amsterdam in The Netherlands, is the longest-running data-driven scoring system in the country and compiles a list of the 600 children older than 12, who are at risk of being involved in serious crimes. The programme assigns to each child a mentor, who helps them navigate the system of social care and works towards the goals of improving their life conditions, preventing their engagement in criminal activities and minimising the negative effects that a young criminal may have on their kin and vice-versa.
Among other aspects of the implementation of the Top 600, we find that data sets are used to identify who may be at risk of being involved in crime but are not used to capture whether the programme is having an impact. The programme is implemented in a relatively top-down fashion and has little consideration for individuals’ analysis of their conditions. It also shows a lack of awareness of the fact that the choice of supporting children who are at risk of committing certain crimes as opposed to others means that the demographic mostly captured in the Top600 list is one characterised by living in poverty and at the margins of Dutch society. This is one of the reasons why the list is perceived as a stigmatizing tool.
Presentation 9: Predictive analytics in care decision making – emerging practice in the US
Presentation by Professor Fred Wulczyn, Center for State Child Welfare Data, Chapin Hall, University of Chicago
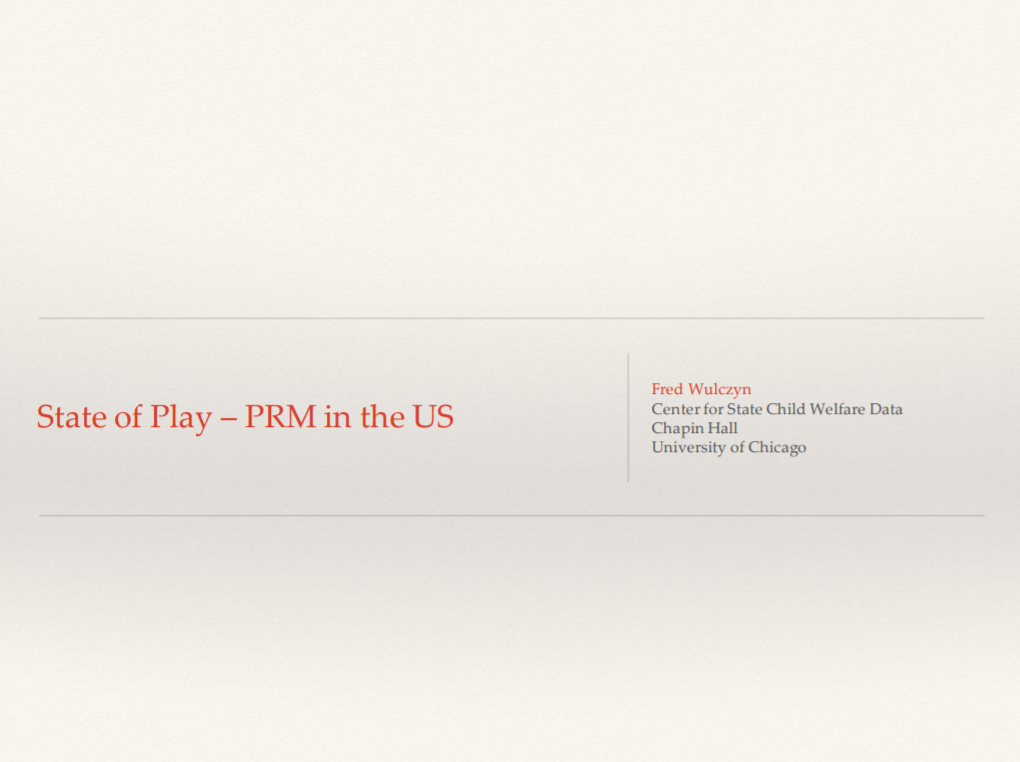
Wulczyn’s presentation touched upon the use of predictive analytics in Allegheny County, Pennsylvania, Tennessee, and New York City and the approach of latent critique that his practice takes vis-à-vis data analytics.
Among the key features of predictive analytics in Allegheny County, there is a general tendency towards caution, but also hope that by screening more families, resources will be allocated more effectively. In line with this principle, the County is gearing up to implement the Hello Baby Programme, which will screen all children at the moment of birth.
The implementation of predictive analytics in Tennessee starts from an observation of disrupted placements and the need to identify ways to make social workers into better decision makers. Among the questions driving this implementation are: how do we develop social workers’ capacity to operationalise large sets of administrative data?
Similarly, in New York City, social workers’ capacity to prioritise the collection of certain information is seen as a priority. In order to be best placed to intervene, social workers blend their practical knowledge with the capacity to understand what additional piece of information may make a difference in their assessment of a situation.
It is necessary to run a latent critique in parallel to the work of producing data analytic models. We ought to remember that the goal of predictive analytics is improving outcomes for people that find themselves in difficult situations rather than producing better algorithms. In carrying out this task a variety of principles should direct our practices, including the fact that each decision is to be taken in context and that the systems on the basis of which a predictive model is built are not currently working for everybody. With regards to this, we may find it useful to move away from talking about data, as data can work only when placed within narratives, and consider in the context of the full-blooded reality from which it is extracted.
Related content
Examining the Black Box
Identifying common language for algorithm audits and impact assessments